Information Technology Reference
In-Depth Information
Table 6.2(b).
Comparison of training and prediction performance of fuzzy and other model
with selected Takagi-Sugeno-type multi-input single-output neuro-fuzzy network with
proposed Levenberg-Marquardt training algorithm for Mackey-Glass chaotic time series.
Sl. No.
Method
Training /
forecasting with
Input data
RMSE
prediction error
1.
Kim and Kim
(coarse partition)
500
0.050809 (5 partitions)
0.044957 (7 partitions)
0.038011 (9 partitions)
2.
Kim and Kim
(after fine tuning)
500
0.049206 (5 partitions)
0.042275 (7 partitions)
0.037873 (9 partitions)
3.
Kim and Kim
(genetic-fuzzy predictor
ensemble)
500
0.026431
4.
Lee and Kim
500
0.0816
5.
Wang (product operator)
500
0.0907
6.
Min operator
500
0.0904
7.
Jang (ANFIS)
500
0.0070 (16 rules)
8.
Auto regression model
500
0.19
9.
Cascade correlation neural
network
500
0.06
10.
Backpropagation neural
network
500
0.02
11.
Sixth-order polynomial
500
0.04
12.
Linear prediction model
500
0.55
13.
FPNN (quadratic polynomial
fuzzy inference)
500
0.0012 (16 rules)
14.
Takagi-Sugeno-type multi-
input single-output neuro-
fuzzy network (proposed
work)
500 (forecasting)
0.0053 (7 rules,
7 GMFs,
non-optimized)
N
2
RMSE =
,
e
r
is the error due to
r
th training sample and
N
is the number
¦
e
N
r
r
1
of training / predicted data samples.
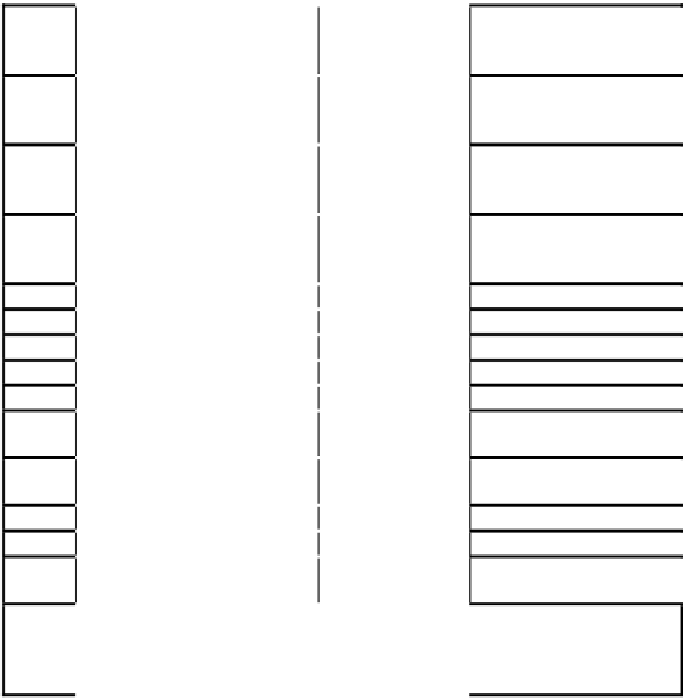
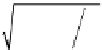




















































Search WWH ::

Custom Search