Digital Signal Processing Reference
In-Depth Information
10
0
V-Blast (ideal CS)
NN V-Blast
LMS-NN Equalizer
10
-1
10
-2
10
-3
10
-4
10
-5
5
10
15
20
25
30
SNR
FIgure 5.17
BER performance versus SNR.
Here the transmitted signals are 16-QAM modulated, and
M
=
L
= 2. he unknown
propagation channel is modeled as time-varying Rician fading gains with a Rician fac-
tor
K
= 5 and normalized Doppler frequency of
f
D
= 10
-4
. For the modeling and iden-
tification part, the noise is taken as white Gaussian with variance 0.001. However, for
the detection part, the noise is varied depending on the channel signal-to-noise ratio
(SNR). Each subnetwork in each block is composed of
N
= 5 neurons. It is assumed in the
simulations that the HPA transmission powers are known to the receiver. The receiver
is trained using a training sequence of fifty thousand transmitted symbols, after which
the DD mode is activated.
Figure 5.17 shows the BER performance of our NN V-BLAST receiver. The proposed
receiver achieves a bit error rate (BER) of 10
-4
at an SNR of 29.2 dB. The NN V-BLAST
receiver is compared to a least mean squares (LMS) tracker following a memoryless neural
network (LMS-NN) equalizer [6]. It is composed of a linear combiner,
W
-1
, acting as an
inverter to the channel matrix
H
, and a NN structure, acting as an inverter to the channel
nonlinearities. The general structure of the LMS-NN equalizer is shown in
Figure 5.18
. BER
simulation results show that our NN V-BLAST receiver outperforms the LMS-NN equal-
izer in terms of BER. We can also see that the performance of the NN V-BLAST receiver is
close to that of the ideal V-BLAST receiver, which assumes perfect channel knowledge. This
should not be surprising, since the different parts of the channel have been well identified.
5.4
Conclusion
The chapter proposed an NN approach for modeling nonlinear MIMO channels com-
posed of a set of memoryless nonlinearities followed by a linear combining channel. We
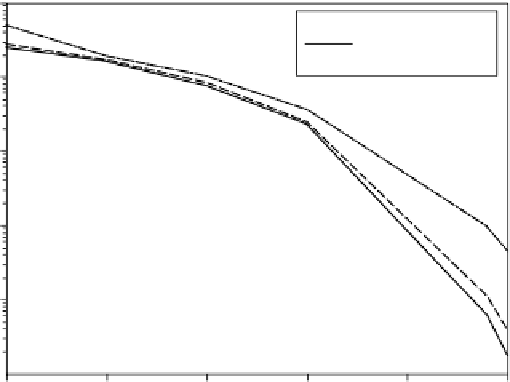
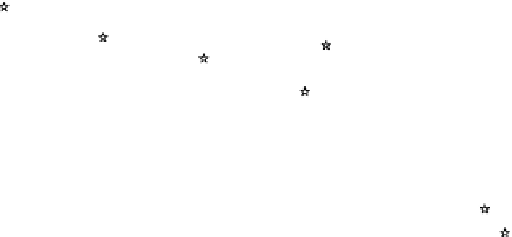







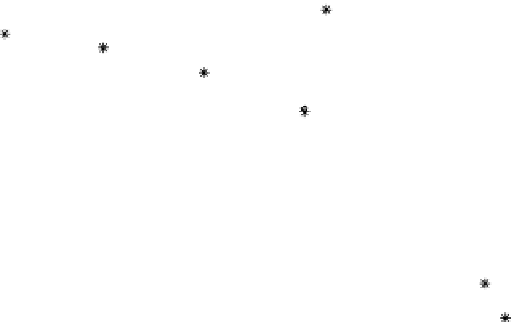


Search WWH ::

Custom Search