Geography Reference
In-Depth Information
Snow cover patterns
In cold regions snow is an important part of the water
balance, so getting snow deposition and snowmelt
parameters right is essential for predicting runoff in
ungauged basins. In gauged basins, model parameters
related to snow processes are usually (partly) estimated
from runoff data and often they can be improved by using
snow information within the basin (e.g.,
Parajka and
Blöschl, 2006
,
2008b
). In ungauged basins, information
on snow within the basin can improve the parameter
estimates above a-priori estimates and estimates
from neighbouring catchments in a similar way. The basic
steps of the approach are: obtain snow data (either
ground-based or, more often,
satellite data)
in the
catchment of interest; implement a rainfall
runoff model
at the ungauged location and estimate, if possible, the
parameters from catchment characteristics or regional
information; use the snow information to condition or
constrain the model parameters obtained from the other
sources of information. Obviously, the snow information
is particularly relevant to model parameters related to
snow processes, so these are the ones that can be
improved by the snow data. The snow data may also help
to update the simulated snow states themselves, which
may improve the runoff predictions.
Snow information that has received much attention
recently is snow cover images from the Moderate Reso-
lution Imaging Spectroradiometer (MODIS), which meas-
ures visible and infrared radiation. MODIS provides
twice-daily coverage at a spatial resolution of about 500
m pixel size. Cloud obscuration has been found to be the
major obstacle to applying MODIS data, but there are
numerous techniques that can effectively reduce cloud
cover, either by combination with other satellite data
and geo-data such as elevation or by spatio-temporal
filters (Parajka et al.,
2008a
,
2010b
). Numerous studies
have assimilated snow cover data into rainfall
-
Figure 10.31. Comparison of MODIS snow cover data (top panel)
with snow simulations with (bottom panel) and without (centre
panel) using MODIS for constraining model parameters. The region
shown is part of the Eastern Alps on 2 May 2001. From
Parajka and
Blöschl (2012).
runoff
models and found that the parameter estimates, the snow
simulations and/or the runoff predictions were indeed
significantly improved (see Parajka and Blöschl,
2012
for a review). Rodell and Houser (
2004
) and Andreadis
and Lettenmaier (
2006
) assimilated MODIS snow cover
into a hydrological model and found more accurate snow
cover simulations.
Udnaes et al. (2007)
and
-
shows a comparison of MODIS snow patterns with simu-
lations. In one variant (centre panel) the model was cali-
brated to runoff alone, while in the other variant (lower
panel), MODIS snow cover was used to constrain the
model parameters. Particularly in the eastern part of the
region, the improvements of the snow simulations are
apparent when MODIS data are used for constraining
the model parameters. Note that each pixel in the image
is ungauged. Clark et al.(
2006
) assimilated MODIS snow
covered area (SCA) into a hydrological model by an
ensemble Kalman filter. They suggested that the effi-
ciency in improving runoff predictions depends on the
speed of the transition from full snow cover to no snow
coverage.
orman et al.
(2009
) examined the potential of MODIS data for esti-
mating the parameters of a conceptual hydrological
model. They found improved snow model performance
and small (but distinct) improvements of the runoff model
efficiency. Parajka and Blöschl (
2008b
) showed that, in a
verification mode, the median NSE of runoff over 148
catchments increased from 0.67 to 0.70 if MODIS data
were used for calibration as compared to the case where
no MODIS data were used. As an example,
Figure 10.31
S¸
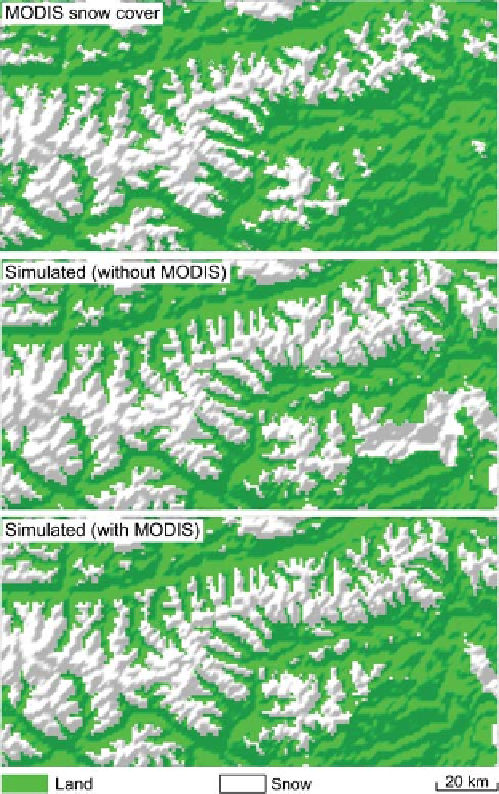
Search WWH ::

Custom Search