Information Technology Reference
In-Depth Information
Fig. 1.
Row 1: Screenshots from Internet streamed video sequences; Row 2: Copy-move
tamper emulation for the scene; Row 3: Detection of tampered regions in the scene
As can be seen in Table 1, the single mode noise residue features perform better
than quantization residue features. For both noise residue and quantization residue
features, the CCA, ICA and FLD features perform better than ARC features. CCA
features result in better accuracy for noise residue features as compared to others, as
they are based on canonical correlation analysis that can extract maximal correlation
properties better than features based on Fisher linear discriminant analysis. However,
for quantization residue features, the ICA features perform better than CCA features
showing that quantization information perturbed by tampering may not be necessarily
correlated, but could contain certain independent components. By fusing intra-frame
and inter-frame macro block features, we can see a better performance is achieved.
This shows that better correlation information can be extracted when multiple frames
are used for detecting tampers. Further, by fusing the two detectors, the detectors
based on noise residue features and quantization residue features, we can see that a
better performance is achieved as the two detectors complement each other, resulting
in a consistent and stable performance. This can be expected as quantization artefacts
for low-bandwidth video can significant damage tamper related correlation properties.
However, by using a hybrid fusion of quantization and noise residue features from
macro blocks, and using different feature selection techniques, we can see that a better
performance is achieved.
As we are using a pattern recognition formulation, the classifier used for making
decision on tampering is also equally important (in addition to an appropriate feature
selection technique). Hence the next experiment involved examining the performance
of GMM classifier with the neural network (NN) classifier based on back propagation
network proposed in [10], and the support vector machine (SVM) classifier based on
RBF kernel proposed in [17]. The results from this experiment are shown in Table 2
and Table 3. Since the experiments reported in Table 1 resulted in CCA and ICA
features as the best performing features, we used CCA and ICA features for
experimental results shown in Table 2 and Table 3.
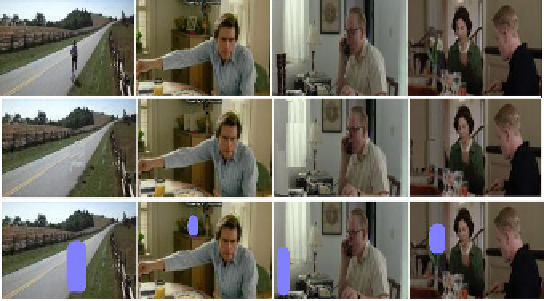
Search WWH ::

Custom Search