Information Technology Reference
In-Depth Information
information could be considered a semantic representa-
tion.
One influential neural network model of human se-
mantic representations (Farah & McClelland, 1991)
used basic neural network principles to explain a some-
what puzzling pattern of dissociations across patients
with different kinds of semantic deficits. The disso-
ciations involve selective deficits for knowledge about
living things versus nonliving things. Different groups
of patients have deficits in one of these categories,
but are relatively spared in the other (i.e., a
double-
dissociation
; Warrington & Shallice, 1984; Warring-
ton & McCarthy, 1983, 1987). One natural conclusion
from this data is that human semantic information is or-
ganized according to the kinds of taxonomic categories
that one might use in playing 20 questions: e.g., animal,
vegetable, or mineral/man-made (Hillis & Caramazza,
1991).
Alternatively, as first proposed by Warrington and
colleagues and subsequently elaborated by Farah and
McClelland (1991), category-specific semantic deficits
might arise based on the
sensory
versus
functional
asso-
ciations with living versus nonliving things. People pre-
dominantly list sensory features when describing living
things (e.g., “brown,”“large” for a bear), and functional
features when describing tools and other artifacts (e.g.,
“used for pounding in nails” for a hammer) (Farah &
McClelland, 1991). Thus, the living-thing deficit could
be explained by damage to sensory semantic areas, and
the nonliving-thing deficit could be explained by dam-
age to functional semantic areas. This account is much
more consistent with the kind of distributed semantics
envisioned in figure 10.23, which builds off of the well-
known specialization of brain areas for different sensory
modalities.
The simulation of this sensory-functional idea not
only captured the basic dissociation between living and
nonliving things, it also resolved an apparent diffi-
culty for this approach quite naturally. Specifically, the
verbal (non-implemented) sensory-functional account
appeared to mistakenly predict that patients with the
living-thing deficit should still show intact functional
semantics for living things (whereas patients with the
nonliving-thing deficit should still show intact sensory
semantics for nonliving things). This pattern does not
kinesthetic
visual
phone
color
action
oriented
tactile
auditory
phonology
orthography
Figure 10.23:
Illustration of how semantic information
might be distributed across a number of more specific process-
ing pathways, represented here by different sensory modali-
ties. Linguistic representations (orthography, phonology) are
associated with corresponding distributed activation patterns
across semantics. Figure adapted from Allport, 1985.
using the mechanistic principles in Leabra that are well
motivated for a number of other reasons. The complex-
ity of the model and the task(s) it performs may need to
be increased to better fit the human data.
10.6
Semantic Representations from Word
Co-occurrences and Hebbian Learning
Our next model explores the development of the seman-
tic representation component of the distributed lexicon
framework based on statistical properties of the linguis-
tic inputs. As discussed in chapter 7, semantic rep-
resentations, like word representations, are viewed as
distributed throughout various specialized brain areas.
This notion of distributed semantics is well captured
by figure 10.23, adapted from Allport (1985), which
shows the representations of various items (a telephone,
velvet, etc.) across several different specialized pro-
cessing pathways. Although these pathways are rep-
resented only by sensory modality in the figure, a num-
ber of other types of specializations (e.g., chronological,
sequential, task-oriented, episodic, etc.) probably also
contribute to the overall distributed representation of an
item. Indeed, essentially any pattern of activity any-
where in the brain that contributes some item-specific
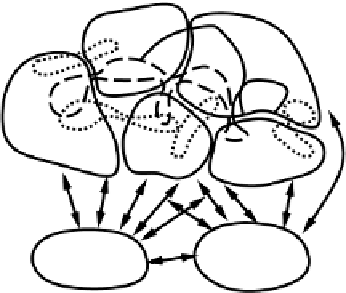

Search WWH ::

Custom Search