Geoscience Reference
In-Depth Information
to the prior probability times the ratio of the derived preposterior probability
to the prior probability . Note that this is a congenial assumption whose conse-
quences have not received much attention in the remote sensing literature (and in other disciplines).
Under this assumption, the final posterior probability
p
k
pc
[( )|
uc
]/
p
k
g
k
pc
k
[( )|
uc
]
p
k
g
can be seen as a modulation
pc
k
[( )| ( ),
uxuc
]
g
of the prior probability
by two factors: the first factor
quantifies the influence
p
k
pc
[( )| ( )]/
uxu
p
k
k
of remote sensing, while the second factor
quantifies the influence of the spatial
pc
[( )|
uc
]/
p
k
g
k
information.
Note that, in the above formulation, both information sources are deemed equally reliable, which
need not be the case in practice. Although individual source preposterior probabilities in the fusion
Equation 11.9 can be discounted via the use of reliability exponents (Benediktsson and Swain, 1992;
Tso and Mather, 2001), this avenue is not explored in this chapter due to space limitations.
11.2.4
Mapping Thematic Classification Accuracy
The set of
K
posterior probabilities of class occurrence
derived
{
'
pc
[ ( ) |
uxuc
( ),
], '
k
= 1
,
…
a
()
,
K
}
k
g
at a particular pixel
u
can be readily converted into a classification accuracy value
. If pixel
u
u
is allocated to, say, category
, then a measure of accuracy associated with this particular class
c
k
allocation is simply
, whereas a measure of inaccuracy (error) associated
a
()
u
=
p
[()| (), ]
c
u
x
u
c
kk
'
=
g
with this allocation is
. If such posterior probabilities are available
1
-
a
()
u
=
1
-
p
[()| (), ]
c
u
x
u
c
kk
'
=
g
at each pixel
, any classified map product can be readily accompanied by a map (of the same
dimensions) that depicts the spatial distribution of classification accuracy.
The accuracy value at each pixel
u
posterior probabilities available
at that pixel; different probability values will therefore yield different accuracy values at the same
pixel. Evidently, the more realistic the set of posterior probabilities at a particular pixel
u
is a sole function of the
K
u
, the more
realistic the accuracy value at that pixel. Consider for example, the set of
preposterior probabilities
derived from a conventional maximum likelihood classifier (Section
K
{
'
pc
[ ( ) |
uxu
( )], '
k
= 1
,
…
,
K
}
k
11.2.1) and the set of
posterior probabilities derived from the
proposed fusion of spectral and spatial information (Section 11.2.3). These two sets of probability
values will yield two different accuracy measures
K
{
'
pc
[ ( ) |
uxuc
( ),
], '
k
= 1
,
…
,
K
}
k
g
and
at the same pixel
u
(subscripts
a
c
()
u
a
f
()
u
c
distinguish the use of conventional vs. fusion-based probabilities). It is argued that the use
of contextual information for deriving the latter posterior probabilities yields a more realistic
accuracy map than that typically constructed using the former preposterior probabilities derived
from a conventional classifier (Foody et al., 1992).
and
f
11.2.5
Generation of Simulated TM Reflectance Values
This section describes a procedure used in the case study (Section 11.3) to realistically simulate
a reference classification and the corresponding set of six TM spectral bands. Availability of an
exhaustive reference classification allows computation of accuracy statistics without the added
complication of a particular sampling design.
Starting from raw TM imagery, a subscene is classified into
clusters using the Iterative Self-
Organizing Data Analysis Technique (ISODATA) clustering algorithm (Jensen, 1996). These
L
L
clusters are assigned into
known classes. To reduce the degree of fragmentation in the resulting
classified map, the classification is smoothed using MAP selection within a window around each
pixel
K
(Deutsch, 1998). The resulting land-cover (LC) map is regarded as the exhaustive reference
classification.
