Information Technology Reference
In-Depth Information
no justification do not support user calibration because they fail to expose assumptions or bring to
the user's attention information on the criticality of assumptions, nor do they suggest to the deci-
sion maker new and alternative views of the situation. Advice-giving systems encourage miscali-
bration because they place the user in the paradoxical and extremely difficult role of monitoring,
and overruling when appropriate, the recommendations of a machine whose competence is pre-
sumed to exceed that of the user (Muir, 1987). In fact, the evidence suggests that ratification of a
recommended action, such as occurs when a dialog highlights, prompts, or otherwise directs or
gives advice to the user, not only increases overconfidence but also results in poorer decisions.
Research indicates that when evaluating another's answers, humans are both less correct and
more overconfident than the original respondent (Block and Harper, 1991; Sniezek et al., 1990).
In other words, those cued to a particular response or decision are likely to be more overconfident
about the correctness of the decision than was the person who made the decision in the first place.
Block and Harper (1991) found similar results: subjects who judged the quality of someone else's
answers were both more overconfident and less correct than those not cued to a particular answer.
These results suggest that for DSS dialog design, the actions of highlighting and prompting, and
perhaps even the recommendation of specific decisions by expert systems, may actually con-
tribute to miscalibration. This is because they place the user in the position of having to evaluate
the quality of a recommendation that he or she has had little or no part in developing and that was
made by a machine touted as having more expertise.
Near the contrarian end of the inquirability continuum, the actions of a DSS dialog are designed
to engage and challenge the decision maker's positions and assumptions to reveal and resolve
meta-knowledge through the dialectic process of debate and resolution. Because of this, inquirabil-
ity based on the dialectic model of reasoning is perhaps the most effective for user calibration and
decision performance. In dialectic inquirability, the actions of a DSS dialog are designed to reveal
and resolve metaknowledge by debating a plan (thesis) and counterplan (antithesis) (Mason, 1969).
Conceptually, the revealing and resolving of metaknowledge, characteristic of the dialectic method,
is essential for user calibration when problem novelty is greatest. Cosier and Schwenk (1990) review
the literature on disagreement in decision making and conclude that dialectic reasoning may be the
best method to use when problem novelty is great. Likewise, Cosier and Schwenk (1990) and Mason
(1969) demonstrate that dialectic reasoning results in better decisions, and Buyukkurt and Buyukkurt
(1990) show that dialectic reasoning affects decision confidence.
The literature suggests that dialectic reasoning improves calibration. Central to Faust's (1986)
suggested rules for improving calibration is the generation of evidence that disconfirms, chal-
lenges, and refutes one's position (i.e., dialectic reasoning). Koriat et al. (1980) found that memory-
based knowledge that challenges or contradicts one's decision improves calibration. The need to
justify one's decision to others has also been shown to improve calibration (Arkes et al., 1987).
Each of these variants of dialectic reasoning improves decision-making performance and user cal-
ibration by revealing and resolving inconsistencies, by challenging, refuting, and or disconfirm-
ing one's prior mental representation.
Ultimately, effective outcomes depend upon the user's interpretation of the quality of decisions
he or she makes using the DSS. For user calibration, this means that the DSS must support and
facilitate the user's interpretation of the quality of decisions made using the aid. At a minimum,
the symbols and actions of the DSS dialog should coincide with those of the user's natural system
of problem solving. Expressiveness, visibility, and inquirability define DSS dialog categories of
symbols and actions that parallel those theorized to constitute the user's mental system of prob-
lem solving/decision making. DSS design requires the judicious and effective application of the
DSS dialog symbols and actions of expressiveness, visibility, and inquirability characteristics.
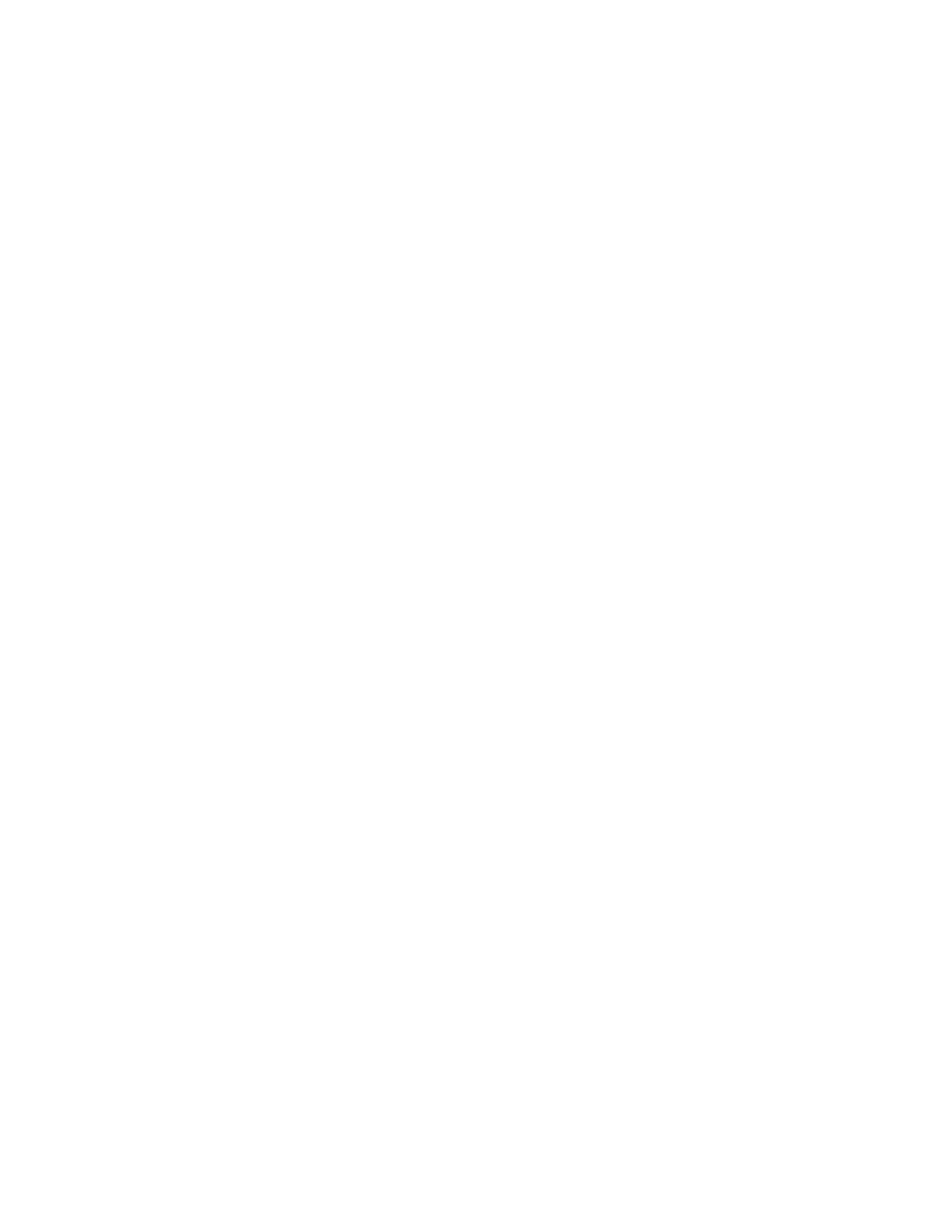
Search WWH ::

Custom Search