Biomedical Engineering Reference
In-Depth Information
achieved for each image are then compared among different methods in order
to illustrate quality differences and to identify superior algorithms.
Computing the accuracy of a segmentation requires a gold standard, or
ground truth. That is, the correct segmentation needs to be known for an image
in order to be able to compute the accuracy of an automatically generated seg-
mentation of that image. While not at all guaranteed to be correct, it is commonly
accepted today to use a manual segmentation by a human expert, supported by
advanced semi-automatic labeling techniques such as intelligent scissors [40],
as the gold standard that automatic segmentation methods are measured
against.
11.5.1
Similarity Index
Figure 11.11 provides a visual impression of the segmentation result for two
representative slices from one segmented bee brain image. However, in order
Figure 11.11: Example of segmentation using non-rigid image registration
(MUL atlas selection paradigm). The two columns show axial images at two
different slice locations.
Top row:
Overlays of segmentation contours (shown
in white) after non-rigid image registration.
Bottom row:
Difference images be-
tween manual and automatic segmentation. Voxels with different labels assigned
by manual and automatic segmentation are shown in black.
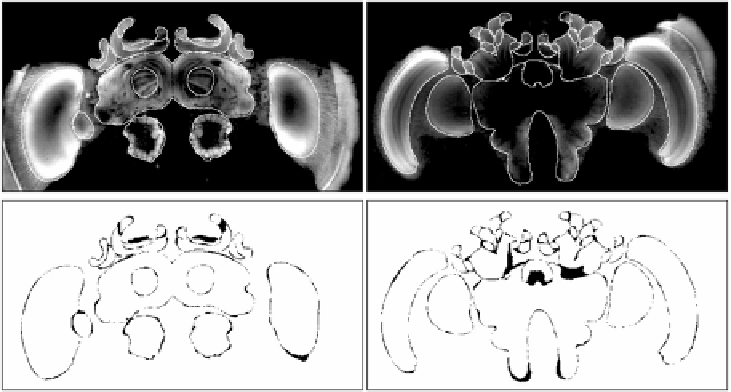