Image Processing Reference
In-Depth Information
The results on simulated and experimental data from a somatosensory MEG/fMRI
experiment confirmed the applicability of Bayesian formalism to the multimodal
imaging even under the set of simplifying assumptions mentioned above.
Usually, model
M
is not explicitly mentioned in Bayesian formulations (such
as Equation 8.20) because only a single model is considered. For instance, Baye-
sian formulation of LORETA E/MEG inverse corresponds to a DECD model,
where is constrained to be smooth (in space), and to cover a whole cortex
surface. In the case of Bayesian model averaging (BMA), the analysis is carried
out for different models
M
i
, which might have different nuisance parameters, e.g.,
E/MEG and BOLD signals forward models, possible spatial locations of the
activations, constraints to regularize E/MEG inverse solutions. In BMA analysis,
we combine results obtained using all considered models to compute the posterior
distribution of the quantity of interest
Θ=
Q
pM
pD
(
∆|
)
=
pDMpMD
(
∆| ,
)
(
|
)
,
(8.21)
i
i
i
where the posterior probability
(
|
pM
()
p
()
D
)
of any given model
M
i
is computed via
i
Bayes' rule using prior probabilities
,
and the likelihood of the data
given each model
∫
pD M
(
|
)
=
pD
(
|
ΘΘΘ
,
M p
)
(
|
M d
)
.
(8.22)
i
i
i
Initially, BMA was introduced into E/MEG imaging [151], in which Baye-
sian interpretation of Equation 8.8 was formulated to obtain for the
case of Gaussian uncorrelated noise ( ). In order to create a model,
we partition the brain volume into a limited set of spatially distinct functional
compartments, which are arbitrarily combined to define a
M
i
search space for the
E/MEG inverse problem.
At the end, different models are sampled from the posterior probability
to get the estimate of the expected activity distribution of ECDs over
all considered source models
p
(
QXB
|
,
)
WC
==
ε
ν
I
X
ε
pM
i
(
|
X
)
∑
E
[
QX
|
]
=
E
[
QX
|
,
M
]
p M
(
|
X
)
i
i
i
∑
Var[
QX
|
]
=
Var[
QX
|
MpM
i
](
X
)
i
i
where the normalized probability
pM
i
(
|
X
)
, Bayes' factor
B
i
0
, and prior odds
α
i
,
are
α
B
pM
pM
(
X
X
|
|
)
M
pM
p
(
i
)
ii
0
i
pM
(
|
X
)
=
B
=
α
=
∑
i
i
0
i
(
)
(
)
α
B
0
0
kk
0
k

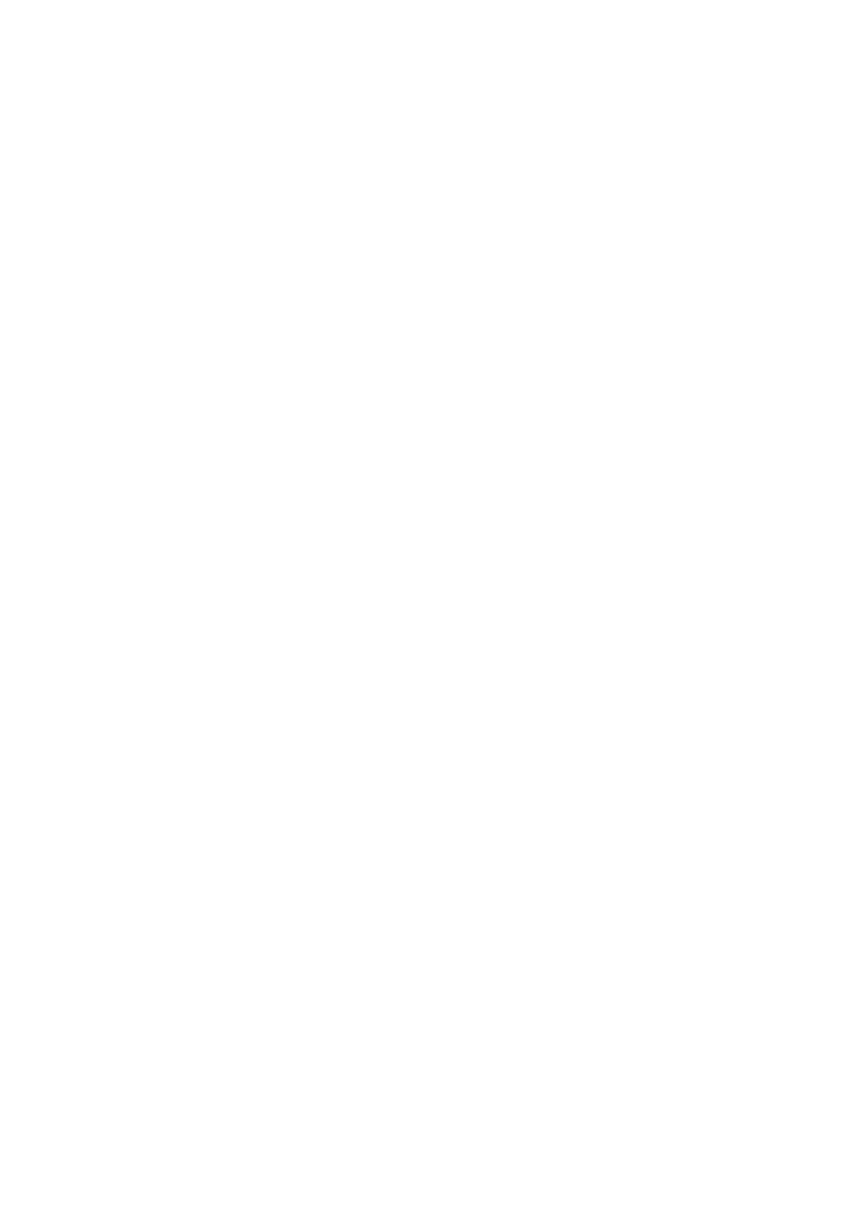





































Search WWH ::

Custom Search