Biomedical Engineering Reference
In-Depth Information
TABLE 5.2
Design Parameters for Selecting a Transducer for a Biosensor
Parameter
Definition
x
Sensitivity
Ratio of change of sensor output,
. Maximum
sensitivity is normally a design goal as long as other key parameters, such as linearity
and accuracy, are not sacrificed.
∆
y
, to change in the input,
x
:
S
y
Linearity
The constancy of the ratio of output to input. If relationship is linear then
y
Kx
where
K
(also called gain) is a constant. If the sensor is nonlinear then the input-output
relationship is given by an equation:
y
f
(
x
).
Working range
Difference between the maximum and minimum values that can be measured by the
biosensor or sensor element. A large working range is often preferred.
Accuracy
Difference between the measured and the actual values. It can either be defined as an
absolute value which is the maximum error within the working range, or a relative
value which is the ratio of the maximum error to the range of measurement.
Repeatability
Difference in value between two successive measurements under the same
operating environment.
Resolution
Minimum change of input that can be detected at the output of the biosensor. In some
circumstances it is preferred to define resolution as the number of incremental
measurements within the range from minimum to maximum value.
Output
Voltage signal is preferred because microcontrollers and computers are being used to
automatically gather the data. If the proposed biosensor output signal is not a voltage
then it must be converted to an electrical signal with additional hardware, increasing
the source of errors.
Response time
Time required for a change in the input to be observed as a stable output change
(also known as
settling time
). In most cases, the response time is measured from the start
of an input change to the time when the output has settled to the specified range.
The response time of the sensor system is determined by its time constant.
Bandwidth
Under ideal conditions, a transducer would produce identical amplitude responses for
input signals with the same amplitude, independent of their constituent frequencies.
However, the output of a physical transducer is dependent on the amplitude and
frequency of an input signal because of the transducer's inherent bandwidth limitations.
This characteristic is often described as the transfer function in the frequency domain.
architectures with self-learning capabilities were reviewed. The discussion focused on the
general principles of these artificial neural networks and how these algorithms can be
applied to biosensor calibration, pattern classification, analyzing patterns generated by
multiple detectors, and representing complex numeric datasets for enhanced scientific
visualization. Illustrations of function approximation for calibration and unsupervised
clustering were achieved using RBF networks and SOFMs, respectively. Although this
overview focused on algorithms taken from the neural network literature, other more clas-
sical functional approximation or innovative pattern classification techniques may also
have worked. The choice depends on the quality of biosensor data and application goals
being addressed. All these issues play a greater role in capturing and analyzing data and
signals from modern biosensors.
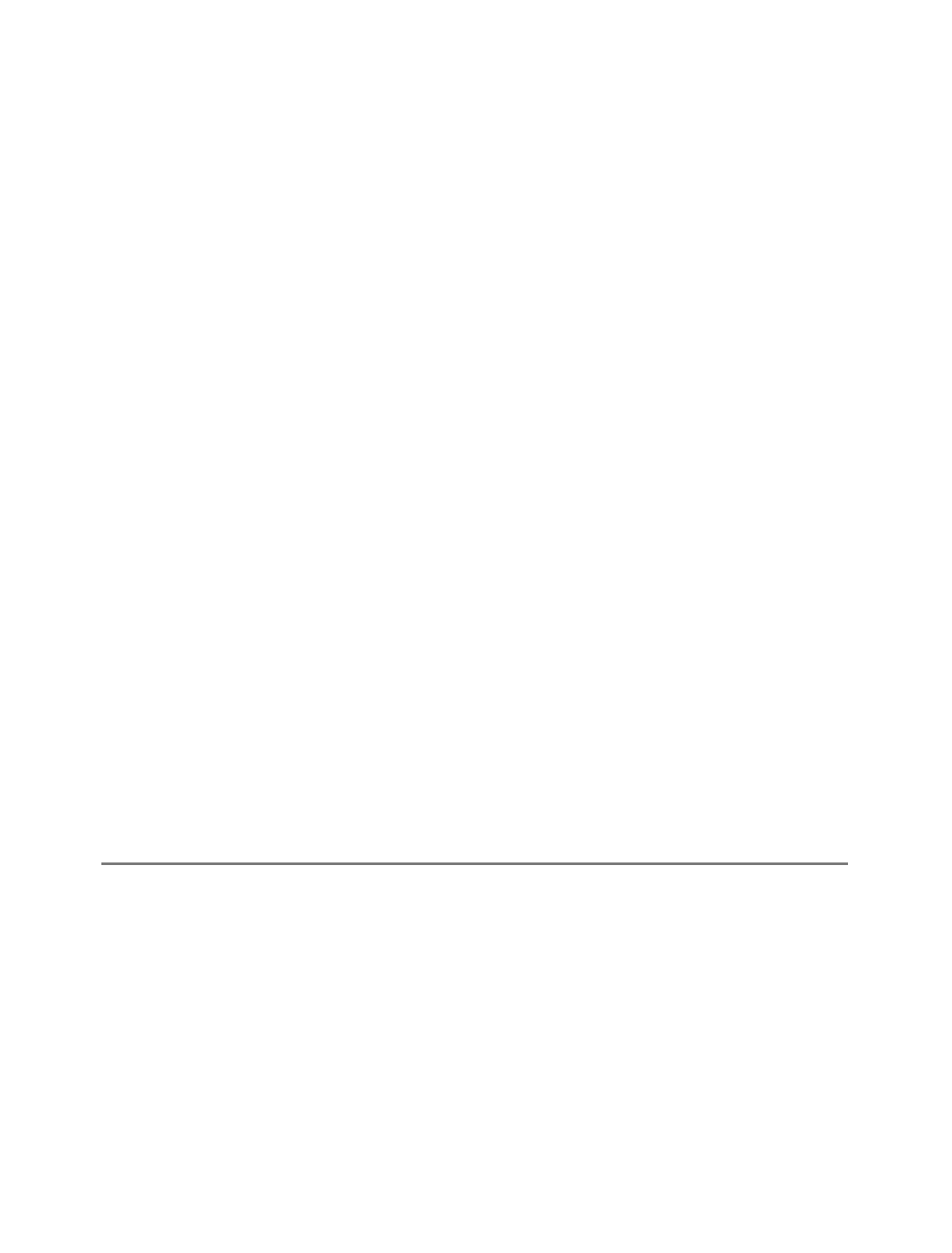
