Information Technology Reference
In-Depth Information
to observe the preference for producing a given output
over the other possibilities. It is also possible to observe
priming effects on reaction time latencies using a sim-
ilar single-learning-mechanism model
trial
0
1
2
3
4
5
6
7
8
9
10
11
12
13
14
15
16
17
18
19
20
21
22
23
24
25
Event
0_a
1_a
2_a
3_a
4_a
5_a
6_a
7_a
8_a
9_a
10_a
11_a
12_a
0_b
1_b
2_b
3_b
4_b
5_b
6_b
7_b
8_b
9_b
10_b
11_b
12_b
sum_se
5.22935
6.48608
7.77501
7.64788
5.41569
0
10.2454
8.33851
5.64973
10.2408
3.21385
2.82117
4.69916
6.68981
5.40769
7.51547
7.73557
1.94789
0.414954
10.5514
8.79166
9.64561
10.2245
3.53423
7.46935
5.72054
Outp_dist
ev_nm
0_b
1_b
2_b
3_b
4_b
5_a
6_b
7_b
8_b
9_b
10_b
11_b
12_b
0_a
1_a
2_a
3_a
4_b
5_b
6_a
7_a
8_a
9_a
10_a
11_a
12_a
sm_nm
both_err
0
0
0
0
0
0
0
0
0
0
0
0
0
0
0
0
0
0
0
0
0
0
0
0
0
0
0
0
0.273233
0
0.551383
0
0
0
2.61438
0
1.06278
2.42077
0.253711
0
0.330821
0
0
1.94789
0.414954
0
0
0
0
0.766472
0
0
1
1
1
1
1
0
1
1
1
1
1
1
1
1
1
1
1
0
0
1
1
1
1
1
1
1
(Becker et al.,
1997).
These results suggest that in addition to learning
powerful semantic representations, the posterior cortex
can exhibit memory-like effects based on single item
presentations. We will discuss in section 9.7 how these
priming kinds of memory effects can play a role in more
complex memory phenomena by providing a
familiarity
signal. Furthermore, in chapter 10 we explore a model
of inflectional morphology in language production that
takes advantage of the priming and competition exhib-
ited in the present model to explain some previously
thorny phenomena in that domain.
Figure 9.3:
Text log output showing weight-based priming
effects — the network responds with the
b
outputs (shown
in the
ev nm
column) to the
a
inputs (shown in the
Event
column), and vice-versa, as a result of prior exposure.
9.2.2
AB-AC List Learning
We have seen that a simple model of the cortex can use
slow learning to both develop new representations (over
many learning trials), and show priming-like effects af-
ter single exposures to familiar items (i.e., those items
having existing representations). However, one is often
faced with the task of having to rapidly encode novel
information on the basis of only a few learning trials
(e.g., learning someone's name, the definition of a new
word, where one's car is parked, a phone number). The
priming-like phenomenon in the cortex does not appear
to be capable of this kind of task (e.g., just look at how
long it took for the network to learn the input-output as-
sociations in the previous exploration). Thus, we have
reason to be pessimistic that the cortex will succeed at
all memory tasks.
In this section we will explore a commonly used ex-
perimental task that demands rapid learning of novel as-
sociations, and see that the basic cortical model does
not in fact succeed. This task is particularly challeng-
ing because there is a high level of overlap between
the novel associations to be learned — this overlap
is ecologically valid, however, because we are often
required to remember information about very similar
things (e.g., where one's car is parked today as opposed
to previous days). The task is commonly known as
the AB-AC
paired associates
list learning task
this)?
(c)
Now,
Test
again, and report what happens
for both the first and second time the events are pre-
sented.
(d)
Explain why this behavior occurs, and re-
late it to the priming results for humans described ear-
lier.
Go to the
PDP++Root
window. To continue on to
the next simulation, close this project first by selecting
.projects/Remove/Project_0
. Or, if you wish to
stop now, quit by selecting
Object/Quit
.
Summary and Discussion
The relatively simple model we just explored provides
a reasonable in-principle account of the mechanistic ba-
sis for long-term priming. Specifically, it shows that the
same learning mechanisms used to construct represen-
tations over long periods of exposure to an environment
(i.e., semantic memories) can also be used to explain
the effects of single exposures to particular items (Mc-
Clelland & Rumelhart, 1986). By demonstrating this in
the context of a one-to-many mapping task (e.g., stem
completion or homophone disambiguation), it was easy
(e.g.,
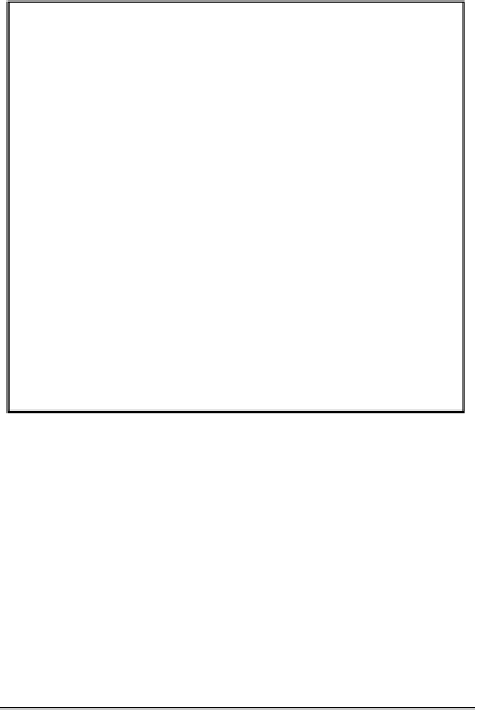

Search WWH ::

Custom Search