Image Processing Reference
In-Depth Information
vectors c
c
L
] and computing contrast (t) maps. It can be shown that for
any contrast c the ratio
=
[c
1
c
2
…
c
cXX c
T
β
T
′
t
=
(15.24)
ˆ
σ
(
)
2
−
1
L degrees of freedom [24].
Thus, a map that highlights brain locations in which there is a significantly
greater activity in the first condition as compared to the second can be easily
obtained in the following way:
follows a Student's
t
distribution with T
−
1.
Define a contrast vector c
=
[1
−
1 0 0
…
0].
2.
Calculate the statistic
t
of Equation 15.24 for each voxel.
3.
Color-code the voxels with a value
t
t
0
, with
t
0
being the value that
corresponds to a prefixed significance value
p
0
.
>
15.4
SELECTION OF SIGNIFICANCE THRESHOLDS
IN FMRI STATISTICAL MAPS
A common problem of the methods based on the voxel-by-voxel statistical anal-
ysis is in the selection of a correct threshold value for segment activated and
nonactivated voxels. This value may be chosen with reference to the “uncorrected”
single pixel significance derived by comparing the statistical values obtained at
each voxel with the values of an assumed [18] or empirically derived [32] null-
hypothesis distribution. The optimum selection of this threshold, however, is not
straightforward. High (conservative) threshold values lower the probability of
incorrect detection of activation (false positives), but they also increase the prob-
ability of failing to detect “true-but-noisy” activated regions (false negatives).
Low threshold values, on the other hand, result in maps in which regions unrelated
to the task may appear as activated. Further, the uncorrected value of significance
is the result of statistical tests performed separately for a large number of time
courses. Thus, this value has to be “corrected” for multiple comparisons. Given
the very large amount of sampled voxels, the simple statistical approach of
preventing false positives by adjusting the significance level
p
using Bonferroni
correction (
p
'
p/
N
) is too conservative and leads to a substantial loss of
statistical power [33].
One of the causes for such loss of power is that in the application of the
Bonferroni correction, it is assumed that each voxel is an independent comparison,
whereas voxels may be spatially correlated and, thus, the number of effectively
independent comparisons is less than the total number of voxels in the data set.
Another cause is the failure of this approach to exploit the spatial extent of
=
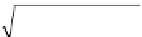
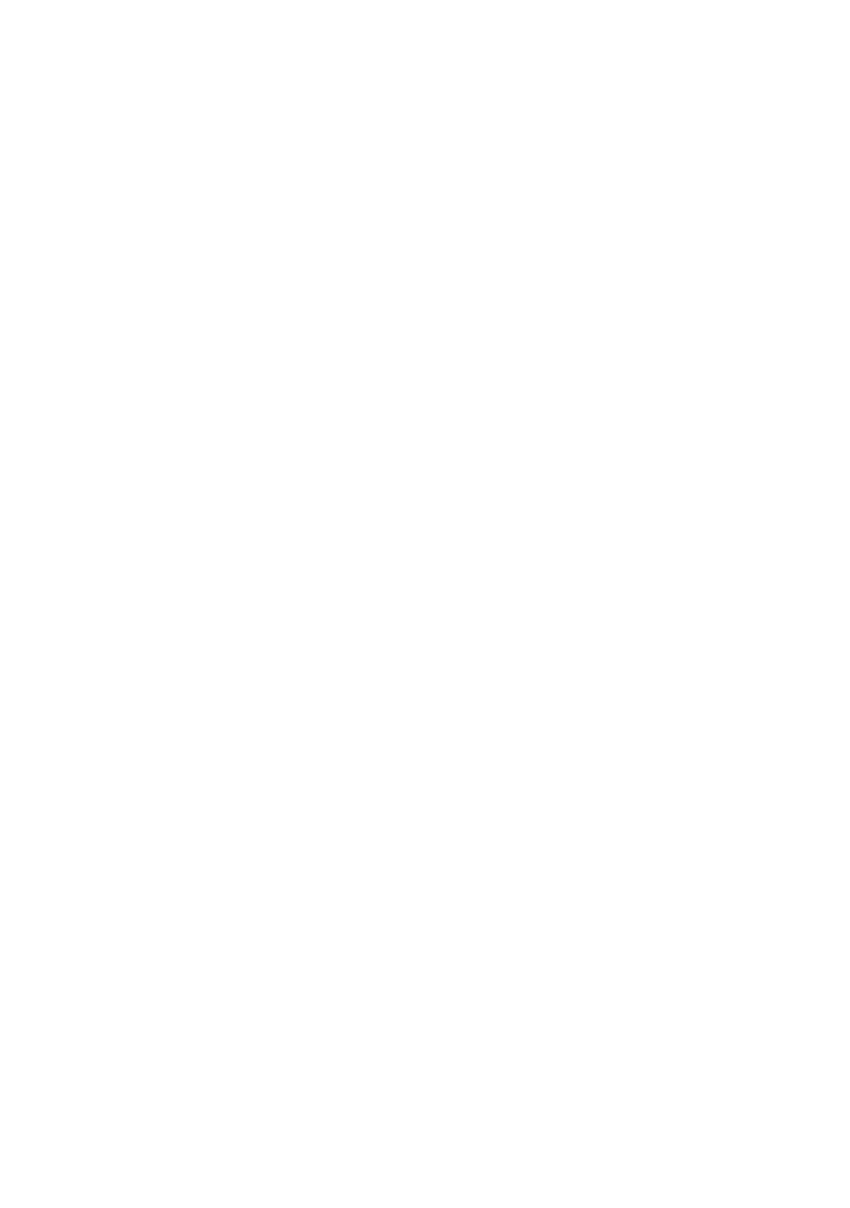





































Search WWH ::

Custom Search