Geoscience Reference
In-Depth Information
15.6 SUMMARY
A total of 43 LC maps from two study sites were used to demonstrate the effects of classification
accuracy on the uncertainties or errors of 15 selected landscape indices. The measures of classifi-
cation accuracy used in this study were the overall accuracy and REA. The REA was defined as
the difference between the reciprocals of user's accuracy and producer's accuracy. Under variable
levels of classification accuracy, different landscape indices had different uncertainties or errors.
These variations or errors were explained by both the overall accuracy and REA. Thematic maps
with relatively high overall accuracy and low absolute REA ensured lower uncertainties or errors
of at least several landscape indices. For landscape indices that were sensitive to classification
accuracy, a small increase in classification accuracy resulted in a large increase in their accuracy.
Assuming that the error matrix truly represents misclassification errors, the total areas of different
class categories can be calibrated using the REA index and the accuracy of quantifying or comparing
relative landscape characteristics can be increased.
ACKNOWLEDGMENTS
Thematic LC maps used in this study were partially provided by 23 students from a remote
sensing class offered at Purdue University in 1999. The Cooperative Ecological Research Program
in cooperation with the China Academy of Sciences and the German Department of Science and
Technology provided the TM data used in this study. The authors would like to thank the topic
editors and anonymous reviewers for their insightful comments and suggestions on the manuscript.
REFERENCES
Anderson, J.R., E.E. Hardy, J.T. Toach, and R.E. Witmer, A Land Use and Land Cover Classification System
for Use with Remote Sensor Data, U.S. Geological Survey professional paper 964, U.S. Government
Printing Office, Washington, DC, 1976.
Bauer, M.E., M.M. Hixson, B.J. Davis, and J.B. Etheridge, Area estimation of crops by digital analysis of
Landsat data,
44, 1033-1043, 1978.
Cain, D.H., K. Riitters, and K. Orvis, A multi-scale analysis of landscape statistics,
Photogram. Eng. Remote Sens.,
Landsc. Ecol.,
12, 199-212,
1997.
Card, D.H., Using map categorical marginal frequencies to improve estimates of thematic map accuracy,
Photogram. Eng. Remote Sens.,
48, 431-439, 1982.
Congalton, R.G. and K. Green,
Assessing the Accuracy of Remotely Sensed Data: Principles and Practices
,
Lewis, Boca Raton, FL, 1999.
Czaplewski, R.L., Misclassification bias in areal estimates,
Photogram. Eng. Remote Sens
., 58, 189-192, 1992.
Dymond, J.R., How accurately do image classifier estimate area?
Int. J. Remote Sens.,
13, 1735-1342, 1992.
Elkie, P., R. Rempel, and A. Carr,
Patch Analyst User's Manual, Ontario Ministry of Natural Resources,
Northwest Science & Technology,
Thunder Bay, Ontario, Tm-002, 1999.
Frohn, R.C.,
Remote Sensing for Landscape Ecology: New Metric Indicators for Monitoring, Modeling, and
Assessment of Ecosystems
, Lewis, Boca Raton, FL, 1998.
Gustafson, E.J., Quantifying landscape spatial pattern: what is the state of the art?
Ecosystems
, 1, 143-156, 1998.
Hay, A.M., The derivation of global estimates from a confusion matrix,
Int. J. Remote Sens
., 9, 1395-1398,
1988.
Hess, G.R., Pattern and error in landscape ecology: a commentary,
9, 3-5, 1994.
Hess, G.R. and J.M. Bay, Generating confidence intervals for composition-based landscape indexes,
Landsc. Ecol.,
Landsc.
12, 309-320, 1997.
Lunetta, R.S., R.G. Congalton, L.F. Fenstermaker, J.R. Jensen, K.C. McGwire, and L.R. Tinney, Remote
sensing and geographic information system data integration: error sources and research issues,
Ecol.,
Pho-
togram. Eng. Remote Sens.,
57, 677-687, 1991.
