Agriculture Reference
In-Depth Information
0
5
10
15
20
25
30
35
Soil Temperature (
o
C)
Figure 3.1
A graphical representation of Jensen's inequality using the example of the nonlinear
relationship between soil temperature (°C) and relative soil microbial activity (arbitrary units).
Here, the simplest case of a heterogeneous system is represented by two states (conditions), 20°C
and 30°C (the dashed black lines). Applying the nonlinear function to each state and then finding
the average relative activity level (27.5, indicated by the short dashed black horizontal line) yields a
approximately 13% higher activity level than when the function is evaluated at the mean soil tem-
perature (25°C, indicated by the solid gray line). The difference between the activity level estimates
for the heterogeneous and uniform soil temperatures (~13%, indicated by the bracket and arrow) is
referred to as the aggregation bias.
I was applying log transformations to data to meet the homogeneity of variance assumption
of an analysis of variance (ANOVA) test and then transforming the means back into a linear
scale for visualization purposes. However, I realized that the back-transformed means were
not equal to the original means. Not understanding this difference, I sought guidance and
was eventually pointed to Jensen's inequality to learn why the transformation process had
biased my results. For lack of a more precise term, I refer to the estimation bias due to Jensen's
inequality (either an over- or an underestimate) as an aggregation bias. It is important to note
that the term
aggregation bias
is also used in a similar but more specific manner in statistics
when discussing the level of inference appropriate for a given data set (Freedman et al., 1998).
Aggregation bias observed when studying nonlinear functions is influenced by sev-
eral components of a functional relationship between environmental variables (or resource
abundance) and process rates, including (1) the type of function used to describe the rela-
tionship, (2) the sign and magnitude of the function constants, (3) the variance around
the mean predictor level (e.g., diel oscillations in soil temperature), and (4) the range in
which the function is evaluated. For simple monotonic nonlinear functions (e.g., quadratic,
single-parameter power, exponential, logarithm), the direction of the aggregation bias is
systematic (i.e., predictable). For example, the exponential relationship between soil tem-
perature and soil metabolic (or enzymatic) activity is an accelerating monotonic function
for which the aggregation bias is always negative (i.e., the mean soil temperature will
always underpredict the soil microbial activity relative to the unaggregated estimate). For
monotonic functions, I recall the direction of the aggregation bias because it is opposite
of the sign of the second derivative (or a close approximation thereof). For decelerating












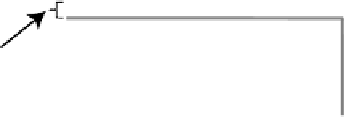
















Search WWH ::

Custom Search