Biomedical Engineering Reference
In-Depth Information
FIGURE 4.31:
The time-frequency maps obtained by modified Morlet wavelet.
a) ECG of healthy subject; b) the same signal with simulated late potentials. Time
and frequency ranges for irregularity factor calculation are marked by straight lines.
From [Lewandowski et al., 2000, Fig. 2].
signal representation by means of principal components (Sect.3.6.1). Representing
ECG morphology by means of PCA provides robust estimates in terms of few de-
scriptive parameters and allows for effective comparison of normal and deviating
ECG pattern vectors exposing differences between them. In the procedure the noisy
outliers may be eliminated on the basis of criteria concerning normalized residual
error.
The application of PCA in ECG signal analysis included compression, filtering
and QRS complex classification [Moody and Mark, 1990], shape representation of
ECG morphology [Taddei et al., 1993], analysis of repolarization ST-T segment [La-
guna et al., 1999], detection of transient ST-segment episodes during ambulatory
ECG monitoring [Jager et al., 1998].
4.2.1.3.5 ECG patterns classification
ECG feature extraction serves diagnostic
purposes and is usually followed by the classification procedures. Artificial intel-
ligence methods such as artificial neural networks are helpful in this respect. Dif-
ferent kinds of ANN including multilayer perceptrons and support vector machine
classifiers have been used for ECG classification; training of networks may be per-
formed by means of supervised or non-supervised learning. Introduction to super-
vised and unsupervised learning for ECG classification may be found in [Osowski
et al., 2006, Wang and Azuaje, 2006].






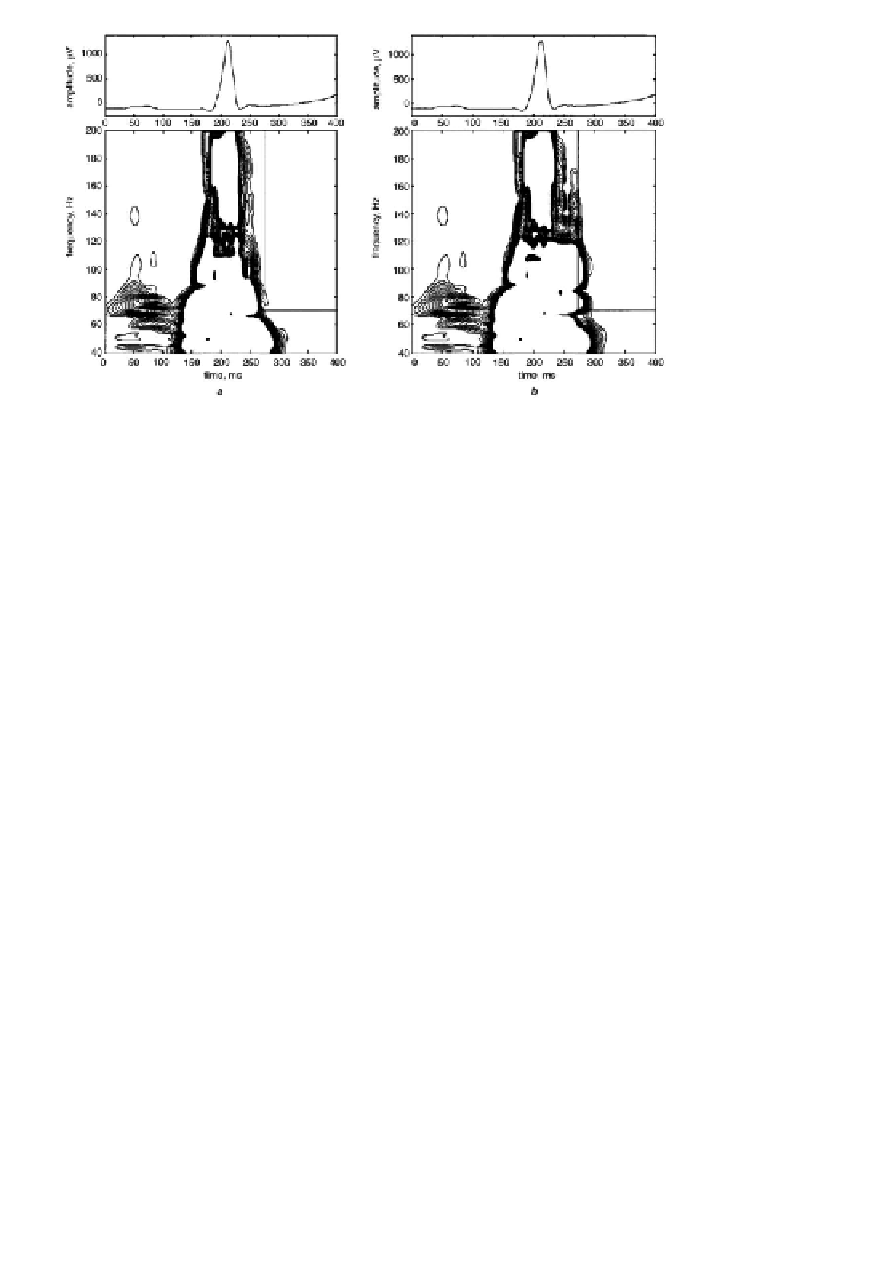
Search WWH ::

Custom Search