Information Technology Reference
In-Depth Information
Table 4.3.
Performance of the fuzzy model generated as nonlinear forecasts combiner
Sl.
No.
Forecasts
Data from
HBXIO matrix
SSE / RMSE
1.
Box and Jenkins
151-224
(column-1)
SSE = 0.4516
RMSE = 0.112
2.
Holt's method
151-224
(column-2)
SSE = 0.3174
RMSE = 0.0933
3.
Fuzzy model
(Mamdani), 21 GMFs
1- 150
(training data)
SSE = 0.5155
RMSE = 0.0832
4.
Fuzzy model
(Mamdani), 21 GMFs
151-224
(validation data)
SSE = 0.1680
RMSE = 0.0678
4.9 Concluding Remarks
In this chapter, various fuzzy models, such as the Mamdani model, the Takagi-
Sugeno model and the relational fuzzy model, along with their corresponding
inferencing mechanisms have been described. As the fuzzy inferencing mechanism
relies on a well-consistent set of fuzzy rules, in order to generate the proper output
in response to an unknown input set from the universe of discourse, various rule-
generation algorithms based on Wang and Mendel's approach, or it's modification,
and fuzzy clustering have also been presented in the chapter. The effectiveness of
the fuzzy models generated has been tested on two application examples, namely
the forecasting of chaotic time series and nonlinear plant modelling. In addition, a
fuzzy model has also been applied as a nonlinear forecasts combiner. It is
important to note that the primary objective of using a fuzzy model is to achieve an
inspectable or interpretable model, unlike the black-box model of neural networks.
However, it should be emphasized here that the fuzzy modelling approach
described in this chapter rather primarily focuses on the function approximation
accuracy than the inspectability of the model and, in fact, none of the methods
presented in the chapter can guarantee model transparency issues. Therefore, the
fuzzy model generated is eventually nothing but a replica of a neural-networks-like
model, and needs to be treated further as discussed in Chapter 7, where the primary
attention is paid to the improvement of model transparency.
References
[1]
Babuška R (1996) Fuzzy Modelling for control, Ph.D thesis, Delft University of
Technology, Netherlands.
[2]
Babuška R and Verbruggen HB (1995) Identification of composite linear models via
fuzzy clustering, Proc. of European Control Conference, Rome, Italy, pp. 1207-1212.
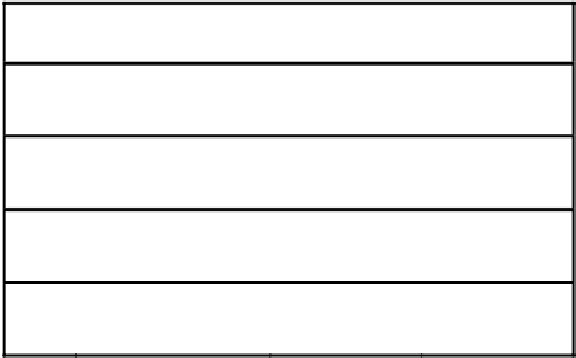
Search WWH ::

Custom Search