Databases Reference
In-Depth Information
1
0.8
0.6
0.4
0.2
0
20
30
40
50
60
Age
(a) Original
1
0.8
0.6
0.4
1
0.8
0.6
0.4
0.2
0.2
0
0
20
30
40
50
60
20
30
40
50
60
Age
Age
(b) Approximated from generalization
(c) Approximated from anatomy
Fig. 2.
Original/re-constructed pdf of tuple 1 in Table 3a
tion are analogous to those discussed for the previous case where A1 is true
and A2 is not.
4.6 Correlation Preservation
A good publication method should preserve both privacy and data correlation
(between QI- and sensitive attributes). Using a concrete query, we have shown
in Section 4.2 that anatomy allows more effective aggregate analysis than
generalization. Next, we provide the underlying theoretical rationale.
Obviously, for any tuple
t
T
, every publication method will lose certain
information of
t
(if not, it is equivalent to disclosing
t
directly, contradicting
the goal of privacy). On the other hand, the method should permit devel-
opment of an approximate modeling of
t
(otherwise, the published table is
useless for research). Hence, the quality of correlation preservation depends
on how accurate the re-constructed modeling is.
Let us first examine the correlation between
Age
and
Disease
in the micro-
data of Table 3a. The two attributes define a 2D space
DS
A,D
. Every tuple in
the table can be mapped to a point in
DS
A,D
. For example, tuple 1, denoted
as
t
1
, corresponds to point (
t
1
[
A
]
,t
1
[
D
]), where
t
1
[
A
] is the age 23 of
t
1
,and
t
1
[
D
] its disease 'pneumonia'.
∈
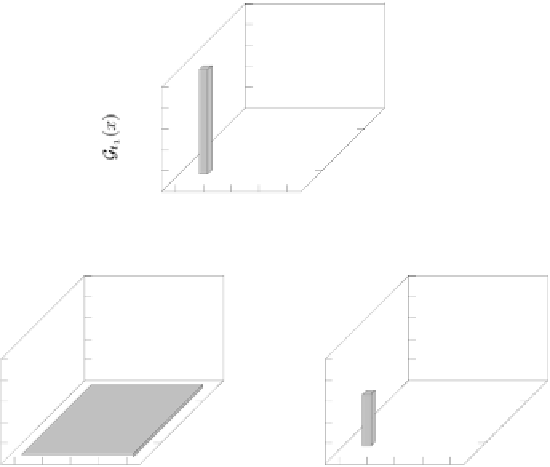