Based on this reference classification, the class-conditional joint PDF of the six TM bands is
modeled as multivariate Gaussian with mean and covariance derived from raw TM bands. Let
and
u
o
o
denote the (6
¥
1) vector of class-conditional mean and the (6
¥
6) matrix of class-
m
X
|
k
S
X
|
k
conditional (co)variances of the raw reflectance values in the
k
-th class. Let
and
denote
m
X
S
the (6
¥
1) mean vector and (6
¥
6) covariance matrix, respectively, of the above
K
class-conditional
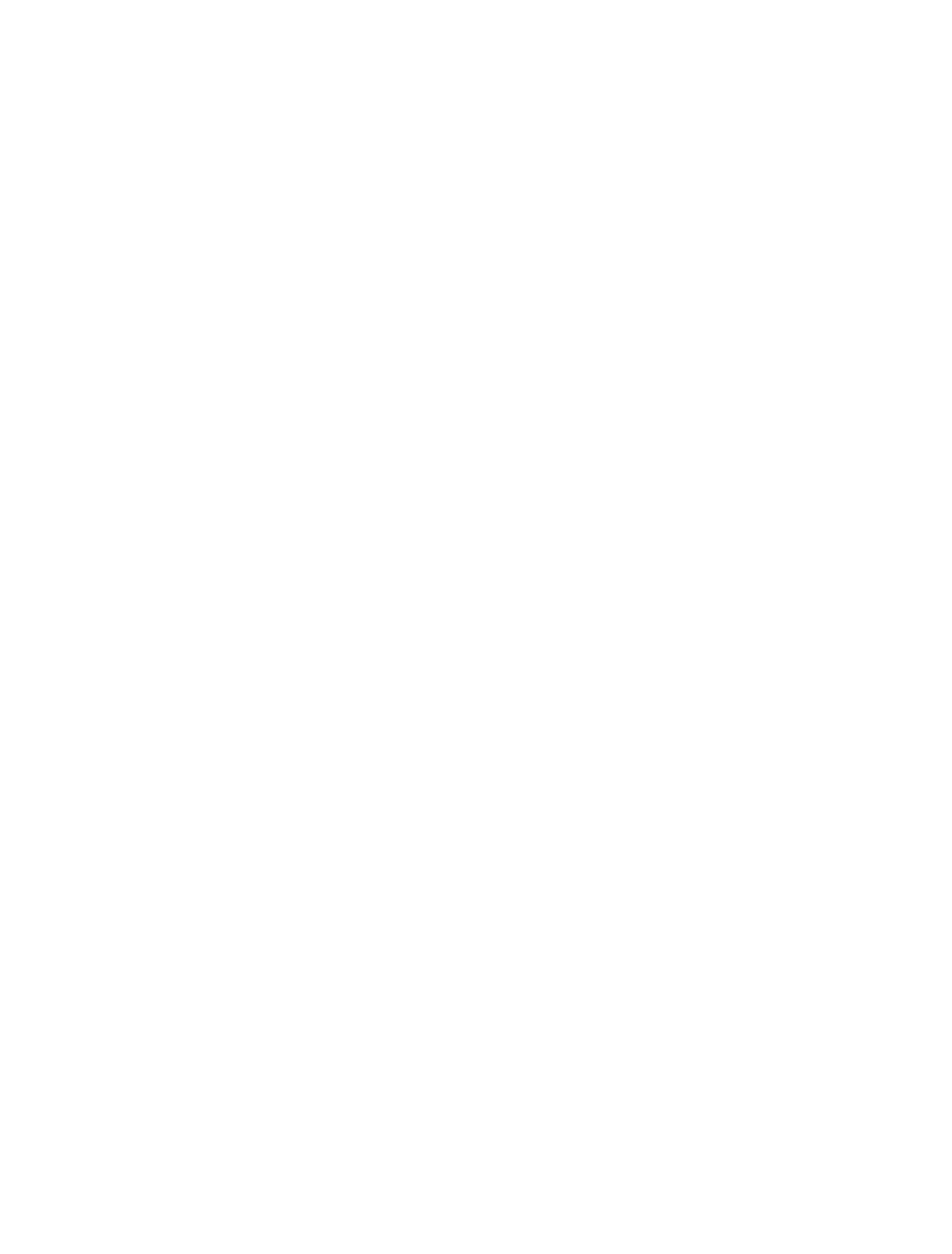














