Image Processing Reference
In-Depth Information
For model-driven ST-SVR, the corresponding input vector is
xuvwt rrr rs s
=
[, ,
, ,
,
,
,
………
,
,
,
,
,
,
smt
,
()
]
t
∈ℜ
++
5
S
R
(18.14)
12
i
R
1
j
S
where
1
0
if run index
otherwise
=
i
1
if sub
ject index
otherwise
=
j
r
=
;
s
=
i
j
0
The output is still the corresponding fMRI signal intensity
y
(
u
,
v
,
w
,
t
).
After normalization, we multiply all
r
by a new coefficient
W-run
and all
s
by a new coefficient
W-subject
. These two associated coefficients will have the
effect of emphasizing between-session (run and subject, respectively) differences
when a higher value is used; lower values could pool common information over
different sessions. This would have important applications on fMRI multisession
inference, especially for analyzing and comparing control and subject group data.
With the multiresolution effect of
W-scale
addressed in Subsection 18.4.3, the
ST-SVR is expected to be able to handle and capture the possible sudden drifts
between runs and subjects. Note that the multirun and multisubject group analysis
scheme proposed here would have the advantage of accounting for between-run
and between-subject variability, and could potentially increase statistical signif-
icance in regions in which temporal variations are shared by a group of runs and
subjects even though the activations in these regions may be too low to be detected
when conducting fMRI analysis over individual runs and individual subjects.
18.4.6
T
ESTING
ON
R
EAL
F
MRI D
ATA
The ST-SVR approach is validated by using the conventional
t
-test (25) on the
SVR-restored fMRI data for activation detection, without additional presmoothing
or postprocessing. Although the conditions of the
t
-test are not satisfied here (as is
often true in fMRI analysis), we believe it provides a straightforward method of
evaluation for the technique. The Gaussian RBF kernel function (Equation 18.6) is
used in our experiments with
σ
set empirically to 0.1. Other SVR parameters are
also set empirically
C
20 (Equation 18.2 and Equation 18.4).
The proposed approach is first applied to a block-design cognitive fMRI exper-
iment performed at Yale (35), examining social attribution to geometric animations.
T2*-weighted images were acquired using a single-shot echo-planar sequence. The
pulse sequence parameters were TR
=
1200 and
ε
=
=
1500 msec, TE
=
60 msec, flip angle
=
60
°
,
3.125 mm
2
. Fourteen
coronal slices were collected and were 10 mm thick (skip 1 mm). Corresponding
T1-weighted structural images of the same thickness were collected in the same
session (TR
and NEX
=
1, providing an in-plane voxel size of 3.125
×
192 mm matrix, 2NEX). The
first four volumes of fMRI time series were discarded to discount T1 saturation
effects. We have examined this dataset for a visuospatial task from one subject and
one run. The window size used is 3
=
500, TE
=
14, FOV
=
200 mm, 256
×
160, where 160 is the total number
of time points. We did not use an isotropic window because the voxel shape is not cubic.
×
3
×
1
×
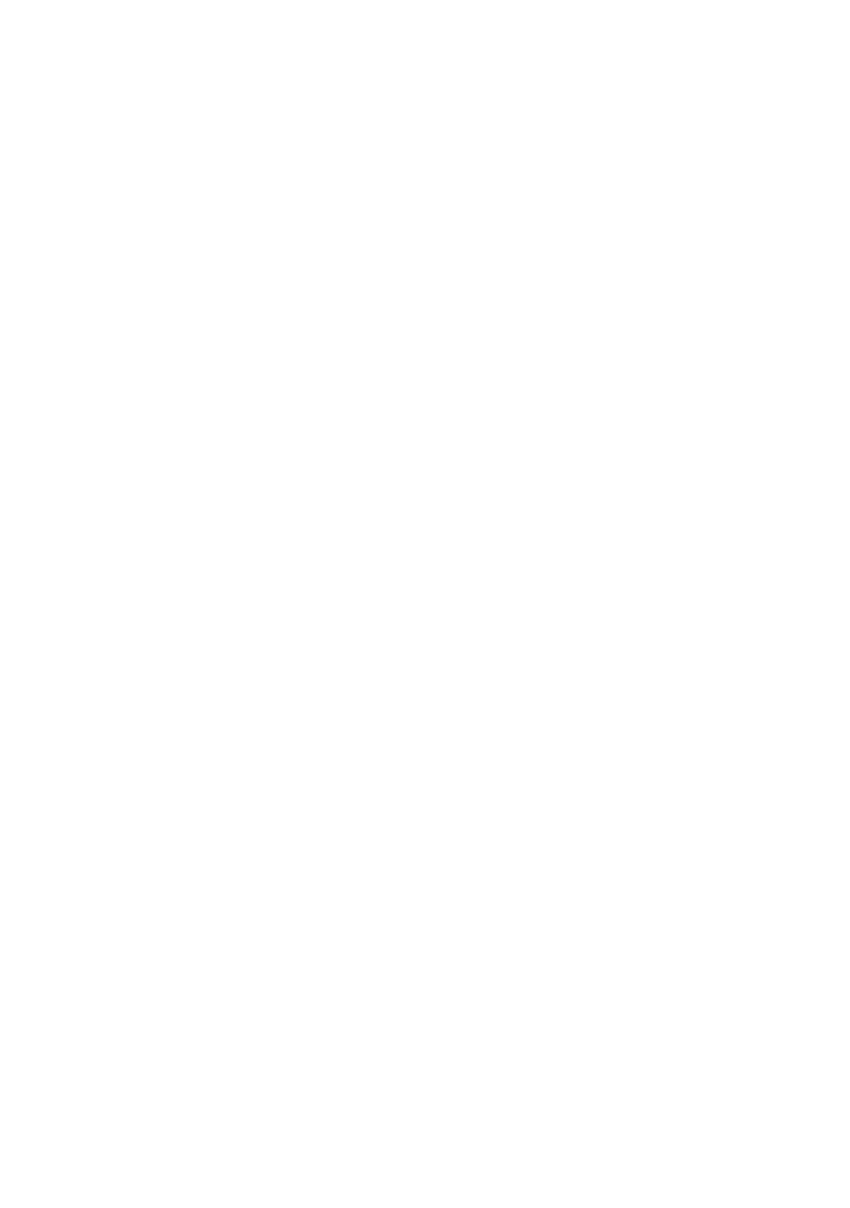





































Search WWH ::

Custom Search