Graphics Reference
In-Depth Information
Fig. 5.5
Using C4.5 to build a rule set to predict noise filtering efficacy
among classes, their separability or the linearity of the decision boundaries. The
most commonly used data complexity set of measures are those gathered together
by Ho and Basu [
35
]. They consist of 12 metrics designed for binary classification
problems that numerically estimate the difficulty of 12 different aspects of the data.
For some measures lower/higher values mean a more difficult problem regarding to
such a characteristic. Having a numeric description of the difficult aspects of the data
opens a new question: can we predict which characteristics are related with noise
and will they be successfully corrected by noise filters?
This prediction can help, for example, to determine an appropriate noise filter
for a concrete noisy data set such a filter providing a signicant advantage in terms
of the results or to design new noise filters which select more or less aggressive
filtering strategies considering the characteristics of the data. Choosing a noise-
sensitive learner facilitates the checking of when a filter removes the appropriate
noisy examples in contrast to a robust learner-the performance of classiers built by
the former is more sensitive to noisy examples retained in the data set after the ltering
process.
A way to formulate rules that describe when it is appropriate to filter the data
follows the scheme depicted in Fig.
5.5
. From an initial set of 34 data sets from
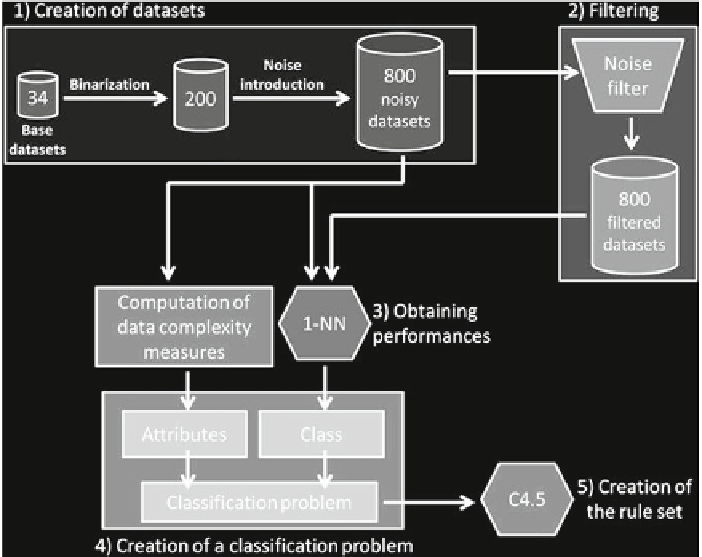