Image Processing Reference
In-Depth Information
16.5.6
S
IMULATION
AND
A
LGORITHM
C
OMPARISON
The analysis of fMRI data by means of independent components is a complex
process, and the final result may depend not only on the processing strategies but
also on the algorithm that has been chosen. Because the real sources are unknown,
it is important to perform some tests on simulated data sets: these may be simple
data sets such as time series with varying degrees of Gaussian noise and with
superimposed simulated activations. The noise can be extracted from real data sets,
as in Reference 44, and may be white or correlated. It is then possible to use images
such as phantoms to simulate the spatial properties of the activation maps such as
the spatial correlation of the sources due to the vascular point-spread function. A
model with a simplified distribution of baseline voxel values was used, with artifi-
cially added movement in Reference 45 to test the behavior of spatial ICA. In order
to have realistic noise models, it is possible to use resting-state data (null data) and
superimpose the activations using self-generated masks or masks obtained from real
activation thresholded maps. These maps can be used as masks in order to modulate
the null data set with simulated activation. In Reference 44 the InfoMax and the
fastICA algorithms were compared along with some preprocessing strategies. The
Kullback—Leibler divergence between the estimated and real source distributions
was found. The results reported in this article show some preference for the PCA
reduction stage at higher noise levels in a completely simulated data set, and always
with hybrid data sets obtained by adding simulated sources to a real fMRI data set.
In this work, it was found that InfoMax seems to outperform the fastICA algorithm
because it does not constrain the sources to be orthogonal to each other. In Reference
46 receiver operating characteristics (ROC) are used to test for the detection of spatial
accuracy of the InfoMax and fastICA algorithms. A likelihood analysis was used to
test the reliability of the model. The filtering effect of each algorithm was tested
using a Gaussian mixture model to fit each map because from this fit it is possible
to estimate the variance or the residual noise. The spatial structure of the maps was
estimated by means of a cluster-sizing function. The test was performed on a sim-
ulated data set, obtained by adding the activated regions to resting-state data, and
on real data. In the latter case, the results of a linear correlation test were used as a
benchmark for the ICA analysis. The results from the simulated data set were aligned
with those from real data sets and it was confirmed that although fastICA exhibited
better overlap with linear correlation results, the likelihood analysis and noise results
showed the better performance of InfoMax, confirming its superiority in global
model estimation and filtering capabilities. This was thought to be due to the adaptive
nature of the InfoMax algorithm.
REFERENCES
1.
Ogawa, S., Menon, D.W., Ellermann, J.M., Kim, S.G., Merkle, H., and Ugurbil,
K. (1992). Intrinsic signal changes accompanying sensory stimulation: functional
brain mapping with magnetic resonance imaging.
Proc. Natl. Acad. Sci.
89:
5951-5955.
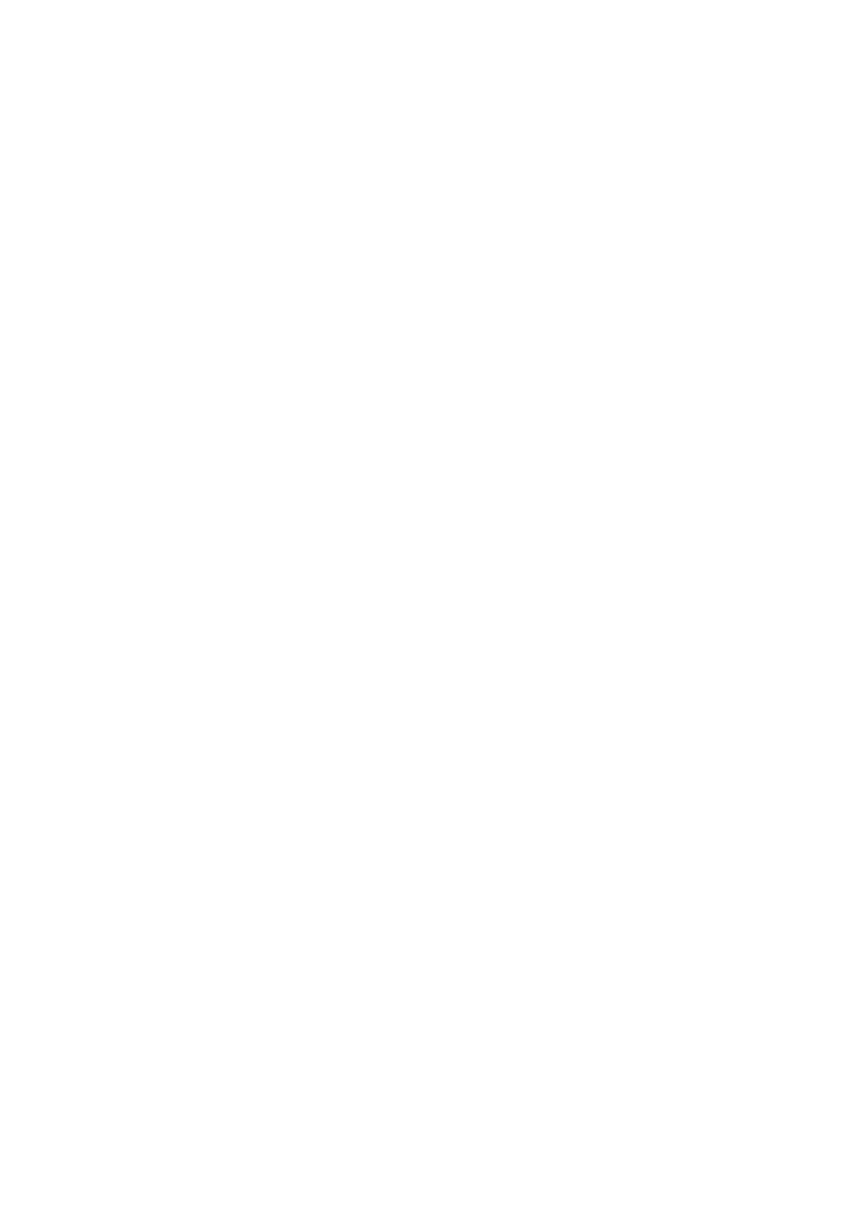





































Search WWH ::

Custom Search