Geoscience Reference
In-Depth Information
2.3.2. Seasonal habitat availability.
Recognizing the po-
tential importance of the seasonal separation of sea ice cover
from preferred continental shelf foraging areas, and duration
of such separation, we determined the number of ice-free
months over the continental shelf and the average shelf-
to-ice distance in both the observed and GCM-projected ice
concentration maps. An ice-free month occurred in an ecore-
gion when <50% of the shelf area was covered by sea ice
of ≥50% concentration. Shelf ice distance was the mean
distance from every shelf pixel in a polar basin ecoregion
to the nearest ice-covered pixel (>50% concentration) dur-
ing the month of minimum ice extent. This described how
far polar bears occupying sea ice habitats would be from
their preferred continental shelf foraging areas. The average
shelf-to-ice distance was not calculated for the SIE and AE
because we considered those ecoregions to be composed en-
tirely of shelf waters.
BNs). BNs are “solved” by specifying the values of input
nodes and having the model calculate posterior probabilities
of the output node(s) through “Bayesian learning” [
Jensen
,
2001]. BNs are useful for modeling systems where empiri-
cal data are lacking, but variable interactions and their un-
certainties can be depicted based on expert judgment [
Das
,
2000]. They are also particularly useful in efforts to synthe-
size large amounts of divergent quantitative and qualitative
information to answer “what if” kinds of questions.
Developing a BN model entails depicting the “causal web”
of interacting variables [
Marcot
et al.
, 2006] in an influence
diagram, assigning states to each node, and assigning prob-
abilities to each node that define the conditions under which
each state could occur. We used the modeling shell Netica®
(Norsys, Inc.) and followed guidelines for developing BN
models developed by
Jensen
[2001],
Cain
[2001], and
Mar-
cot
et al.
[2006].
Our BN stressor model was based on the knowledge of
one polar bear expert (S. Amstrup), who established the
model structure and probability tables according to expected
influences among variables. B. Marcot served as a “knowl-
edge engineer” and provided guidance to help structure the
expert's knowledge into an appropriate BN format. Amstrup
compiled an initial list of ecological correlates which were
organized into an influence diagram (Plate 2). With discus-
sion and questioning, Marcot guided Amstrup through sev-
eral stages to a final model structure.
The BN model structure was divided into three kinds
of nodes: (1) input nodes were anthropogenic stressors
or environmental variables, states of input nodes were
parameterized with unconditional probabilities; (2) sum-
mary nodes, sometimes called latent variables [e.g.,
Bollen
, 1989], collect and summarize effects of multiple
input nodes, states of these were parameterized with con-
ditional probability tables; and (3) output nodes that rep-
resented numerical, distribution, and overall population
responses to the suite of inputs. Probabilities of the vari-
ous states of output nodes are derived through Bayesian
learning. We developed the model structure in an iterative
fashion adding variables for which we could hypothesize
important roles. Published as well as unpublished informa-
tion on how polar bears respond to changes in sea ice al-
lowed us to parameterize the model to ensure it responded
to particular input conditions in ways that paralleled re-
sponses of polar bear populations that have been observed
or for which there are strong prevailing hypotheses among
polar bear biologists worldwide.
To assure our outcomes were relevant to the question
whether to list polar bears as a threatened species, we
designed the summary nodes in the BN model to include four
of the five major listing factors used to determine a species'
2.4. Bayesian Network Population Stressor Model
A Bayesian network is a graphical model that represents a
set of variables (nodes) linked by probabilities [
Neopolitan
,
2003;
McCann
et al.
, 2006]. Nodes can represent correlates
or causal variables that affect some outcome of interest, and
links define which specific variables directly affect which
other specific variables. BNs can combine expert knowl-
edge and empirical data into the same modeling structure.
Crafting a BN augments understanding of relationships and
sensitivities among the elements of a causal web and pro-
vides insights into the workings of the system that otherwise
would not have been evident. BNs have become an accepted
and popular modeling tool in many fields [
Pourret
et al.
,
2008] including ecological and environmental sciences [e.g.,
Aalders
, 2008;
Uusitalo
, 2007]. Each node in a BN model
typically has two or more mutually exclusive states, the
probabilities of which sum to one. Prior probabilities are dis-
tributed as discontinuous Dirichlet functions in the form of
D(
x
) = lim
cos
2
n
(
m
!p
x
), which is a multivariate, n state
generalization of the two-state Beta distribution with state
probabilities being continuous within [0,1]. BN nodes can
represent categorical, ordinal, or continuous variable states
or constant (scalar) values and typically have an associated
probability table that describes either prior (unconditional)
probabilities of each state for input nodes or conditional
probabilities of each state for nodes that directly depend on
other nodes (see
Marcot
et al.
