Information Technology Reference
In-Depth Information
1
0.9
0.8
0.7
Πο = 0.3
Πο = 0.4
Πο = 0.5
Πο = 0.6
Πο = 0.7
0
Bif
1
0.6
0.5
0.4
0.3
0.2
0.1
0
0
0.1
0.2
0.3
0.4
0.5
0.6
0.7
0.8
0.9
1
pa1
Fig. 6.
Dynamics that emerge for coefficients of concepts, according to values set to
p(a1)
and
p(a2)
(bifurcation, 1 = convergence to 1, 0 = convergence to 0) and lines for different values of
Π
0
according to the Markov chain model
must meet
p(a1)
and
p(a2)
for obtaining different values of
Π
0
. Figure 6 shows the
same graph presented in Figure 4, but displaying lines for
0
= 0.3 to 0.7 according to
the Markov chain model that corresponds to expression (12).
From Figure 6, we can see that the line for
Π
Π
0
= 0.5 (the ideal condition for getting
a bifurcation) approximately coincides with a line equal to the one we calculated for
the simple probabilistic model (see expression (3) and Figure 4). The other lines for
Π
0
= 0.6 and 0.7 are located in the region where the ABM exhibits the convergence to
one dynamic and the lines for
Π
0
= 0.3 and 0.4 lie in the region where the
convergence to zero dynamic emerges. Thus, we can see that the Markov chain model
represents fairly well the conditions under which the ABM exhibits the three different
dynamics. Moreover, note that the lines tend to converge toward the lower right
corner of the graph, where
p(a1)
>>
p(a2)
and tend to diverge toward the upper left
corner, where
p(a1)
<<
p(a2)
. This means, that the region where we get the
bifurcation and which separates the areas where we obtain the convergence to one and
zero, gets narrower when
p(a1)
>>
p(a2)
and wider when
p(a1)
<<
p(a2)
. That
characteristic was the one that the probabilistic model described in 5.1 was not able to
capture.
5.3
Sensitivity of Models to Changes in Values of Some Parameters
By analyzing the ABM's and its associated probabilistic models' sensitivity to
different parameters, we are able to derive predictions for “real world” situations.
Although the Markov chain model presented in 5.2 better explains the dynamical
properties of the ABM than the probabilistic model described in 5.1, the latter model
is easier to analyze from a substantive point of view. Thus, based on expression (1),
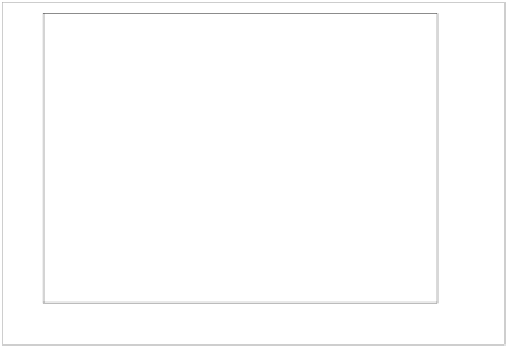
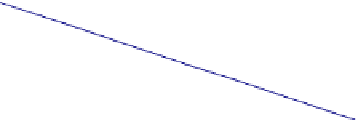



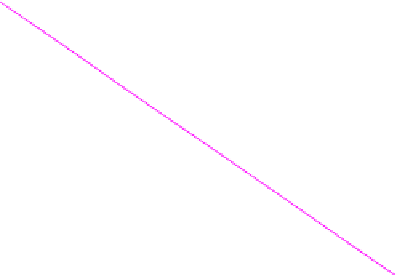

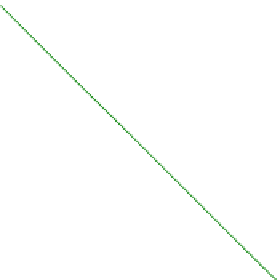

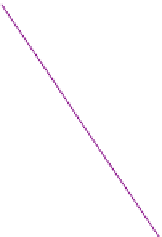
























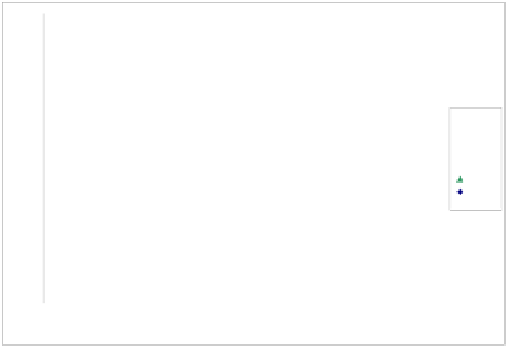
















