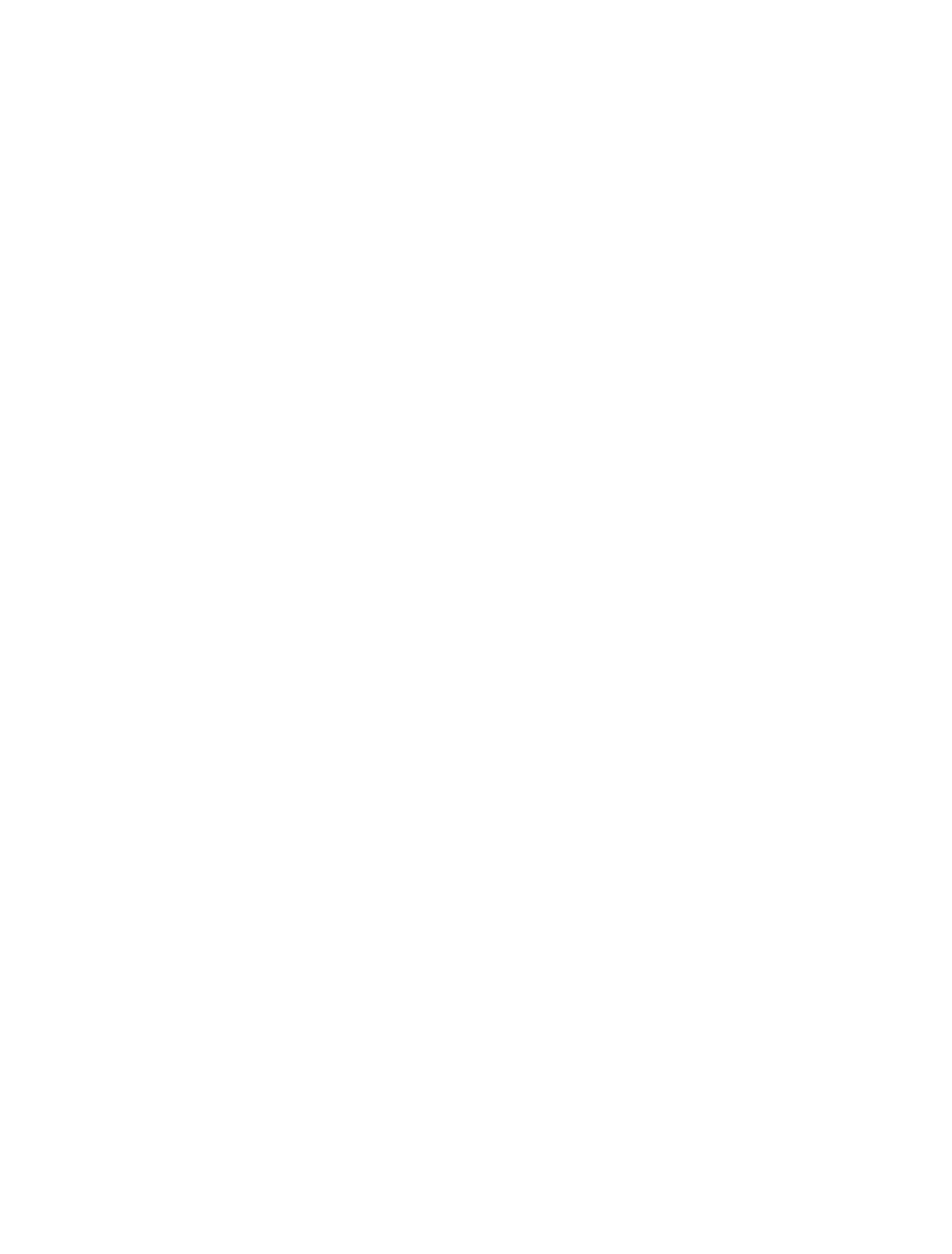














