Geology Reference
In-Depth Information
processing, each designed to remove some specific ad-
verse effect of filtering in the ground along the transmis-
sion path, such as absorption or multiple reflection.
Deconvolution is the analytical process of removing
the effect of some previous filtering operation (convolu-
tion). Inverse filters are designed to deconvolve seismic
traces by removing the adverse filtering effects associated
with the propagation of seismic pulses through a layered
ground or through a recording system. In general, such
effects lengthen the seismic pulse; for example, by the
generation of multiple wave trains and by progressive ab-
sorption of the higher frequencies. Mutual interference
of extended reflection wave trains from individual inter-
faces seriously degrades seismic records since onsets of
reflections from deeper interfaces are totally or partially
concealed by the wave trains of reflections from shal-
lower interfaces.
Examples of inverse filtering to remove particular
filtering effects include:
•
dereverberation
to remove ringing associated with
multiple reflections in a water layer;
•
deghosting
to remove the short-path multiple asso-
ciated with energy travelling upwards from the source
and reflected back from the base of the weathered layer
or the surface; and
•
whitening
to equalize the amplitude of all frequency
components within the recorded frequency band (see
below).
All these deconvolution operations have the effect of
shortening the pulse length on processed seismic sec-
tions and, thus, improve the vertical resolution.
Consider a composite waveform
w
k
resulting from an
initial spike source extended by the presence of short-
path multiples near source such as, especially, water layer
reverberations. The resultant seismic trace
x
k
will be
given by the convolution of the reflectivity function
r
k
with the composite input waveform
w
k
as shown
schematically in Fig. 4.6 (neglecting the effects of
attenuation and absorption)
therefore not suppressed by CDP stacking, and they have
similar frequency content to the primary reflection so
that they cannot be removed by frequency filtering.
Deconvolution has the general aim, not fully realiz-
able, of compressing every occurrence of a composite
waveform
w
k
on a seismic trace into a spike output, in
order to reproduce the reflectivity function
r
k
that would
fully define the subsurface layering.This is equivalent to
the elimination of the multiple wave train.The required
deconvolution operator is an inverse filter
i
k
which,
when convolved with the composite waveform
w
k
,
yields a spike function
d
k
i
w d
=
*
k
k
k
Convolution of the same operator with the entire
seismic trace yields the reflectivity function
i
x r
=
*
k
k
k
Where
w
k
is known, deconvolution can be achieved
by the use of
matched filters
which effectively cross-
correlate the output with the known input signal (as in
the initial processing of
Vibroseis
®
seismic records to
compress the long source wave train; see Section 3.8.1).
Wiener filters
may also be used when the input signal is
known. A Wiener filter (Fig. 4.20) converts the known
input signal into an output signal that comes closest, in a
least-squares sense, to a desired output signal. The filter
optimizes the output signal by arranging that the sum of
squares of differences between the actual output and the
desired output is a minimum.
Although special attempts are sometimes made in
marine surveys to measure the source signature directly,
by suspending hydrophones in the vicinity of the source,
both
w
k
and
r
k
are generally unknown in reflection sur-
veying.The reflectivity function
r
k
is, of course, the main
target of reflection surveying. Since, normally, only the
seismic time series
x
k
is known, a special approach is
required to design suitable inverse filters. This approach
uses statistical analysis of the seismic time series, as in
predictive deconvolution
which attempts to remove the
effect of multiples by predicting their arrival times from
knowledge of the arrival times of the relevant primary
events. Two important assumptions underlying predic-
tive deconvolution (see e.g. Robinson & Treitel 2000)
are:
1.
that the reflectivity function represents a random
series (i.e. that there is no systematic pattern to the
distribution of reflecting interfaces in the ground); and
xr
=
w
(
plus noise
)
*
k
k
k
Reflected waveforms from closely-spaced reflectors will
overlap in time on the seismic trace and, hence, will in-
terfere. Deeper reflections may thus be concealed by the
reverberation wave train associated with reflections from
shallower interfaces, so that only by the elimination of
the multiples will all the primary reflections be revealed.
Note that short-path multiples have effectively the same
normal moveout as the related primary reflection and are


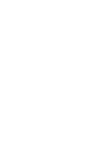