Geoscience Reference
In-Depth Information
/(9(/
YDULDEOHV
PHUJHGYDULDEOHVYDULDEOHVUHWDLQHG
/(9(/
ILQDOYDULDEOHV
5HWDLQHG
0HUJHGB 0HUJHGB 0HUJHGB 0HUJHGB
$
%
&
&XZW
&RSSP
)HZW
&KDOB:W
6XOB:W
&XZW 0HUJHGB 0HUJHGB
82SSP 0RSSP
$OZW
%RUQB:W
$B6ROB:W
82SSP0HUJHGB 0HUJHGB
6*
3ESSP
6LZW
&KDOFRB:W $B,QVROB:W
6*
$JSSP
=QSSP
.ZW
3\UB:W
$JSSP
$XSSP
/DZW
&DZW
$XSSP
%DGM6
&HZW
3ZW
%DGM6
7LZW
6ZW
&2ZW
Fig. 14.60
Variables used in the limited model. A total of 28 input variables are considered
bivariate relationships between the input data indicated very
few non-linear relationships; therefore, stepwise conditional
transformations are not considered.
These correlation matrices may be poorly conditioned
with few data. Poorly conditioned matrices are the cause of
extreme weights
(λ
i
)
and introduce unwarranted noise in the
predictions. To prevent this, the correlation matrices are
fixed
to improve their stability. This correction is accomplished by
decreasing the values of the off diagonal elements of the ma-
trix, which increases the value of the smallest eigenvalue for
the matrix and increases stability. The minimum eigenvalue
for the correlation matrices was set to 0.05. Twenty-four of
the correlation matrices for the full model required a cor-
rection, 18 of the correlation matrices for the typical model
required a correction and 12 of the correlation matrices for
the limited model required a correction.
The merged variables are a linear combination of
N
(0,1)
variables. Thus, the mean of the merged variables will be
0 but the variance will not be 1. The merged variables are
standardized by the standard deviation determined from the
following classical relationship:
Step 2: Merge variables—reduce 112 input variables to
23 merged super secondary variables
There is a danger
of over fitting the available calibration data if a regression
model is constructed on all 112 input variables. Therefore,
subsets of the input data were amalgamated to construct
super secondary merged variables. These merged variables
are linear combinations of a subset of variables and signifi-
cantly reduces the dimensionality of the problem while also
reducing the risk of over fitting. The selection of subsets is
based on the nature of the measurements; similar rock mea-
surements are kept together.
The merged “super secondary” variables are generated by
assigning weights to each variable:
=
∑
n
Mv
()
λ
ii
i
=
1
nn
=
∑∑
2
σ
(
M v
( ))
λλ
==
Cov v
(
,
v
)
where
n
is the number of variables to be merged based on
the weights from a likelihood calculation. These weights
are generated by solving the corresponding matrix for each
merged variable and for each of the six output variables:
i
j
i
j
i
11
j
Thus, the final merged variable becomes:
∑
∑∑
n
λ
v
ρρ ρ ρ
…
…
λ
ρρ ρ ρ
ii
Mv
()
=
i
=
1
1,1
2 ,1
n
,1
1
0 ,1
n
n
λλ
Cov v
(, )
v
λ
i
j
i
j
i
=
1
j
=
1
=
1,2
2,2
n
,2
2
0,2
Step 3: Merge variables—reduce 23 input variables to
4 merged variables for regression
There are two levels
of variable amalgamation. The first level grouped related
variables into 16 merged variables and retains 7 additional
variables for a total of 23 variables. Figure
14.60
shows the
variables used in the limited model, while Fig.
14.61
shows
the variables used in the regression models.
ρρ ρ ρ
…
λ
1,
n
2,
n
nn
,
n
0,
n
The right-hand side of this equation contains the correlation
between one of the variables of interest and the n input vari-
ables to be merged, while the left hand side is the correlation
between all n variables to be merged.
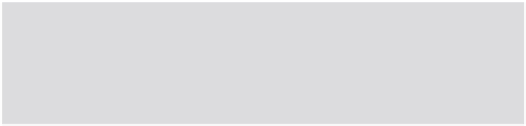

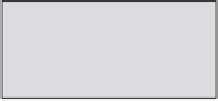