[2006] for a description of
the underlying statistics). States
S
of output nodes contain
posterior probabilities that are calculated conditional upon
nodes
H
that directly affect them, using Bayes theorem, as
P
(
S
|
H
) = [
P
(
H
|
S
)
P
(
S
)/
P
(
H
)]
(see
Jensen
[2001] and
Mar-
cot
[2006] for further explanation of the statistical basis of
m
®¥
lim
n
®¥
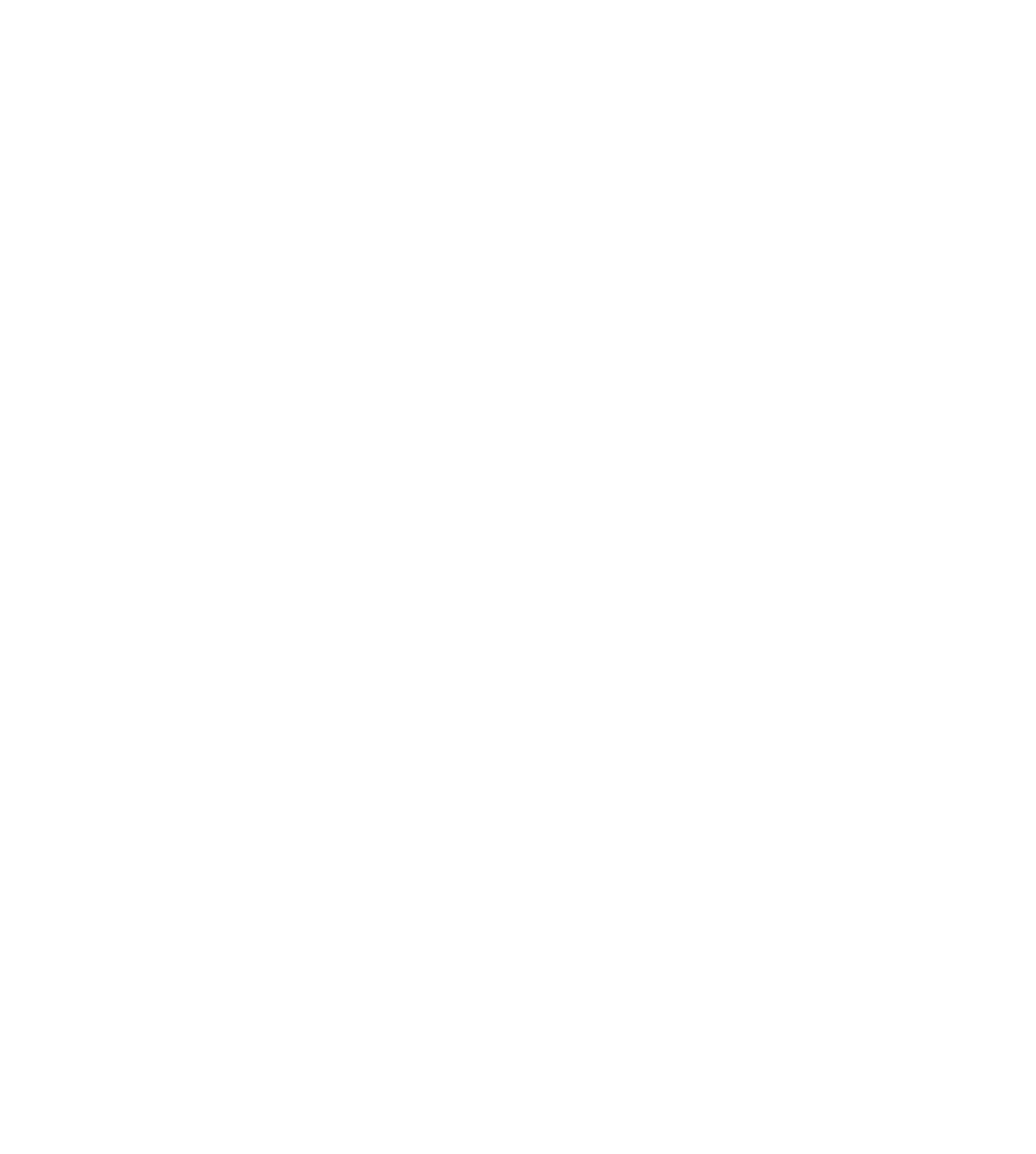
Search WWH ::

Custom Search