Geoscience Reference
In-Depth Information
choosing a new sample is dependent on knowledge of the local values of likelihood, but where
it is possible to start off a new chain in areas that have not been sampled before.
The most commonly used form of MC
2
sampling is the Metropolis-Hastings algorithm (see
the work of Kuczera and Parent (1998), Engeland
et al.
(2005), Gallagher and Doherty (2007)
and Smith and Marshall (2008) for examples in rainfall-runoff modelling). MC
2
sampling is
an essential part of the BATEA uncertainty estimation methodology of Kuczera
et al.
(2006;
see also Thyer
et al.
, 2009; Renard
et al.
, 2010) and the DREAM approach of Vrugt
et al.
(2008a, 2009; Schoups
et al.
, 2010). A variant is the Gibbs sampler, where the
form
of the
posterior distributions is assumed known (e.g. multivariate Gaussian) but the details of the
posterior marginal distributions for each parameter and any covariance structure are not known
beforehand.
The potential user needs to be aware that the implementation of such schemes needs some
expertise. It is possible to implement them in such a way that the sampling of the posterior
is biased (or “unbalanced”) in important ways (see Renard
et al.
, 2009; Vrugt
et al.
, 2008a).
Improvements to MC
2
sampling strategies for hydrological applications are continuing to be
made (Kuczera
et al.
, 2010b; Vrugt
et al.
, 2008a, 2009; Schoups and Vrugt, 2010). MC
2
sampling has been most often used in the context of defining the shape of posterior likelihood
surfaces in a Bayesian analysis of uncertainty. They can also be used to improve the efficiency
of finding behavioural models within a GLUE analysis (Blasone
et al.
, 2008; McMillan and
Clark, 2009). Recent developments in search algorithms for approximate Bayesian computation
(ABC) might also be usefully applied to some GLUE applications (Sisson
et al.
, 2007; Beaumont
et al.
, 2009; Toni
et al.
, 2009).
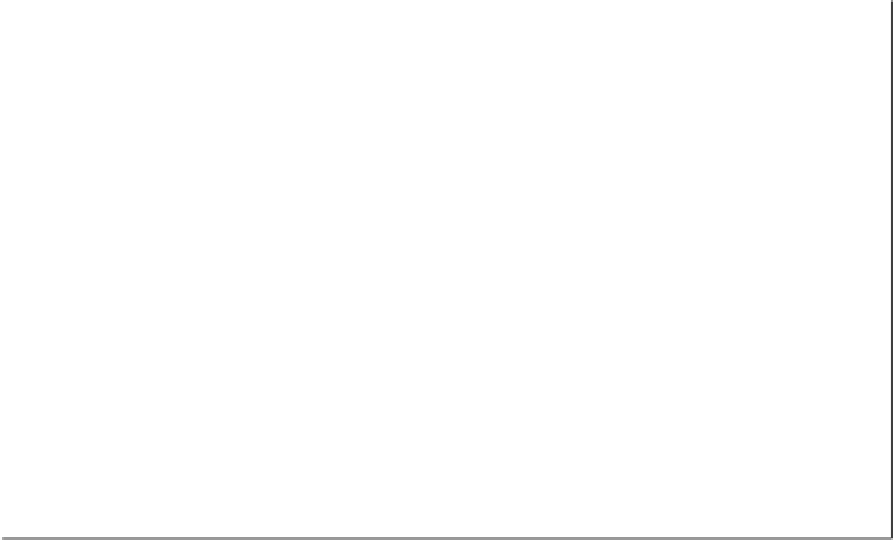