Information Technology Reference
In-Depth Information
mcgtry3.m:
f
uzzy-forecast
of Mackey-G
l
ass-Series
1.5
1
0.5
0
550
600
650
700
750
800
850
900
950
1000
time(Second)-(nMF=51 & Training Data =1 to 500)
mcgtry
3
.m: fuzzy pre
d
iction errors
f
or Mackey-G
l
ass Chaotic
S
eries)
0.2
0.15
0.1
0.05
0
-0.05
-0.1
-0.15
-0.2
550
600
650
700
750
800
850
900
950
1000
time(Second)-(nMF=51 & Training Data= 1 to 500)
Figure 4.4(e).
Forecasting chaotic series with fuzzy predictor with
n
= 51 GMFs
Table 4.1.
Forecasting performance comparisons for various fuzzy predictors with 500 to
1000 input data sets (evaluation data) for Mackey-Glass chaotic series
Sl. No.
No. of GMFs
SSE
RMSE
1.
17
0.1252
0.0224,
2.
27
0.0389
0.0125
3.
37
0.0255
0.0101
4.
51
0.0164
0.0081
conventional approaches, like the linear predictive method and Gabor polynomial
method,
etc
. Here, we apply the fuzzy predictor to forecast the future values of
Mackey-Glass chaotic time series data that were directly obtained from MATLAB
version 5.2 as “mgdata.dat” (M
ATLAB
, 1998).
From the numerical data of the chaotic series, a fuzzy logic system capable of
forecasting the future values of the above time series was developed. For this
purpose, neglecting the first 100 transient data points of the chaotic series, with the
remaining data 1000 rows of the
XIO
matrix have been built, out of which the first
500 rows (training data) are used for rules generation and the remaining 500 rows
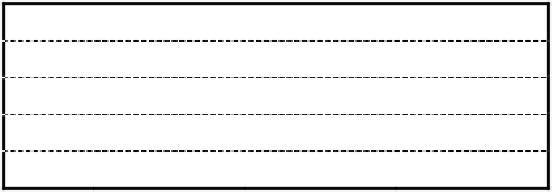
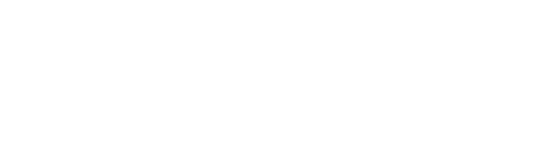
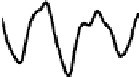

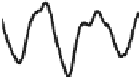











































































































































Search WWH ::

Custom Search