Digital Signal Processing Reference
In-Depth Information
N
1
(
n
)
h
11
x
1
(
n
)
g
1
(.)
y
1
(
n
)
∑
h
1
M
N
2
(
n
)
x
2
(
n
)
g
2
(.)
y
2
(
n
)
∑
h
L
1
N
L
(
n
)
g
M
(.)
y
L
(
n
)
x
M
(
n
)
∑
h
LM
FIgure 5.1
Nonlinear MIMO system.
system to low-order modulation schemes. In addition to the nonlinear behavior of the
HPAs, a further challenge lies in the variations of the wireless fading channel [5, 17, 18].
Therefore, to reach maximum throughput, knowledge of accurate and timely
channel state information (CSI) is needed by the receiver for accurate detection and
demodulation.
Modeling the channel time-varying parameters and the HPA nonlinearity [5, 12] is
a highly challenging task, especially when both the nonlinearity and fading parameters
are unknown.
The nonlinear MIMO channel studied in this chapter is depicted in Figure 5.1. It is
composed of
M
inputs,
M
memoryless nonlinearities (representing the HPAs), a linear
combiner
H
(representing the propagation channel), and
L
outputs (representing the
receiving antennas).
The chapter proposes a block-oriented neural network (NN) approach [4, 6] to adap-
tively identify the overall MIMO input-output transfer function and characterize each
component of the system (i.e., the memoryless nonlinearities and the linear combiner).
The proposed NN model is composed of a set of memoryless NN blocks followed by an
adaptive linear combiner. Each block in the adaptive system aims at identifying the cor-
responding block in the unknown MIMO system.
The chapter is organized as follows. Section 5.2 presents the system to be identified
and the NN algorithm. Section 5.3 presents some applications and simulation results.
5.2
System Model and Neural Network Algorithm
5.2.1 System Model
The nonlinear MIMO channel is presented in Figure 5.1. The system is assumed to
be composed of
M
zero-mean uncorrelated inputs
x
i
(
n
),
i
= 1, …,
M
. Each input is
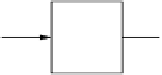


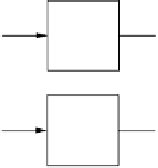




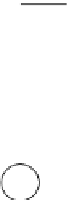


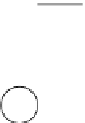


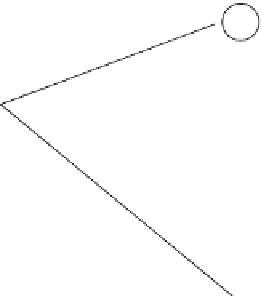


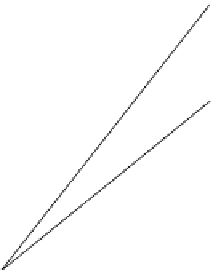


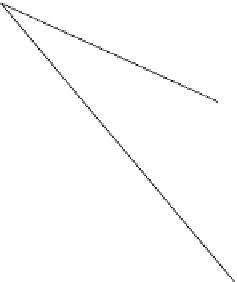











Search WWH ::

Custom Search