Image Processing Reference
In-Depth Information
Raw MR data, directly obtained from an MR scanner, represent the FT of a
magnetization distribution of a volume at a certain point in time. Such data are
generally complex valued and corrupted by zero-mean Gaussian-distributed noise
[29]. An inverse FT, which yields the complex magnetization distribution of the
object under study, does not change the type of data PDF because of the linearity
and orthogonality of the FT. Hence, these data are corrupted by zero-mean Gaus-
sian-distributed noise as well. The complex data, however, are generally not
retained but transformed into magnitude and phase data. This is because the
amplitudes and phase values of the magnetizations are of greater interest than the
real and imaginary components of the complex data values. Transformation to a
magnitude or phase MR image, however, is nonlinear. As a consequence of this
transformation, the PDF of the data changes. As discussed in the preceding text,
magnitude data are then governed by a Rician PDF.
Nevertheless, conventional estimation techniques usually assume the data to be
Gaussian distributed. Whenever other PDFs come into play (such as a Rician dis-
tribution), one still tends to use parameter estimation techniques that are based on
Gaussian-distributed data [30-32]. The justification for this is that when the SNR is
high, the actual data PDF is very similar to a Gaussian PDF (cf.
Subsection 4.2.1
).
In addition, Gaussian PDFs have attractive computational properties.
However, the Rician PDF deviates significantly from a Gaussian PDF when
the SNR is low, leading to significantly biased results. To reduce this bias,
parameter estimation methods were proposed that exploit the properties of the
Rician PDF [24,33-35]. Although these estimators reduce the bias, they are not
optimal. In this section, it is shown where the bias appears in the conventional
estimation. In addition, the ML estimator for Rician-distributed data is constructed
and its performance is compared that of conventional estimators [36].
Furthermore, consider a data-processing application (e.g., noise filtering) that
requires estimation of the underlying signal amplitude from a number of noise-
corrupted, complex-valued data points. Thereby, for each complex data point
belonging to the data set, the underlying signal amplitude is assumed to be the same.
This amplitude can be estimated either by first transforming the complex data points
into a set of magnitude data points and then estimating the signal amplitude from
the so-obtained data set, or by directly estimating the signal amplitude from the
original complex-valued data points. Indeed, both data sets contain the signal ampli-
tude to be estimated.
In general, if
N
complex points are available, the data set consists of 2
N
observations (
N
real and
N
imaginary data points) and
N
2 unknowns (
N
true
phase values, 1 true signal amplitude, and the noise variance). On the other hand,
if the
N
complex data points are transformed into a set of
N
magnitude data
points, such a data set has only two unknown parameters (the true signal amplitude
and the noise variance). Hence, questions such as “Should we use the complex
data set or the magnitude data set when estimating the unknown signal ampli-
tude?” and “Does it matter whether or not the true phase values of the complex
data points, from which the signal amplitude is estimated, are the same?” may
arise. In this section, these questions are addressed. In order to simplify the
+
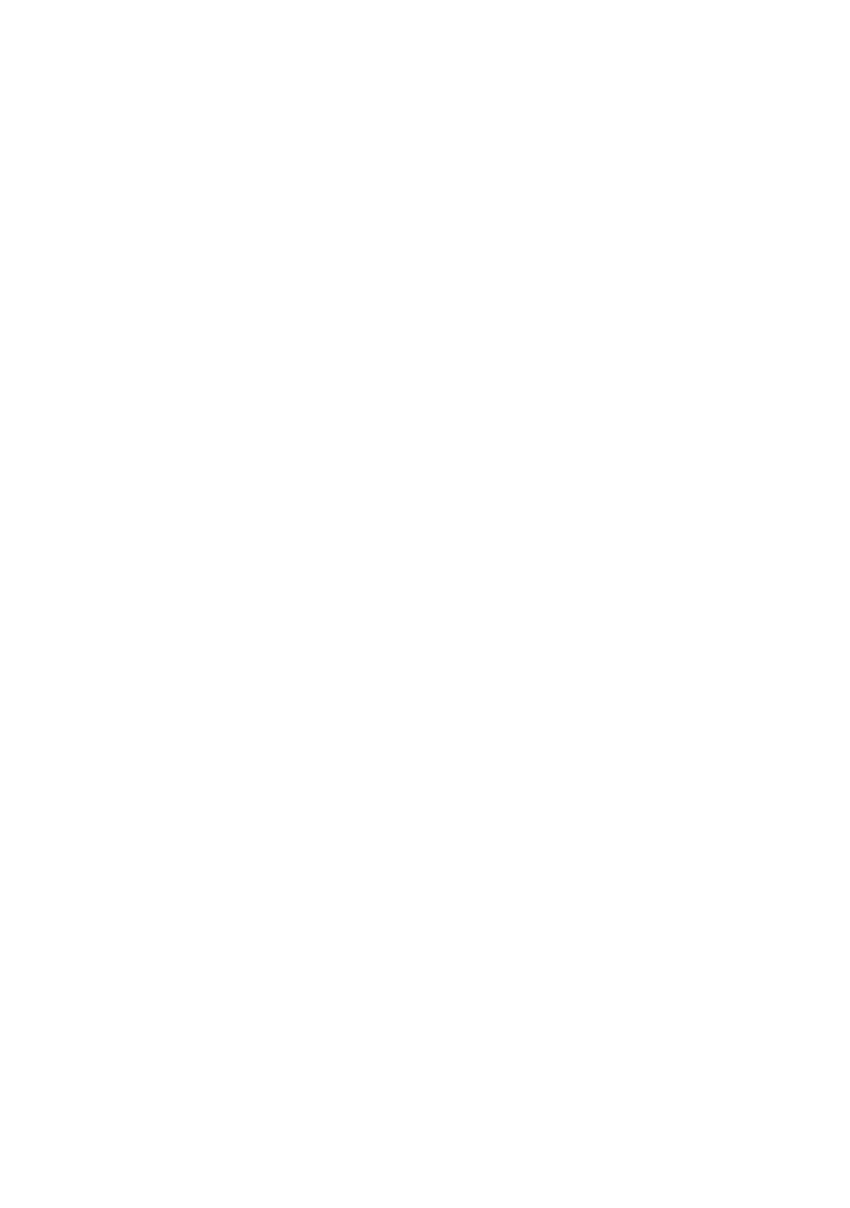





































Search WWH ::

Custom Search