Image Processing Reference
In-Depth Information
FIGURE 16.1
Schematic representation of the data matrix. Each image is vectorized and
assigned to a column of the data matrix X.
(see Figure 16.1). In data clustering, each voxel time series is seen as an individual
element to be classified, so these methods can be seen as
individual-directed
methods
. The
i
th voxel's time course can be written as
x
(
t
) with
t
=
1, 2,
…
,
p
i
and can be seen as a
p
-dimensional vector belonging to
R
p
,
x
=
x
(1),
i
i
x
(2),
…
,
x
(
p
)} [5]. In
Figure 16.2
the procedure is shown for a time course with
i
i
three observations. The entire data set, consisting of
n
time series corresponding
to
-dimensional space.
In other multivariate analysis methods, such as PCA or ICA, each voxel time
series can be seen as a set of time-domain observations of a variable. These
methods are called
n
brain voxels, can be seen as a collection of
n
vectors in
p
because they try to find the rela-
tionships among variables. These approaches can be applied to fMRI data both
in the temporal and spatial domains: in the temporal domain the variables are the
voxels time series as described earlier, and in the spatial domain the variables
are the time points and the observations are the voxels values at each time point.
Within this framework the time series extracted from the
variable-directed methods
i
th brain voxel can be
seen as a variable
x
with
p
time observations: the entire data set can be seen as
n
i
observed variables that can be written as a random
n
-dimensional vector
x
=
{
x
,
1
x
, …,
x
}. The dual approach considers the time points as variables and the rows
2
n
, the voxels values, as observations. Common preprocessing strategies involve
the operation of slice timing correction and registration of images in order to
of
X
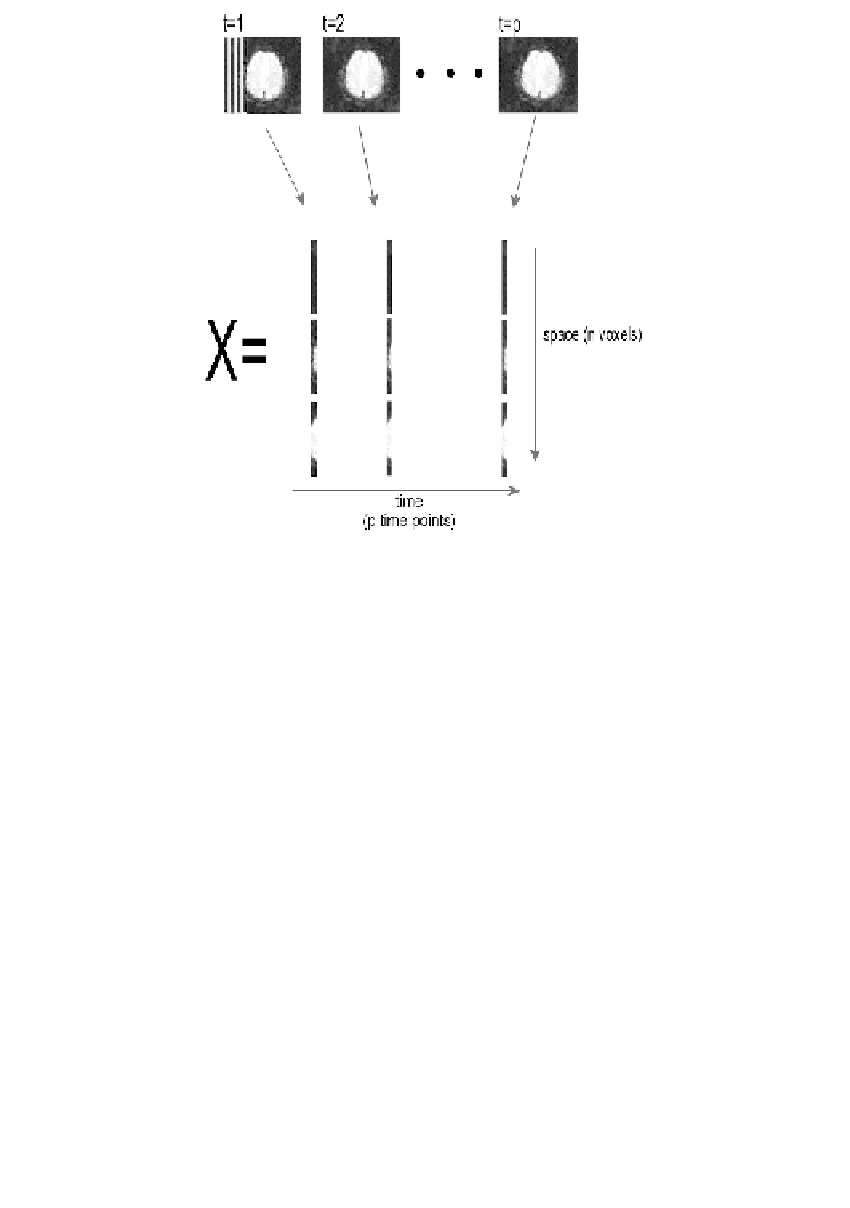





































Search WWH ::

Custom Search