Image Processing Reference
In-Depth Information
models, which restricts their applicability to the specific image processing scenarios
they were designed for. For instance, the number of tissue types present in the images
must be specified in advance, and appropriate intensity models have to be provided
for each of the tissues considered. Histogram-based techniques, on the other hand,
do not depend on such models, and can therefore be more generally applied, for
instance, to correct images of different anatomical areas or images of pathological
cases that might otherwise violate the model assumptions. It should be noted, how-
ever, that histogram-based techniques typically require the removal of undesired
structures, such as air and nonbrain tissues in MR images of the head, prior to the
inhomogeneity estimation. As the employed tissue removal steps are often tailored
to specific body regions and acquisition protocols, applying histogram-based meth-
ods to different types of images does require some adaptation in practice. Further-
more, it remains to be seen how robust histogram-based techniques are with respect
to intensity variations due to pathology such as brain lesions, whose intensities vary
across the range of nonpathological tissue intensities. Whereas combined segmen-
tation and inhomogeneity estimation methods can explicitly take such pathological
tissues into account, either by incorporating them in their model or by detecting
them as model outliers [24], histogram-based approaches treat all voxels alike, which
may lead to erroneous inhomogeneity field estimations in such cases.
As a final remark, the methods presented in this chapter can be mainly applied
to structural MR imaging and MR angiography. Images acquired with the more
recently introduced diffusion tensor imaging (DTI) sequences remain currently
uncorrected, and no methods have been proposed thus far.
ACKNOWLEDGMENTS
Both authors Koen Van Leemput and Martin Styner contributed equally to this
chapter. The authors would like to thank many colleagues and friends in the medical
image processing community for the great discussions and collaborations in regard
to intensity inhomogeneity correction. Especially they would like to mention Guido
Gerig, Christian Brechbühler, Gábor Székely, Mike Brady, Frederik Maes, Dirk
Vandermeulen, Alan Colchester, and Nicholas Ayache.
REFERENCES
1.
Jungke, M., von Seelen, W., Bielke, G., Meindl, S. et al. (1987). A system for the
diagnostic use of tissue characterizing parameters in NMR-tomography. in
Proceed-
ings of Information Processing in Medical Imaging,
IPMI'87, Vol. 39, pp. 471-481.
2.
Vannier, M.W., Speidel, Ch.M., Rickman, D.L., Schertz, L.D. et al. (November
1988). Validation of magnetic resonance imaging (MRI) multispectral tissue clas-
sification. in
Proceedings of 9th International Conference on Pattern Recognition,
ICPR'88, pp. 1182-1186.
3.
Merickel, M.B., Carman, C.S., Watterson, W.K., Brookeman, J.R., and Ayers, C.R.
(November 1988). Multispectral pattern recognition of MR imagery for the non-
invasive analysis of atherosclerosis. in
Proceedings of 9th International Confer-
ence on Pattern Recognition,
ICPR'88. pp. 1192-1197.
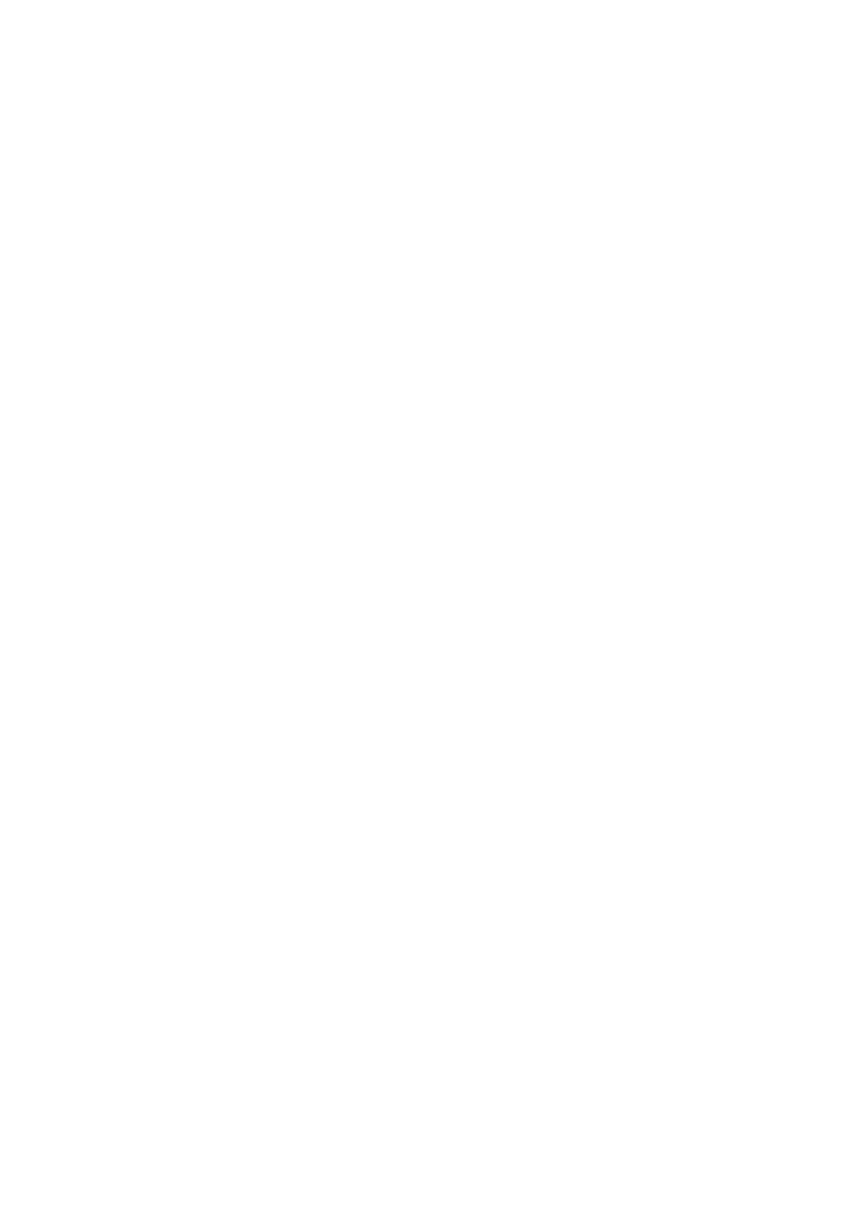





































Search WWH ::

Custom Search