Information Technology Reference
In-Depth Information
80
IDCV−EVD
IDCV−GSO
70
0.8
60
50
0.78
40
0.76
30
DCV
IDCV−EVD
20
0.74
10
0
16
20
24
28
32
36
40
44
49
16
20
24
28
32
36
40
44
49
Training samples
Training samples
(a)
(b)
80
IDCV−EVD
IDCV−GSO
70
1
60
50
0.98
40
0.96
30
20
DCV
IDCV−EVD
0.94
10
0
6
8
10
12
14
16
18
6
8
10
12
14
16
18
Training samples
Training samples
(c)
(d)
1
80
IDCV−EVD
IDCV−GSO
70
0.98
60
50
0.96
40
30
0.94
DCV
IDCV−EVD
20
10
0.92
0
22
26
30
34
38
42
46
50
54
58
62
22
26
30
34
38
42
46
50
54
58
62
Training samples
Training samples
(e)
(f)
Fig. 2.
Results obtained with the 3 databases, CMU-PIE, JAFFE and Coil-20, one
at each row. (a),(c),(e) Averaged accuracy vs accumulated training set size for DCV
and IDCV-EVD. Results with IDCV-GSO and DCV are identical. (b),(d),(f) Relative
CPU time of both IDCV methods with regard to corresponding DCV ones.
At each iteration,
N
new images per class are available. The IDCV algorithms
are then run using the previous
M
images. The basic DCV algorithm is also run
from scratch using the current
M
+
N
images. In this way,
M
values range
approximately from 30 to 80% while the value of
N
has been fixed for each
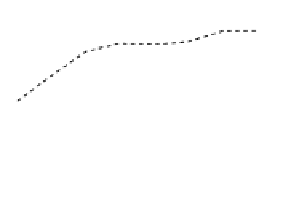
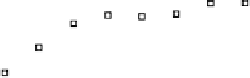







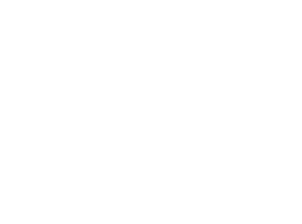
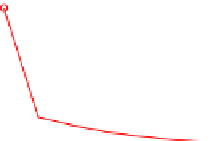






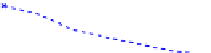












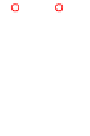







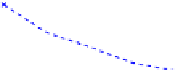



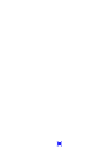

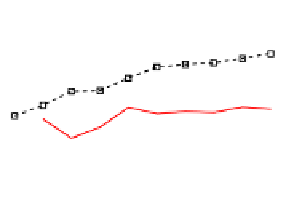





























































































































































































































































































































































































































































































































































































































































Search WWH ::

Custom Search