Biology Reference
In-Depth Information
from the individual representation of amino acids using physico-
chemical properties (ANN2) outperformed an ANN derived from the
clustering of amino acids (ANN1). Also, Nielsen
et al
. (2003) reported
that an ANN trained with a novel sequence encoding scheme using
Blosum50 and hidden Markov model sequence encoding for predic-
tion of T-cell class I epitopes was superior to one trained on 20-bin
sequence encoding (accuracy of 91.2% and 87.7%, respectively).
Another strategy to enhance prediction performance is to combine
several ANNs derived from different sequence-encoding schemes
instead of using one ANN system derived from a single sequence-
encoding scheme.
202,215
Although in some cases using either 20-bin
representation or physicochemical features may not affect classifica-
tion performance, the latter encoding strategy has important advan-
tages over sparse encoding: 1) it allows researchers to weigh in the
contribution of specific amino acid parameters and directly extract
their correlation to MHC peptide-binding properties and CTL epi-
tope SAR; 2) continuous-property scales for signal encoding, unlike
sparse encoding, reduces the dimension of the vector input and the
danger of generating arbitrary correlations that may lead the ANN to
overfit (this is especially important when data are sparse); and 3) in
contrast to sparse representation, physicochemical or encoding
schemes, like Blosum50, provide the neural network with less precise
and more general information about the sequence, which allows the
system to generalize better, mainly because it encodes subtle chemi-
cal and evolutionary relationships between the 20 amino acids.
202
Relevance of T-cell epitope prediction by ANN methods
. The
experiments described above provide compelling evidence for two
possible applications of ANN technology for immunological therapies
involving cytotoxic T-cell and T-cell mediated immunoresponses: vac-
cine and drug design. These applications differ, however, in their
requirements for specificity and sensitivity. For vaccine design, it is
more important to predict all possible binders to a given MHC mol-
ecule (specificity), whereas for drug design it is desirable to predict
those peptides that will bind with highest affinity (sensitivity). It
should be possible to manipulate the performance of the ANN to
optimize specificity or sensitivity as required for a given application.
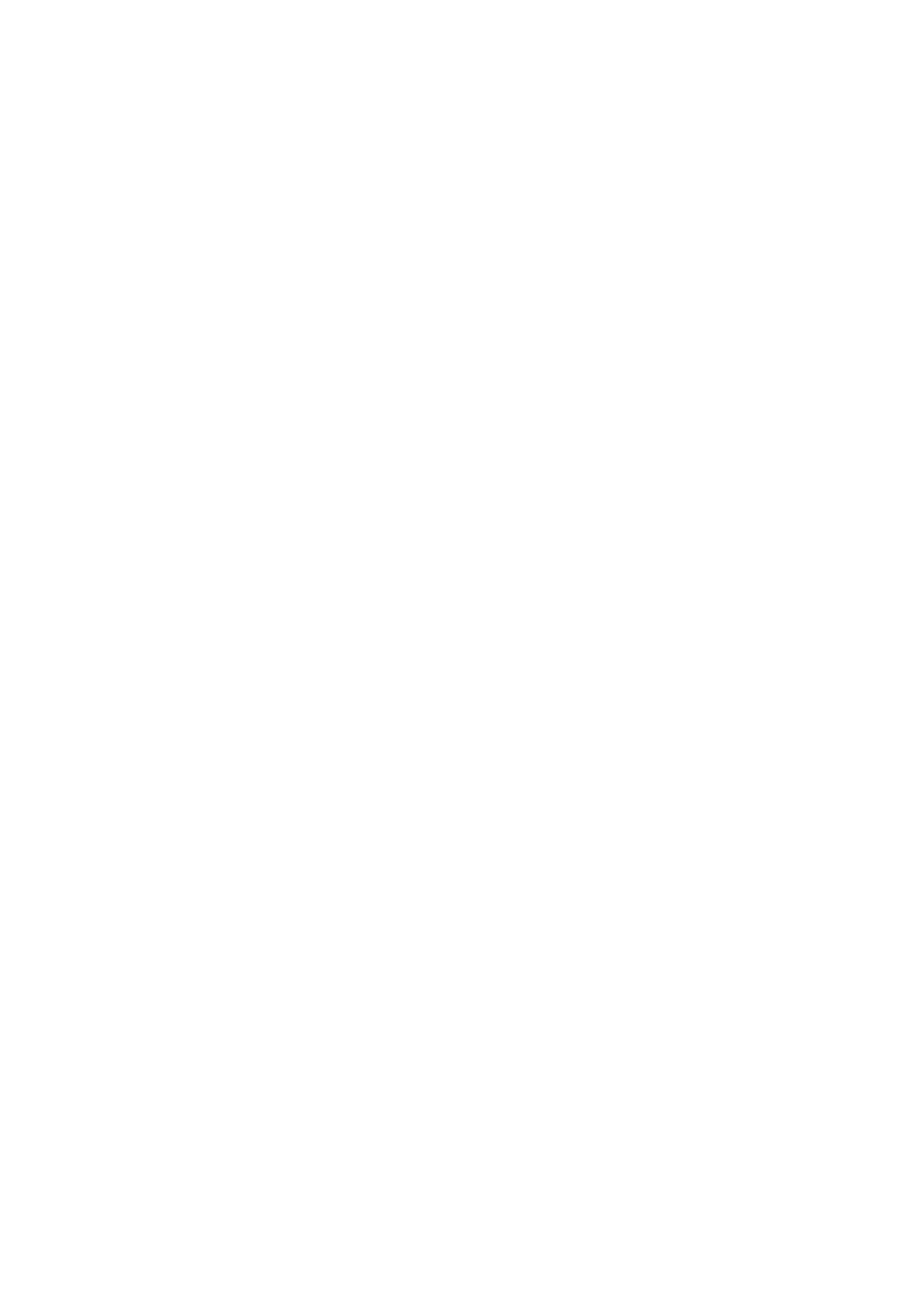