Geoscience Reference
In-Depth Information
into consideration);
I
is a binary function that equals 1 if the sampled point
k
has the agreement
k
level
i
and 0 otherwise;
p
is the exponent of distance used in the calculation; and
d
is the
k
photographic interpretation confidence score of the sampled pixel
. As information on photographic
interpretation confidence was not available for the Region III data set,
k
d
was set as constant (
d
=
k
k
1) in this study. The division by the maximum of
was to normalize the fuzzy membership function
(Equation 13.1). Verbally, the fuzzy number of multilevel agreement at a mapped pixel defined in
Equation 13.1 is a modified inverse distance weighted (IDW) interpolation of the
A
i
n
nearest sample
points for each agreement level defined in Table 13.2. But instead of using all
n
data points together
in the interpolation, as in conventional IDW for continuous data, the
sample pixels were divided
into six separate groups based on their agreement levels and six iterations of IDW interpolation
(one for each agreement level) were run. For each iteration of a particular agreement level, only
those samples (among
n
sample pixels) with that agreement level would be coded as 1, while other
reference samples were coded as 0 by the use of the binary function
n
I
. IDW then returned a value
k
between 0 and 1 for
M
in each iteration. In other words,
M
is an IDW-based weight of sample
i
i
closest sample pixels surrounding the pixel under study.
With the “winner-takes-all” rule, the agreement level with maximum
pixels at the agreement level
i
among the
n
M
(i.e., maximum membership
i
value
= 1) will be assigned as the agreement level of the mapped pixel under study.
After the multilevel agreement fuzzy set
m
i
was calculated (Equation 13.1), its scalar cardinality
was computed as follows (Bárdossy and Duckstein, 1995):
A
6
Â
m
1
car A
()=
(13.2)
i
i
=
Thus, the scalar cardinality of the multilevel agreement fuzzy set
is a real number between 1 and
6. This is an indicator of the agreement-level “homogeneity” of sampled pixels surrounding the pixel
under study. If
A
) is close to 1, the majority of sampled pixels surrounding the mapped pixel
under study have the same agreement level. Conversely, the greater
car
(
A
) is, the more heterogeneous
in agreement levels the sampled pixels are. Note that there is another way for a mapped pixel to
have a near 1 cardinality. That is when the distance between the mapped pixel and a sampled pixel
is very close compared to those of other sampled pixels, reflecting the local effect in the IDW
interpolation. However, this case occurs only in small areas surrounding each sampled pixel.
car
(
A
13.2.2
Spatial Accuracy Map
Using the above equations, discrete fuzzy sets representing multilevel agreement and their
cardinalities were calculated for all mapped pixels associated with a particular cover type. Then,
the cardinality values of all pixels were divided into three unequal intervals (1-2, 2-3, and > 3).
They were assigned (labeled) to the appropriate category, representing different conditions of
agreement-level heterogeneity of neighboring sampled pixels. The three cardinality classes were
then combined with six levels of agreement to create 18-category accuracy maps.
13.2.3
Degrees of Fuzzy Membership
This step calculated the possible occurrence of multiple cover types for any given pixel(s)
locations expressed in terms of degrees of fuzzy membership. This was done by comparing cover
types of mapped pixels and sampled pixels at the same location based on individual pixels and a
3
3 window-based evaluation. To illustrate, assume that the mapped pixel and the sampled pixel
had cover types
¥
x
and
y
, respectively. In the one-to-one comparison between the mapped and
sampled pixels, if
are the same, then it is reasonable to state that the mapped pixel was
classified correctly. In that case, the degree of membership for cover type
x
and
y
x
to remain the same
is
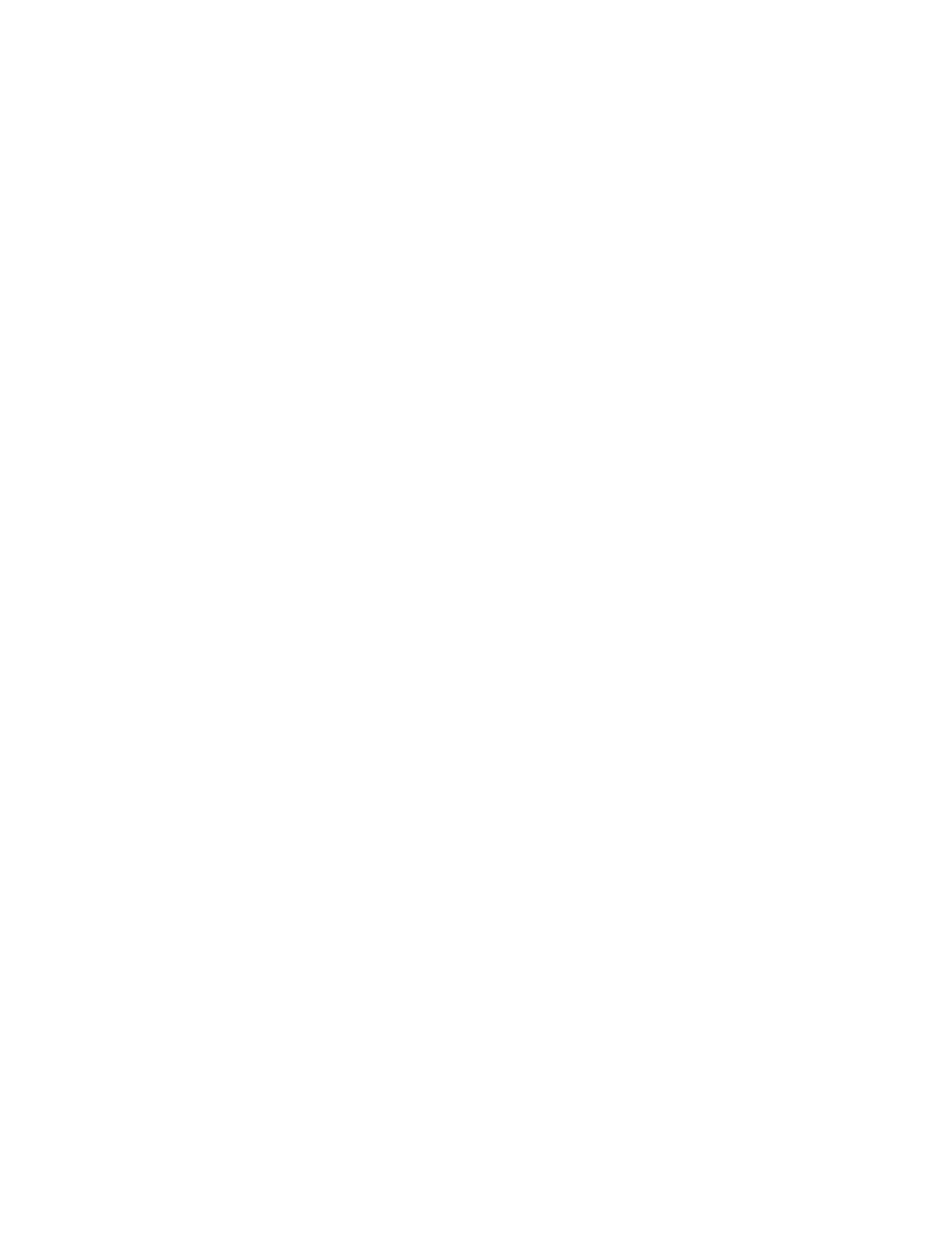














