Biomedical Engineering Reference
In-Depth Information
Continuous cell culture
Day 3
0
Day 1
Day 14
(3) Experiment data collection
(Cell growth rate = Target value)
(1) Image data
collection
Cell yield prediction
from early cell images
Length
Breadth
Size
Length
Breadth
Size
(2) Image data
processing
Unknown image set
О
О
О
(4) Multiple regression modeling
Total 120 parameters
(Morphological features = Inputs)
Fig. 3
Schematic illustration of the cell yield prediction model and its construction
original filter sets. Briefly, the raw images were pre-processed using open-close
filters and binarized using the optimized threshold, and all objects in the image
were measured with the integrated morphometry analysis process. Image data were
pre-processed using the universal threshold optimized with 20 randomly picked
image samples. Using integrated morphometry analysis according to the manu-
factuer's manual (
http://mdc.custhelp.com/app/answers/list/c/110
)
, the number of
objects was measured together with 22 individual morphological features in
MetaMorph, such as total area, standard area count, perimeter, width, height,
orientation, length, breadth, fiber length, fiber breadth, shape factor, elliptical form
factor, inner radius, outer radius, mean radius, equivalent radius, equivalent sphere
surface area, equivalent sphere volume, equivalent prolate volume, equivalent
oblate volume, hole area, and relative hole area. Prior to statistical analysis,
morphological data from noise objects (non-cellular objects) were automatically
removed using the original noise-reduction algorithm (image auto-wash method).
Statistics were calculated with each measured feature in five view fields from the
same well. For each feature, (a) average of day 1, (b) standard deviation of day 1,
(c) average of day 3, (d) standard deviation of day 3, (e) average ratio of the day 1,
and day 3 averages, (f) average ratio of the day 1 and day 3 standard deviations,
were calculated as input features. From a total of 184 input features, 120 features
with a CV (coefficient of variation) of \20 were used as inputs in the modeling.
Input features were selected by stepwise parameter selection in the multiple
regression model analysis using SPSS software
(for Windows
11.5.1, IBM,
Armonk, NY, USA).

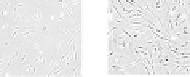
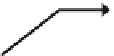




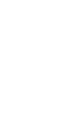



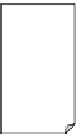







































































Search WWH ::

Custom Search