Information Technology Reference
In-Depth Information
Figure 5.6
Mean Calibration of Expressiveness (E) and Visibility (V) for Higher and Lower
Problem Novelty
5-10, F
(1,36)
0.9), so the data was collapsed over the problem content scenarios. In
terms of percentage correct, these results are identical to those found by Bauer and Johnson-Laird
(1990) who also collapsed the data over the same people-and-places and electric circuit scenarios.
The mean of user calibration of this pooled data is shown for expressiveness and visibility for the
two problem novelty categories in Figure 5.6, with the calibration mean printed in each bar. Recall
that perfect calibration is zero, thus, the shorter the bar, the better the performance. Likewise, prob-
lem novelty is categorized from lower, calibration based on the last six questions answered by each
subject, to higher, where the first four questions were used to compute calibration.
For the visibility (V) treatment, Figure 5.6 shows that average user calibration was overall the
best, closest to zero, at the higher category of problem novelty (.078). The next best level of user
calibration was at the visibility (V) lower category of problem novelty (.100). Comparing these
results, the data suggest that the same subjects exposed to the visibility (V) treatment produced
better user calibration in the first four tries (.078), when problem novelty was highest, than they
did over the last six tries (.100) when problem novelty was lower.
For the expressiveness (E) treatment, the results in Figure 5.6 show that subjects exposed to the
expressiveness (E) treatment had on average poorer calibration than did those exposed to visibil-
ity. Expressiveness produced the poorest average user calibration at both the higher and lower cat-
egories of problem novelty (.163 and .116). However, comparing the two expressiveness (E) bars
shows that there was a marked improvement in user calibration from the higher to the lower cat-
egory of problem novelty for those exposed to expressiveness (.163 to .116). In this regard, the
change in user calibration for those exposed to the expressiveness treatment was as might be
expected: user calibration improved as problem novelty decreased.
To assess the statistical significance of the differences in user calibration suggested by the
means depicted in Figure 5.6, a multivariate analysis of variance (MANOVA) was computed using
the two dependent variables, user calibration at the higher and lower groupings of problem novelty,
.01, p
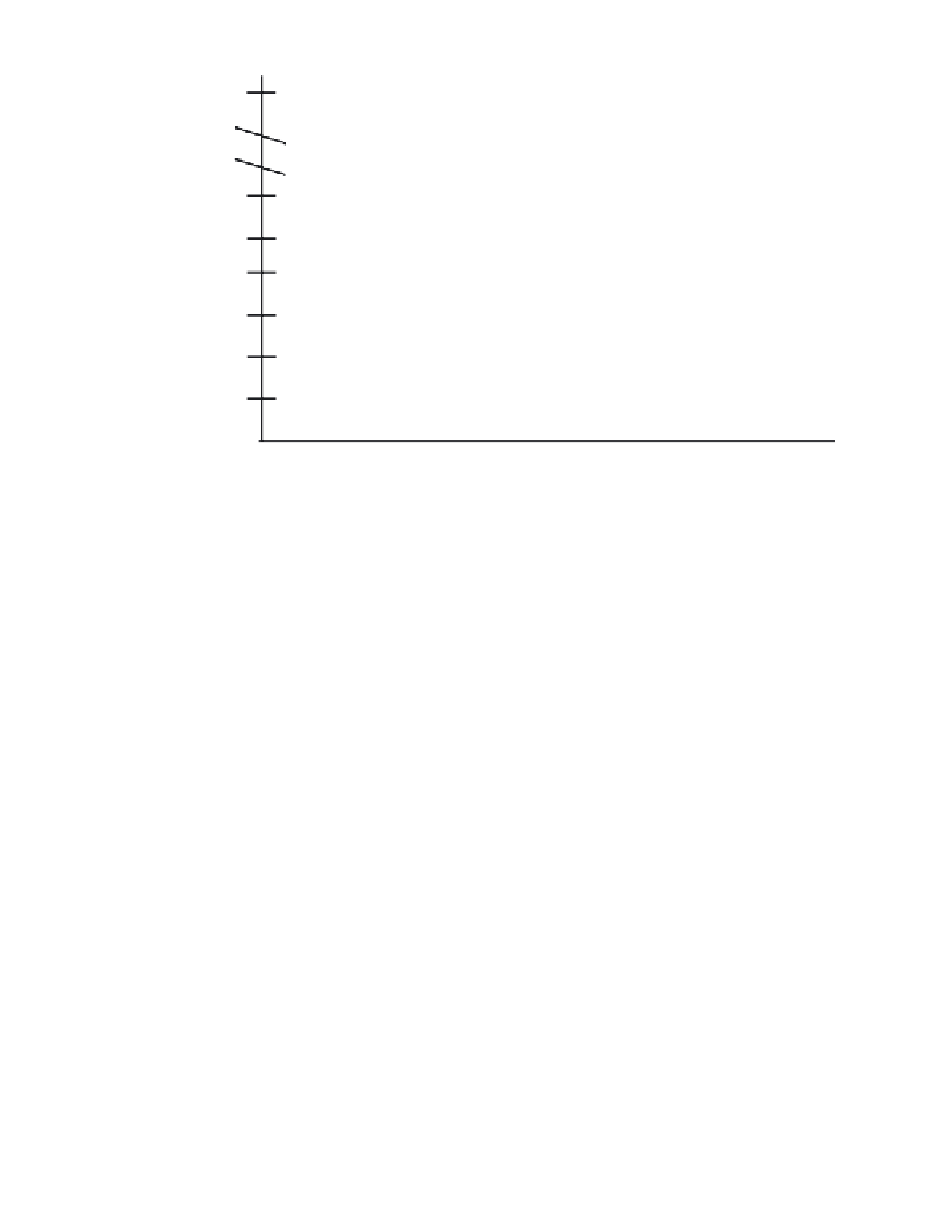

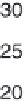







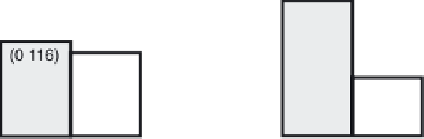



























Search WWH ::

Custom Search