Information Technology Reference
In-Depth Information
1
§ ·
t
E
\
()
t
\
¨
© ¹
,
DE
,
D
D
whereby \ is a fixed time-frequency function meeting the restrictions
1
\J
H
()
t
d
1
t
and
\Z J Z
1
H
()
d
1
,
where (
\Z represents the Fourier transform of
)
\ and H meets the condition
( )
H > 0.
10.3.2 Wavelet Neural Networks
The wavelet decomposition approach, formulated at the end of 1980s, became a
powerful tool for function approximation, and it was also applicable to time series
analysis. Based on this decomposition, some structural representations of wavelet
neural networks have been developed. In the first half of the 1990s, a number of
publications reported on the synthesis and applications of wavelet neural networks
(Zang and Benveniste, 1992; Pati and Krishnaprasad, 1993; Zhang
et al.
, 1995).
The initial idea of Zang and Benveniste (1992) was to depict the wavelet neural
network as an approximator of continuous functions using the universal
approximation capability of wavelet decomposition.
-t
1
w
1
R
1
D
1
\
g
-t
2
w
2
R
2
D
2
\
x
g
(
x
)
:
:
:
:
:
:
:
:
-t
N
w
N
R
N
D
N
\
Figure 10.6.
Wavelet network as a function approximator
Hence, they proposed a neural network structure described by the
decomposition algorithm
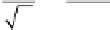









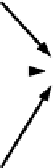














Search WWH ::

Custom Search