Biomedical Engineering Reference
In-Depth Information
2500
2000
568 nm
530 nm
520 nm
488 nm
476 nm
1500
1000
500
0
0
5
10
15
20
25
Laser power (mW)
FIGURE 5.6
The intensity-dependent photoelectric response of bR photocell measured using a tunable argon or krypton laser
system with selectable wavelengths at 476, 488, 520, 530, and 568 nm. The power of the laser system is in the
range of 0.65 mW to 37.4 mW.
separated into training and testing data. Typically, 10-14% of the sampled data pair is ran-
domly removed from the dataset for testing and validation. For this very limited dataset
only 10% were removed for testing. The dual-input single-output system is modeled using
a RBF network with two external inputs, 25 basis nodes in layer 1, and only one feedfor-
ward node in layer 2.
During the initial learning phase, the basis nodes in the first layer are fed with a ran-
domly selected input vector
x
p
from the training data. Once the parameters of the basis
function were determined, the nodes in the second layer were adapted using an LMS algo-
rithm. The learning rate is set to 0.1, and the number of cycles through the training dataset
for the LMS adaptation of weights in layer 2 is 10,000. Upon completion, it is possible to
test the resultant model with the remaining test data. The continuous calibration surface
displayed in Figure 5.7 for the bR photovoltage was generated from 21
21 (wavelength,
light power) evenly spaced points along the 3D functional surface. The mean-squared
error in this situation is 0.092.
5.3.2
Pattern Analysis
Many of the pattern classification algorithms exploit supervised or unsupervised learning
methods to assign data samples (8) to the classes. Supervised techniques are used in the
analysis of biosensor data where individual classes of analytes are already known. The
class labeled data is used to train the pattern recognition system to identify specific biosen-
sor inputs or develop a response among the many true and false possibilities. These clas-
sification techniques often test sample features for statistical significance using
K
-nearest
neighbor, logical regression, support vector machines, and artificial neural networks
(9-11). Once the supervised learning phase is complete, the pattern recognition system
must be tested and evaluated for satisfactory performance. The process of validation
involves determining the expected error rate by introducing a new unknown dataset to

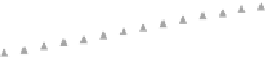

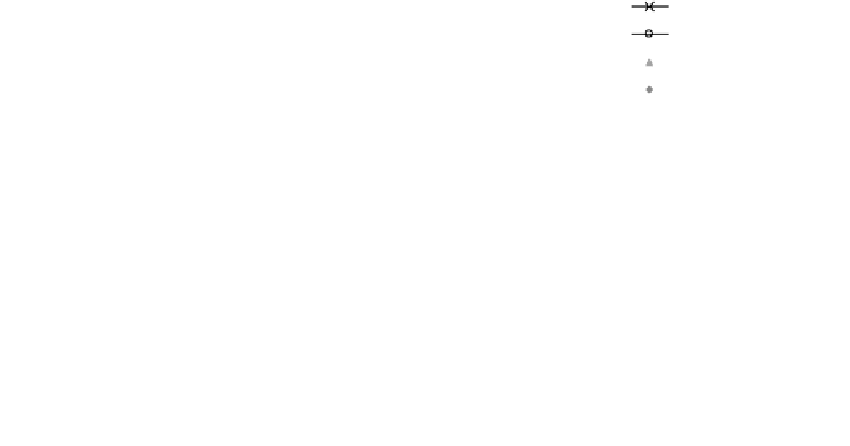





















