Image Processing Reference
In-Depth Information
accounts for the temporal covariance in the data set, i.e., covariances among the
time points. The associated eigenvectors
v
i
span the row space of the data. It is
important to note that if we want to study the covariances among the time points,
the mean value has to be subtracted from each image; otherwise, we study the
correlation matrix. The two approaches, the study of the spatial covariance matrix
or temporal covariance matrix, are called, respectively,
temporal
and
spatial prin-
cipal-component analysis
[30]. Depending on which features we are more interested
in, we can choose between the two approaches. In the case of fMRI data analysis,
these were not proved to exhibit great difference. In Reference 31 these approaches
are referred to as R and Q analysis, respectively, whereas subtracting the mean both
from time series and images is referred to as M analysis. It can often be found that
instead of normalization by the number of observations, the matrices
XX
T
or
X
T
X
are used. If the mean from each row (time series) is subtracted, then we have the
matrix
XX
T
proportional to the sample covariance matrix for the voxels
ˆ
C
∝
X
T
(16.21)
V
If the mean from each column (images) is subtracted, the matrix
X
T
X
is
proportional to the sample covariance matrix for the time points
ˆ
CXX
t
∝
T
(16.22)
The results in terms of eigenvectors are the same, whereas the eigenvalues
differ by a scaling term. PCA can be achieved by means of singular value
decomposition (SVD)[63] of the data matrix
XUSV
=
T
(16.23)
where
U
is an orthogonal matrix of
n
×
r
dimension whose columns are the
s
i
12
,
vectors
u
i
,
S
is an
r
r
diagonal matrix with elements and
V
is an orthogonal
matrix whose columns are the vectors
v
i
. Reorganizing Equation 16.23 it is
possible to see that
×
XX
=
US U
(16.24)
T
2
T
confirming that the columns
u
i
are eigenvectors of
XX
T
and
XX VSV
T
=
2
T
(16.25)
so that the columns of
V
are eigenvectors of the matrix
X
T
X
. Equation 16.23 can
be written as
r
∑
X
=
s
i
u v
T
(16.26)
i
i
ki
=


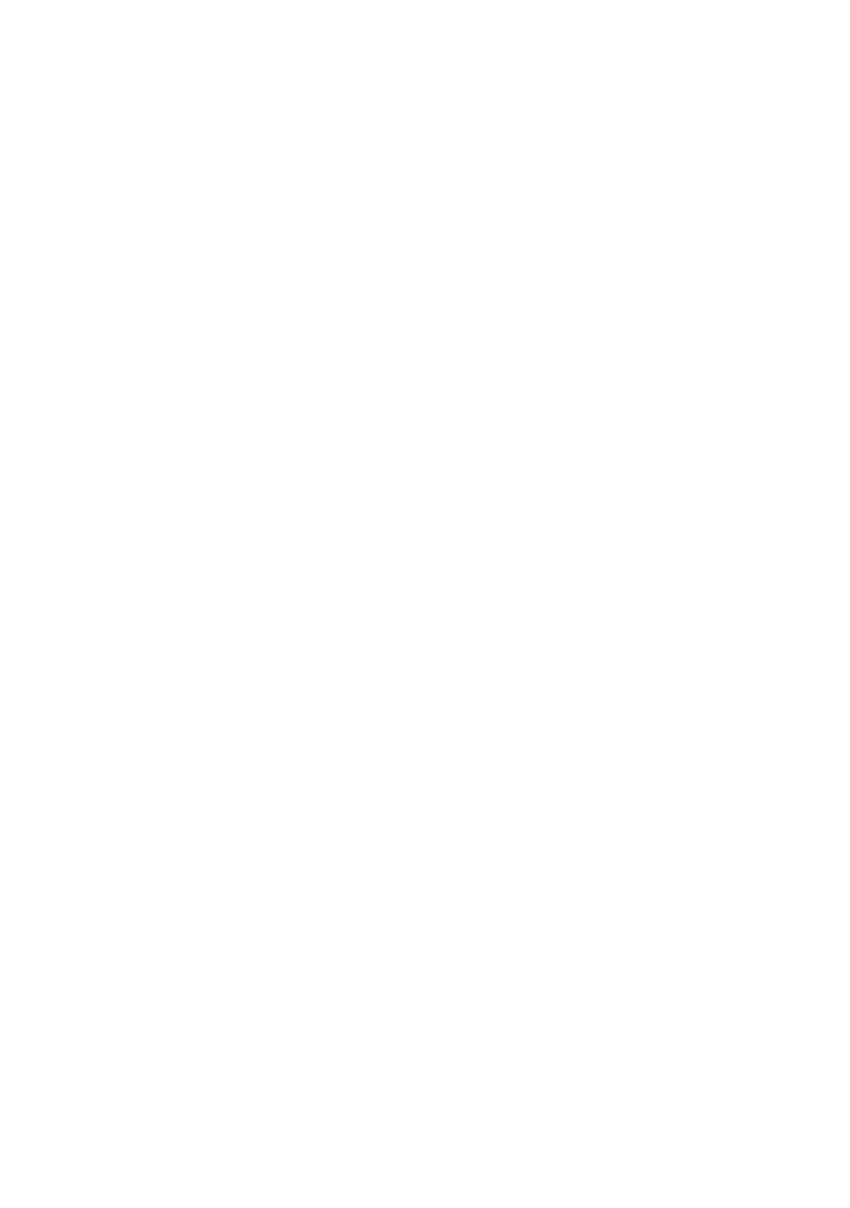





































Search WWH ::

Custom Search