Geoscience Reference
In-Depth Information
Box 7.1 Likelihood Measures for use in Evaluating Models
B7.1.1 Likelihood Measures and Likelihood Functions
The definition of a likelihood function for use in statistical inference is based explicitly on the
expected statistical structure of the errors conditional on the idea that there is a correct (even if
not true) model and that the error is
aleatory
in nature. This is readily appreciated if the simple
example of linear regression is considered. A set of data is observed for two (or more) variables.
A scatter plot suggests that there is a linear relationship between two of the variables. A linear
regression is used to calculate the values of the intercept (
a
) and gradient (
b
) parameters of the
model:
Y
=
a
+
bX
(B7.1.1)
The line does not go through all the data points but the line is calculated so as to minimise
the sum of the squared errors between observed and predicted values of
. The data points
are then in error, relative to the model. The form of the likelihood function used then depends
on assumptions about the nature of the errors (whether they are normally distributed, whether
they are independent or correlated, etc.). A common set of assumptions for the case of linear
regression is that the residuals are independent and identically distributed (iid), with a Gauss
distribution (after some transformation of the
Y
variables if necessary). The theory of linear
regression then provides equations for the identification of
Y
and
X
a
,
b
and their covariance
under
those assumptions
and the uncertainty of any predicted
Y
given a value of the independent
variable
.
The errors associated with a rainfall-runoff model are not quite so simple (actually this is
even true for statistical regressions, see the interesting example provided by Draper, 1995). They
arise from multiple sources, including the model inputs and model structure itself. The data
with which the model is being compared might also be associated with significant observation
error. There is also a tendency for the errors at successive time steps to be correlated, in some
cases with correlation that remains high for many time steps (see, for example, Feyen
et al.
,
2007). Thus, defining a statistical structure for the model residuals or for the individual sources
of uncertainty is difficult (and Chapter 7 makes the case that many of the errors in the modelling
processes might not be statistical in nature at all).
There have been two approaches within the Bayesian statistical framework of model con-
ditioning. The first has been to base a likelihood function only on the nature of the model
residuals. Thus at any point in space or time, the residual
X
(
x, t
) can be defined as:
(
x, t
)
=
O
(
x, t
)
−
M
(
, I, x, t
)
(B7.1.2)
where
O
(
x, t
) is an observed value and
M
(
, I, x, t
) is the prediction of a model with parameter
set
. In this form, the error is assumed to be additive. A multiplicative error can
also be easily implemented in this framework by taking logs of the values
and inputs
I
).
It is then common to follow Gauss and base the contribution of a single residual to the
likelihood as being proportional to the square of its value so that:
O
(
x, t
) and
M
(
, I, x, t
2
−
L
(
|
M
(
, I
)) ∝
exp
(B7.1.3)
2
such residuals, assuming independence, the individual contributions
combine multiplicatively so that
Over a time series of
N
N
−
2
L
(
|
M
(
, I
))
∝
exp
(B7.1.4)
2
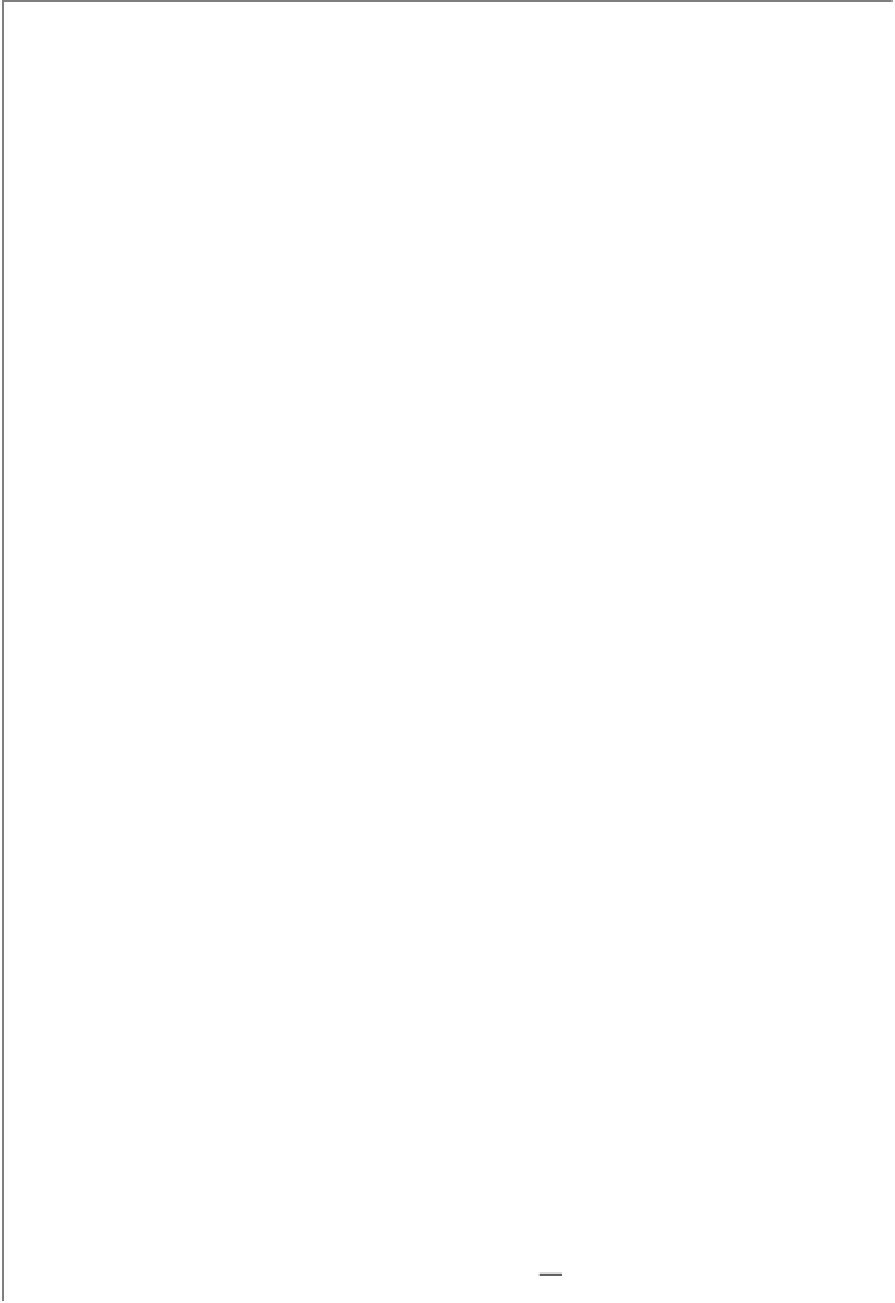