Database Reference
In-Depth Information
The next cluster is more clearly associated with dramas and contains some foreign lan-
guage films in particular.
The last cluster
The final cluster seems to be related predominantly to action and thrillers as well as ro-
mance movies, and seems to contain a number of relatively popular movies.
As you can see, it is not always straightforward to determine exactly what each cluster
represents. However, there is some evidence here that the clustering is picking out attrib-
utes or commonalities between groups of movies, which might not be immediately obvi-
ous based only on the movie titles and genres (such as a foreign language segment, a clas-
sic movie segment, and so on). If we had more metadata available, such as directors, act-
ors, and so on, we might find out more details about the defining features of each cluster.
Tip
We leave it as an exercise for you to perform a similar investigation into the clustering of
the user factors. We have already created the input vectors in the
userVectors
vari-
able, so you can train a K-means model on these vectors. After that, in order to evaluate
the clusters, you would need to investigate the closest users for each cluster center (as we
did for movies) and see if some common characteristics can be identified from the movies
they have rated or the user metadata available.
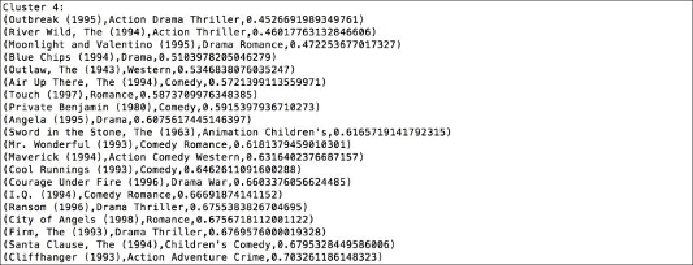