Agriculture Reference
In-Depth Information
suggested that the genotype being regressed should be
omitted from calculating the site mean, although this
has been shown to make little difference to the slopes
obtained. Another way to avoid this is to carry out
regression of the breeding lines onto the performance of
one (or more) control cultivar (say one which is known
to have stable performance over locations and years).
Other methods are available to examine G
a
c
b
×
E
interactions in breeders' trials. Genotypic stability over
environments can be examined by comparing the
variance of each genotype over the different environ-
ments. This method can produce very similar results to
joint regression analysis with increasing variation over
environments related to less stability.
Genotype means and variances over environments
can also be used simultaneously to predict the frequency
that test entries would exceed specified target values
(often the value of controls in the trials). One advantage
of the probability prediction method is that it provides a
single datum for each test entry to be evaluated (i.e. the
probability that a genotype will produce a yield greater
than 2000 kg/ha). A second, perhaps more important
feature, is that this method can be expanded to cover sev-
eral traits simultaneously. This is achieved by estimating
the average performance for each trait, the variation over
environments for each trait and the covariance between
traits and environments. Probabilities are calculated by
evaluating the area under a univariate or multi-variate
normal frequency distribution (see cross prediction
later).
Finally, another form of analysis called
Additive
Main Effects and Multiplicative Interaction
(AMMI)
has been suggested as suitable by several researchers.
An AMMI analysis partitions the residual interaction
effects between genotypes and environments by prin-
cipal component analysis. The technique therefore
involves a multi-variate transformation of the residuals
(after the main effects of genotypes and environments
have been removed) within a two-dimensional table
of genotypes and environments. Provided the first
and second eigen vectors (transformations) account
for a large proportion of the residual data, two-
dimensional graphic inspection of eigen values from the
first and second eigen vectors, for different locations and
genotypes can be used to examine the interaction effect.
The theory of AMMI is outside the scope of this topic
although readers should be aware that this option of
interpreting data is available.
d
Environment means
Figure 7.11
Different phenotypic response (yield) to
environment as measured by average performance and
regression coefficient of performance against average
environment performance where (a) has high average yield
and high 1
+
β
response, (b) low average yield and high
1
+
β
+
β
, (c) high average yield and low 1
, and (d) low
+
β
average yield and low 1
.
+
β
than those with low 1
values. From the analysis
there can be four types of genotype:
+
β
•
High average performance and high 1
value
Low average performance and high 1
+
β
value
•
High average performance and low 1
+
β
value
•
Low average performance and low 1
+
β
value
•
The four types of genotype are shown diagram-
matically in Figure 7.11. Obviously all plant breeders
would like to develop cultivars which have high average
performance and low 1
values as these geno-
types would produce high yield in all environments.
Unfortunately performance and regression coefficient
are usually related with high expression associated with
high sensitivity.
Major criticism of joint regression analysis is that the
regression is carried out between the performance of
each genotype at each site onto the average performance
of all genotypes at that site. Obviously these two are
not independent as the genotype performance is
a fac-
tor in determining the site mean
. To avoid this it has been
+
β
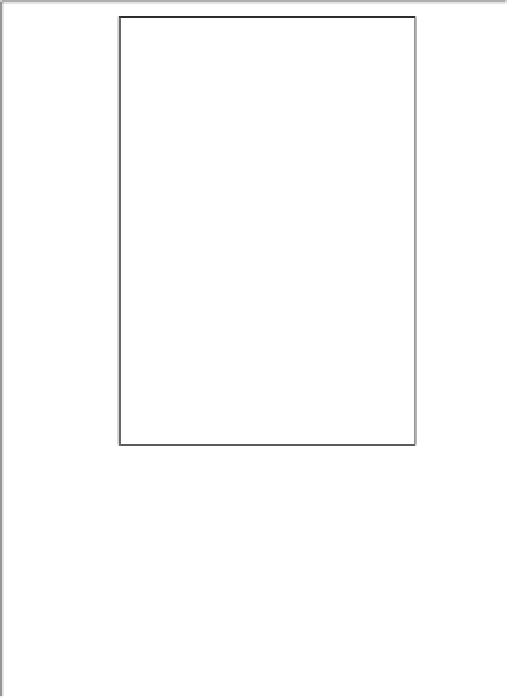
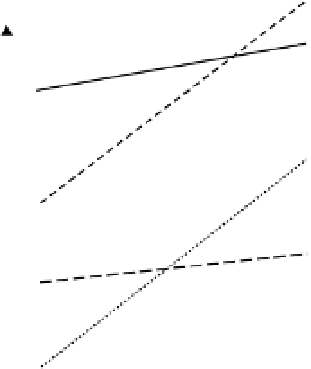








