Agriculture Reference
In-Depth Information
1
2
3
4
5
6
7
8
9
10
11
12
13
14
15
16
17
18
19
20
21
22
23
24
25
26
27
28
29
30
31
32
33
34
35
36
37
38
39
40
41
42
43
44
45
46
data-based variables that can be used to predict crop yield are described in
chapters 5, 6, 9, 13, 19, and 28.
The short-term estimates are available just before or around harvest
time. But many times long-term estimates are required to predict drought
for next year, so that long-term planning for dealing with the effects of
drought can be initiated in time. Long-term predictions are especially useful
for food-grain exporting countries, such the United States and Canada,
because grain-exporting agencies are interested in knowing the amount of
food grains available for export next year to help set the export targets.
For such prediction, time series analysis can be performed.
Ti
me Series Analysis
Weather data during a growing season cannot be used for obtaining the
long-term estimates simply because the long-term estimates are required
before crops are even sown. As the yield is known to be influenced most by
weather conditions during the growing season, it is a common practice to
estimate yield using weather data. Attempts to obtain long-term estimates
that do not employ weather data are limited.
As an alternative to weather data, annual time series of yield data is
used to obtain the long-term yield estimates by modeling the series. In a
time series analysis, a variable to be forecasted (yield, in the present case)
is modeled as a function of time:
[41],
Line
——
-0.1
——
Norm
PgEn
Y
t
=
f
(
t
)
+
ε
t
[4.1]
[41],
where
Y
t
is yield for year
t
,
f
(
t
) is a function of time
t,
and
ε
t
refers to error
(i.e., the difference between observed yield and forecasted yield for year
t
). Once a functional relationship between yield and time is developed,
yield for the year ahead can be forecasted. A few techniques (e.g., linear
trend, quadratic trend, simple exponential smoothing, double exponential
smoothing, simple moving averaging, and double moving averaging) can
be used to model an yield series (Boken, 2000).
Pa
ttern Recognition
Pattern recognition (PR) is a process to classify an object by analyzing the
numerical data that characterize the object. Various academic fields, such
as image processing, medical engineering, criminology, speech recognition,
and signature identification, have applied PR to classify objects of interest
(Duda et al., 2001). However, PR techniques have not been exploited for
drought prediction. The process of PR begins with the identification of a
variable that can be used to define the object under study. In the case of
agricultural drought, the object can be yield of a major crop in the region.
Various pattern recognition techniques are available in the literature
(Jain and Flynn, 1993; Duda et al., 2001), but only a few techniques are
relevant to the case of agricultural drought. First, potential variables that
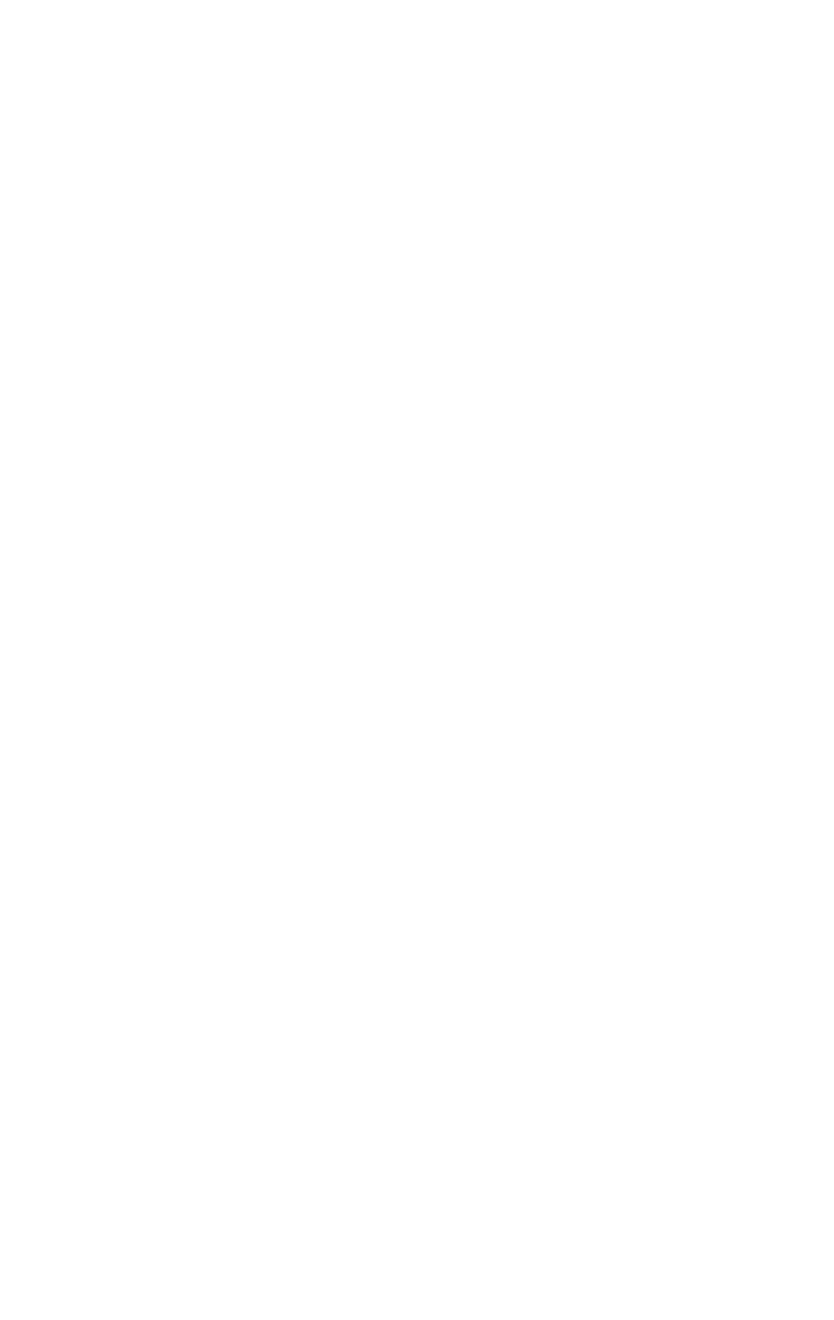










































Search WWH ::

Custom Search