Environmental Engineering Reference
In-Depth Information
FIGURE 9.12
Output generated by the rule set 4 (PCA 2
feature value less than 22 000 at scale level 1). Note: A few
pixels mistakenly identified as pool.
FIGURE 9.13
Output map produced by the nearest neigh-
bor classifier using mean value of all the original bands and
the first three PCA bands. Note: soil
=
yellow; building
=
white; impervious
=
gray; trees
=
dark green; pool
=
cyan,
and, buildings appeared to be brighter than other land-cover
objects in the brightness band.
Substantial confusion in spectral signatures between unman-
aged soil and some impervious surface types was also evident in
the image subset. For example, unmanaged soil in a false color
composite display (near infrared, red visible, and green visible
bands in red, green, and blue) appears as light brown or dark
colors, as do some rooftop materials and roads. After examining
different levels of object scales, we felt that an object scale level of
1 (scale parameter 10) was appropriate for this dataset. Hence,
we decided to use scale level 1 for the nearest neighbor classifier.
The object-oriented approach allows additional selection or
modification of new objects (training samples) each time after
performing a nearest neighbor classification quickly until the
satisfactory result is obtained (Myint,
et al
., 2008). Hence, we
attempted to use many different training samples (objects) per
class as a trial and error approach. Figures 9.13 and 9.14 present
output maps showing swimming pool and other urban land-
cover classes generated by the nearest neighbor classifier with the
above approach with the above two sets of features. There were 29
soil object samples, 15 building samples, 28 impervious samples,
six tree samples, two pool samples, nine shadow samples, and
two grass samples used to identify the selected classes using mean
values of the original bands and PCA bands 1, 2, and 3. Hence,
there were a total of 91 training samples for the first combination
of features. We used 15 soil object samples, 16 building samples,
17 impervious samples, 14 tree samples, two pool samples, six
shadow samples, and two grass samples to identify the same
classes using mean values of the original bands, brightness, and
maximum difference (Max. Diff.). There were 72 total training
samples for this combination.
shadows
=
black; grass
=
light green.
FIGURE 9.14
Output map produced by the nearest neigh-
bor classifier using mean value of all the original bands,
Brightness band, and max. Dif. Band. Note: soil = yellow;
building = white; impervious = gray; trees = dark green;
pool = cyan, shadows = black; grass = light green.
and 99.65% respectively (Tables 9.3, 9.4, 9.5, 9.6). However, we
should not be satisfied with these overall accuracies since only
1973 pixels belong to the pool area and the entire image contains
175 062 pixels. Hence, the overall accuracy in this case does not
reflect appropriately the effectiveness of a particular rule set in
identifying the swimming pool.
Producer's accuracies produced by the rule sets 1, 2, 3, and
4 are 82.82, 88.60, 95.39, and 94.48% respectively. While rule
set 3 yielded the highest producer's accuracy, it had a slightly
lower user's accuracy (80.77%). This implies that even though
95.93% of the pool area has been correctly identified as pool,
only 80.77% of the area identified as pool in the output map is
truly of that category. The highest user's accuracy resulted from
rule set 2 (95.26%). The same rule s
et al
so produced relatively
high producer's accuracy (88.60%). In fact this was the rule set
that yielded the highest overall accuracy. However, we cannot
make a conclusive decision on which one of the two rule sets is
the best to extract the pool. In practice, one could apply both
9.5
Results and discussion
9.5.1
Decision rule set to extract pool
Classification accuracies are not significantly different among
the four outputs generated by different decision rules or object
scale levels. The overall accuracies achieved by the rule sets
1, 2, 3, and 4 for the pool detection are 99.59, 99.82, 99.69,










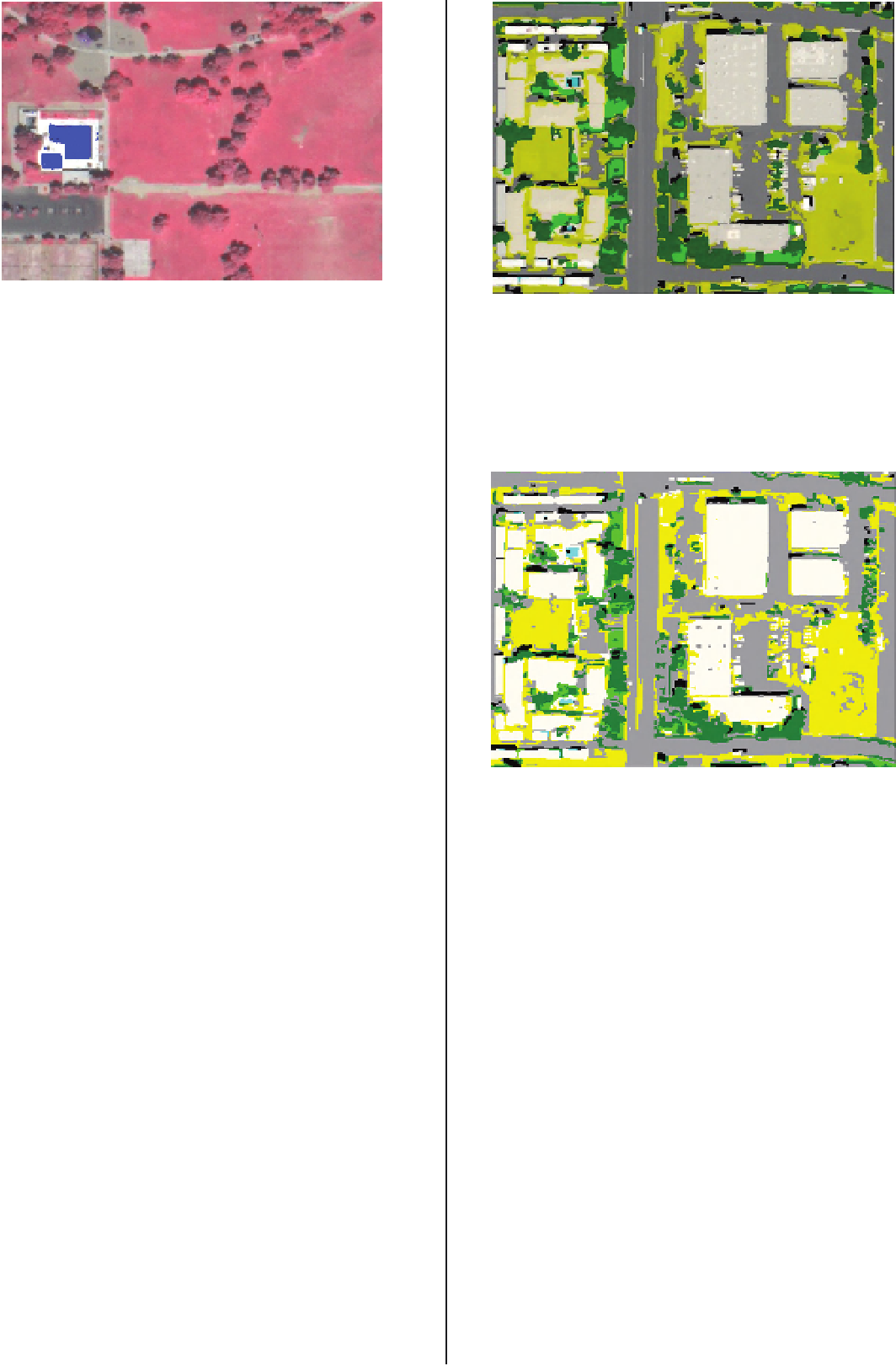
Search WWH ::

Custom Search