Information Technology Reference
In-Depth Information
is employed to reduce the number of input variables of ELM-QNN. Owing to
the introduction of PCA, the number of input variables is reduced to 8.
We establish three ELM-QNNs with the same network structure to assess the
CO concentration, H
2
concentration, and CO
2
concentration in the Texaco syn-
gas, respectively. The number of the hidden nodes is set to 80. The training data
are exposed to the PCA-ELM-QNNs. As shown in Fig. 2(a), Fig. 3(a) and Fig.
4(a), the training results of the three PCA-ELM-QNNs fit the analyzed values of
CO, H
2
,andCO
2
concentrations well. By using ELM method, the parameters
of QNN are identified. Then, in Fig. 2(b), Fig. 3(b) and Fig. 4(b), the testing
results indicate that PCA-ELM-QNNs provide the accurate measurements of
the three components. The proposed models have good prediction capability.
Fig. 2.
Assessment of CO concentration in Texaco syngas (a) for training data and (b)
for testing data
In a separate study, three other modeling methods, including SLFN (i.e. 3-
layer BPNN), ELM-SLFN, and ELM-QNN, are adopted for comparison purpose.
The network structures of the compared methods are the same as that of PCA-
ELM-QNN. The comparison results of the four methods are shown in Table 1.
For SLFN, the training results might over-fit to the training data. This would
cause the bad generalization performance. For the PCA-ELM-QNN, the redun-
dant information in the original sample data is eliminated by using PCA, and
then ELM approach is used to identify the parameters of the proposed QNN.
Generally, the generalization capability is the primary concern. In comparison
with the three other methods on the testing sample, the mean square error (MSE)
when using PCA-ELM-QNN is smaller for CO and H
2
. Although the MSE for
CO
2
is a little larger than that when using ELM-QNN, the concentration of
CO and H
2
are the more significant components than CO
2
concentration. Thus,
the performance degradation of the PCA-ELM-QNN for CO
2
is acceptable. The
above results indicate that the proposed PCA-ELM-QNN performs better than
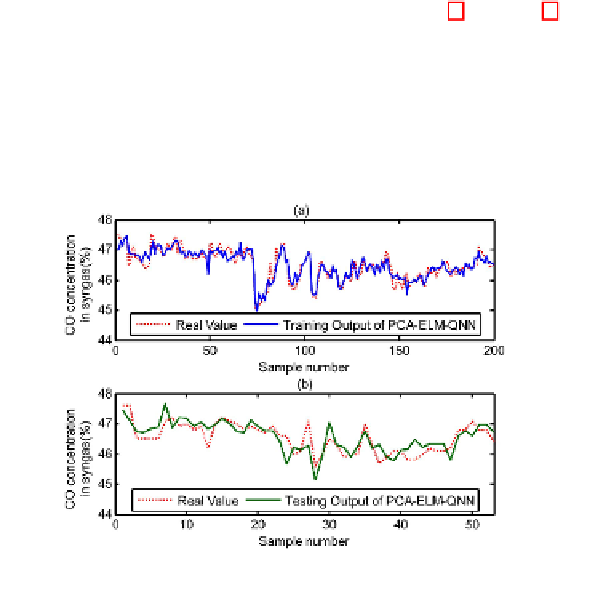


Search WWH ::

Custom Search