Geography Reference
In-Depth Information
10
17
9
13
4
11
3
15
1
12
19
Unknown
6
8
16
2
5
18
7
14
0
10
20 km
Figure 4.7
Locations of observations listed in Table 4.2.
predicted using inverse distance weighting. Figure 4.7 shows the locations of the
observations, along with the location for which a prediction will be made. h e weights,
obtained using the inverse distance weighting scheme detailed above (with exponents
of 1, 2, and 3), are given for each distance. h e weights for each location are then mul-
tiplied by the value at that location. As an example, following Equation 4.4 (and with
weights as dei ned in Equation 4.3) for an exponent of 1, the products of the multipli-
cations are summed, giving a value of 27.582. h e weight values are also summed,
giving a value of 1.347. h e weighted mean is then obtained as
27.582
/
1.347
= 20.474.
h e mean obtained without geographical weighting (i.e. with all weights equal to 1)
is 19.579.
Weighted means (or other statistics) can be calculated anywhere: at the location
of an observation or anywhere else. Section 8.4 demonstrates the geographically
weighted mean using another weighting scheme. Fotheringham
et al.
(2002) discuss a
range of geographically weighted statistics. Geographical weights will be encountered
throughout this topic.
Selection of a weighting scheme is usually arbitrary, but there may be characteristics
of a data set that guide selection. In essence, the choice of weighting function (and,
where relevant, parameters like the bandwidth, see Sections 7.3 and 8.4) should be
determined either through experimentation (e.g. for interpolation, which weighting
function leads to the most accurate predictions) or knowledge of the process of
interest—if we know something about the scale of variation (see Section 2.7) this may
inform our choice of weighting scheme.
h e focus now moves from measurement of distances to characterizing the spatial
structure of values (i.e. how similar neighbouring values are to one another).
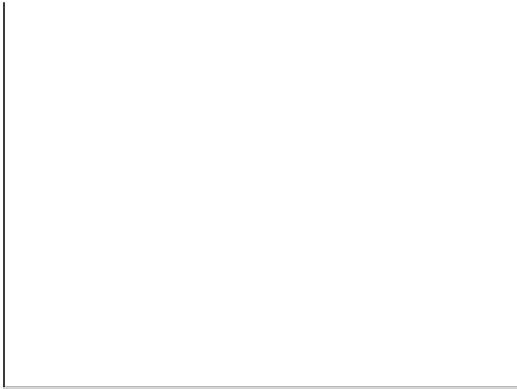




















Search WWH ::

Custom Search