Information Technology Reference
In-Depth Information
1.0
0.8
0.6
0.4
V
0.2
0.0
0.2
0
2000
4000
6000
8000
10 000
I
Fig. 1.
Illustration of upper and lower bounds on the result of cross-validation with respect to
the size of sample
I
. Other constants are:
η
=
0
.
01
⇒
−
α
(
η
)
≈
0
.
93,
N
=
100,
n
=
3. With
1
probability 1
−
α
(
η
)
or greater, the result
C
of cross-validation falls between the bounds.
Proof (
Proof of Theorem 2
).
We remind:
I
=
n
−
n
I
,
I
=
n
I
.
With probability at least 1
−
η
, the following bound on true risk holds true:
ω
I
)+
ln
N
−
ln
η
R
emp
(
R
(
ω
I
)
≤
.
(19)
2
I
For the selected function
ω
I
, fixed from now on, Chernoff inequality is satisfied on the
testing set (empirical testing risk) in either of its one-side-versions:
−
η
2
I
ln
R
emp
(
ω
I
)
−
R
(
ω
I
)
≤
,
(20)
−
η
2
I
ln
R
emp
(
ω
I
)
≤
R
(
ω
I
)
−
,
(21)
with probability at least 1
−
η
each. By joining (19) and (20) we obtain, with probability
at least
10
1
−
2
η
the system of inequalities:
−
η
2
I
≤
ln
R
emp
(
R
emp
(
ω
I
)
−
R
(
ω
I
)
≤
ω
I
)
+
ln
N
−
ln
η
.
(22)
2
I
10
The minimum probability must be 1
−
2η rather than
(
1
−
η
)
2
(probabilistic independence
case) due to correlations between inequalities. It can be also viewed as the consequence of
Bernoulli's inequality.

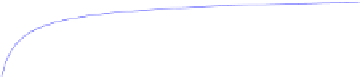

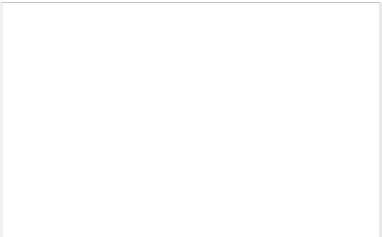





















































