Geology Reference
In-Depth Information
(based on nine grid point values). This example
comes from a fairly shallow reservoir, with minimal
overburden or seismic imaging issues, so the nearest
points to the wells (coloured blue) define a reason-
able scaler relating the well points to the seismic.
Following calibration, the final function is shown in
Fig. 10.9c
incorporating both synthetic and seismic
data. Uncertainties in the prediction owing to
the variability of rock properties might be incorpor-
ated into the crossplot model by the generation of
pseudo-wells.
Mukerji and Mavko (
2008
) discuss a potential
pitfall in the use of averages for determining an attri-
bute/reservoir property relationship.
Figure 10.10
illustrates the problem. In
Fig. 10.10a
various N:G
models for a thin bed (i.e. below tuning) scenario
are generated using average values of acoustic imped-
ance for sands and shales. These scenarios are aver-
aged, using the Backus average, and normal incidence
synthetics are generated. The model suggests that
there is a linear relationship between amplitude and
N:G (
Fig. 10.10b
). However if the impedance distri-
butions of sands and shales are used (
Fig. 10.10c
),
together with Monte Carlo simulation to generate
the N:G scenarios prior to upscaling then the result
suggests a different (non-linear) relationship between
amplitude and N:G. Effectively, for this distribution
of impedances, N:G predicted from amplitude would
be less than the
a)
80
60
y = -0.8385x
R
2
= 0.3978
40
20
0
-80
-70
-60 -50 -40
Seismic Attribute
-30
-20
-10
0
b)
0
-20
-40
-60
-80
-100
-120
y = 1.275
8
x
-90
-80
-70 -60 -50 -40
Attribute (synthetic)
-30 -20 -10
0
c)
80
60
y = -0.63x
40
20
0
-120
-100
-80 -60 -40
Seismic Attribute
-20
0
model. There is
also the possibility that some of the scenarios would
give amplitudes of the opposite polarity. In addition,
considering distributions gives an inherent descrip-
tion of probability.
A number of practical insights can be drawn from
this example. Certainly one conclusion is that an
'
'
average of averages
'
Figure 10.9
Defining a relationship between a seismic attribute
and a reservoir property; (a) function estimated from well synthetics,
(b) the calibration step, estimating the scalar for matching the
seismic and synthetic data and taking into account the potential for
mis-positioning of seismic and wells (the data plotted are the nine
nearest grid points for each well, with the nearest grid point to the
well coloured blue), (c) final function with both synthetic and
seismic data displayed.
'
model is likely to be inappropri-
ate both in terms of potential bias in the prediction of
N:G but also in the nature of the attribute/rock prop-
erty relationship (i.e. linear vs nonlinear). In practice,
the linear model assumption could result in either
positive or negative bias. For example, consider a
practical situation in which wells have been drilled
on the brightest amplitudes represented in
Fig. 10.10d
and it is assumed that amplitude and N:G are linearly
related, possibly as a result of the type of modelling
shown in
Figs. 10.10a,b
. If the green, blue and red
curves in
Fig. 10.10d
represent the real geological
situation then a linear assumption based on the
average of averages
average over an area or the value at the best match
location? The answer will be case-dependent, with the
interpreter evaluating well tie issues, such as wavelet
shape and scaling uncertainties, as part of the
decision-making process. The potential uncertainty
should be appreciated and documented.
Figure 10.9
illustrates the issue of obtaining an
attribute/reservoir property relationship. A simple
linear regression fit of a seismic attribute and reser-
voir property has been defined from well synthetics
(
Fig. 10.9a
). The calibration step (
Fig. 10.9b
) shows
the synthetic attribute plotted against a range of
seismic values within ~40 m of the well
'
high
amplitude
well data would give N:G predictions for
the lower amplitudes which are too low.
'
228
location
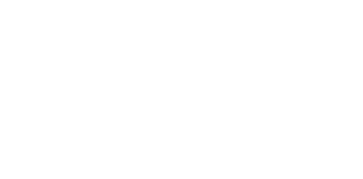






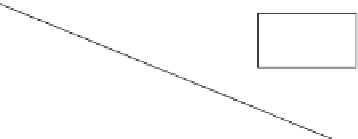



































































































































