Database Reference
In-Depth Information
Figure 2.1
Key roles for a successful analytics project
Although most of these roles are not new, the last two roles—data engineer and
data scientist—have become popular and in high demand [2] as interest in Big Data
has grown.
2.1.2 Background and Overview of Data Analytics Lifecycle
The Data Analytics Lifecycle defines analytics process best practices spanning
discovery to project completion. The lifecycle draws from established methods in
the realm of data analytics and decision science. This synthesis was developed
after gathering input from data scientists and consulting established approaches
that provided input on pieces of the process. Several of the processes that were
consulted include these:
•
Scientific method
[3], in use for centuries, still provides a solid
framework for thinking about and deconstructing problems into their
principal parts. One of the most valuable ideas of the scientific method
relates to forming hypotheses and finding ways to test ideas.
•
CRISP-DM
[4] provides useful input on ways to frame analytics
problems and is a popular approach for data mining.
• Tom Davenport's
DELTA
framework [5]: The DELTA framework offers
an approach for data analytics projects, including the context of the
organization's skills, datasets, and leadership engagement.
• Doug Hubbard's
Applied Information Economics (AIE)
approach
[6]: AIE provides a framework for measuring intangibles and provides
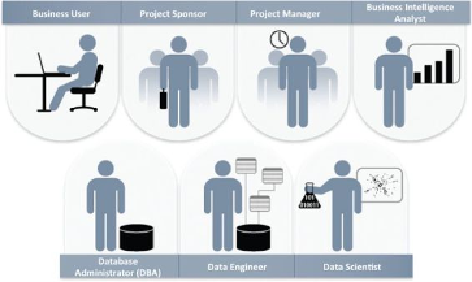