Agriculture Reference
In-Depth Information
variance to their expectations in the random model.
Therefore, in terms of a mathematical model the yield
y
ijk
of the
k
th replicate of the
i
th genotype, grown in
the
j
th environment can be estimated by the formula:
y
ijk
=
µ
+
(i.e.
y
years and
s
sites would result in
ys
environments).
Environmental differences are often greater over years
than over locations and it can be informative to separate
year and location effects in the analysis of variance.
More complex models and expected mean squares
can be derived for location trials grown over more than
one year which produce year
g
i
+
e
j
+
ge
ij
+
E
ijk
(7.1)
µ
where
is the average yield over all trials,
g
i
is the effect
of the
i
th genotype,
e
j
is the effect of the
j
th environ-
ment,
ge
ij
is the interaction between the
i
th genotype
and the
j
th environment and
E
ijk
is an error term. Also:
i
g
i
=
j
e
j
=
ij
ge
ij
=
×
×
genotype, location
×
×
genotype and year
year interactions. In
this case the expected mean squares (EMS) would be
those shown in Table 7.7.
Given that a significant interaction is detected in the
analysis of variance, and this is not due to a scalar
effect, then it can often be useful to have additional
information regarding the partition of the interaction
variance.
location
0
The expected mean squares from an analysis of vari-
ance are dependent on whether main effects are fixed
or random. In the case of genotype
×
environment tri-
als it is usually assumed that both effects are random.
From this, given a set of trials with
g
genotype entries,
r
replicates at each location and
l
locations the follow-
ing expected mean squares would be obtained from an
analysis of variance, shown in Table 7.6.
In this case the genotype
Worked example
Consider the example where 20 spring canola (
B. napus
)
cultivars or breeding lines were tested for yield potential
in nine different environments throughout the Pacific
Northwest region of the United States. The analysis of
variance was found to be that shown in Table 7.8.
From this we have detected highly significant dif-
ferences between sites (locations) and cultivars; and
a
location interaction
would be tested for significance against the pooled repli-
cate
w
locations
×
genotypes interaction. The effect of
genotypes would be tested in an F-test against the inter-
action G
×
E. Replicates
w
locations would be tested
against the replicate error and the effect of locations
would be tested against the replicates
w
locations mean
square.
When both years and locations are included, often
different locations (or different numbers of locations)
are used in each year. In these cases the analysis reduces
to genotypes
×
highly
significant
interaction
between
sites
and
cultivars.
However, when yield data from each location were
analyzed separately we find that the error mean squares
from each analysis of variance differ. This might have
been expected given the large difference between the
average yield at each location. The question is whether
these differences are significant.
A significant
×
environments interactions where envi-
ronments include a combination of years and sites
2
0.001) is found from
a
Bartlett analysis
indicating that these error variances
are significantly different. In this case, therefore, we are
violating one of the least robust assumptions on which
the analysis of variance is based.
This is not uncommon and the question would then
arise '
well, what can I do about a situation where there
is heterogeneity of variances?
' The only practical solution
to overcome this is to transform the data. The most
common transformations are square root, log, and to
transform the data to standardized normal distributions
with mean zero and variance of one.
Given that a significant interaction is detected in the
analysis of variance, and this is not due to a scalar
effect, then it can often be useful to have additional
χ
value (
p
<
Table 7.6
Degrees of freedom and expected mean squares
from an analysis of variance with
g
genotype entries, grown at
l
locations, and
r
replicates at each location. In this analysis
it is assumed that locations are random effects.
Source
d.f.
EMS
2
2
l
e
+
−
σ
σ
r
w
l
+
σ
Locations (
l
)
l
1
g
rg
Replicates
w
locations (
r
)
2
r
w
l
e
+
−
σ
σ
l
(
r
1)
g
e
+
2
g
Genotypes (
g
)
g
−
1
σ
r
σ
gl
+
rl
σ
×
Genotypes
e
+
2
gl
−
−
σ
σ
locations
(
g
1)(
l
1)
r
e
−
−
σ
Error
l
(
r
1)(
g
1)
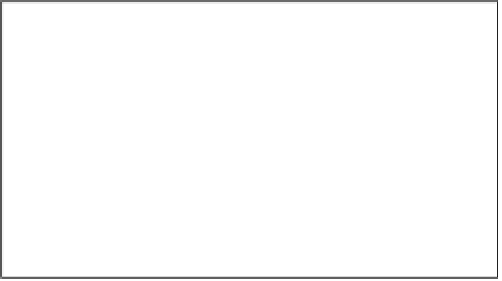







