Image Processing Reference
In-Depth Information
thought of as a collection of effects that one wants to test together. Such a contrast
may look like,
−
0 100
…
1000
…
which would test for the significance of the first or second parameter estimates.
The fact that the first weight is -1 as opposed to 1 has no effect on the test because
F statistics are based on sums of squares.
17.3.3
T
B
F
EMPORAL
ASIS
UNCTIONS
Functional MRI using blood oxygen level dependent (BOLD) contrast provides
an index of neuronal activity indirectly via changes in blood oxygenation levels.
For a given impulse of neuronal activity, the fMRI signal peaks some 4-6 sec
later, then after 10 sec or so drops below zero and returns to baseline after 20 to
30 sec. This response varies from subject to subject and from voxel to voxel and
this variation can be captured using temporal basis functions.
In Reference 15, the form of the hemodynamic impulse response function
(HRF) was estimated using a least squares deconvolution and a time invariant
model, where evoked neuronal responses are convolved with the HRF to give the
measured hemodynamic response (16). This simple linear framework is the cor-
nerstone for making statistical inferences about activations in fMRI with the
GLM. An impulse response function is the response to a single impulse, measured
at a series of times after the input. It characterizes the input-output behavior of
the system (i.e., voxel) and places important constraints on the sorts of inputs
that will excite a response. The HRFs, estimated in (15) resembled a Poisson or
gamma function, peaking at about 5 sec.
Knowing the forms that the HRF can take is important for several reasons,
not the least of which is because it allows for better statistical models of the
data. The HRF may vary from voxel to voxel, and this has to be accommodated
in the GLM. To allow for different HRFs in different brain regions, the notion
of temporal basis functions to model evoked responses in fMRI was introduced
(17) and applied to event-related responses in (18,19). The basic idea behind
temporal basis functions is that the hemodynamic response induced by any
given trial type can be expressed as the linear combination of several (basis)
functions of peristimulus time. The convolution model for fMRI responses takes
a stimulus function encoding the supposed neuronal responses and convolves
it with an HRF to give a regressor that enters into the design matrix. When
using basis functions, the stimulus function is convolved with all the basis
functions to give a series of regressors. The associated parameter estimates are
the coefficients or weights that determine the mixture of basis functions that
best models the HRF for the trial type and voxel in question. We find the
most useful basis set to be a canonical HRF and its derivatives with respect
to the key parameters that determine its form (e.g., latency and dispersion).
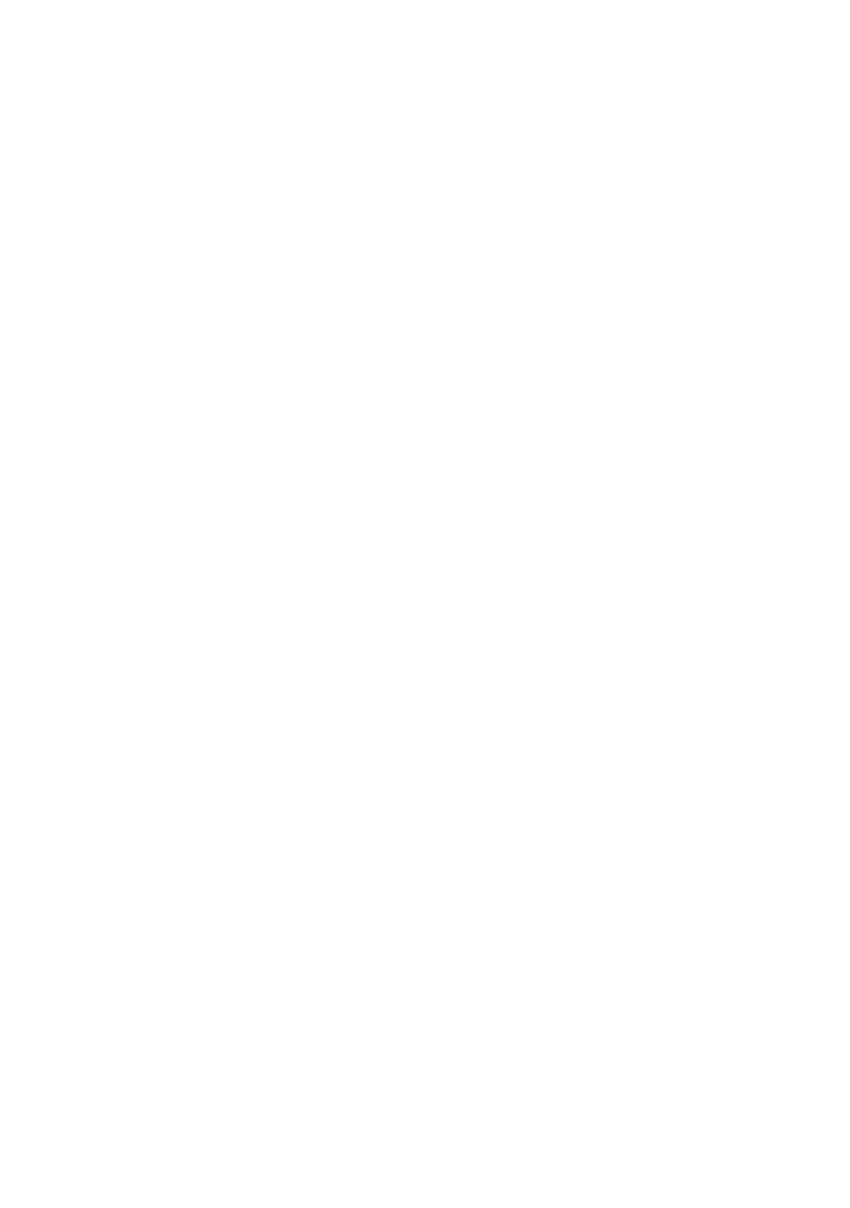





































Search WWH ::

Custom Search